Generalising Random Forest Parameter Optimisation to Include Stability and Cost
Lecture Notes in Artificial Intelligence(2017)
摘要
Random forests are among the most popular classification and regression methods used in industrial applications. To be effective, the parameters of random forests must be carefully tuned. This is usually done by choosing values that minimize the prediction error on a held out dataset. We argue that error reduction is only one of several metrics that must be considered when optimizing random forest parameters for commercial applications. We propose a novel metric that captures the stability of random forests predictions, which we argue is key for scenarios that require successive predictions. We motivate the need for multi-criteria optimization by showing that in practical applications, simply choosing the parameters that lead to the lowest error can introduce unnecessary costs and produce predictions that are not stable across independent runs. To optimize this multi-criteria trade-off, we present a new framework that efficiently finds a principled balance between these three considerations using Bayesian optimisation. The pitfalls of optimising forest parameters purely for error reduction are demonstrated using two publicly available real world datasets. We show that our framework leads to parameter settings that are markedly different from the values discovered by error reduction metrics.
更多查看译文
关键词
Bayesian optimisation,Parameter tuning,Random forest,Machine learning application,Model stability
AI 理解论文
溯源树
样例
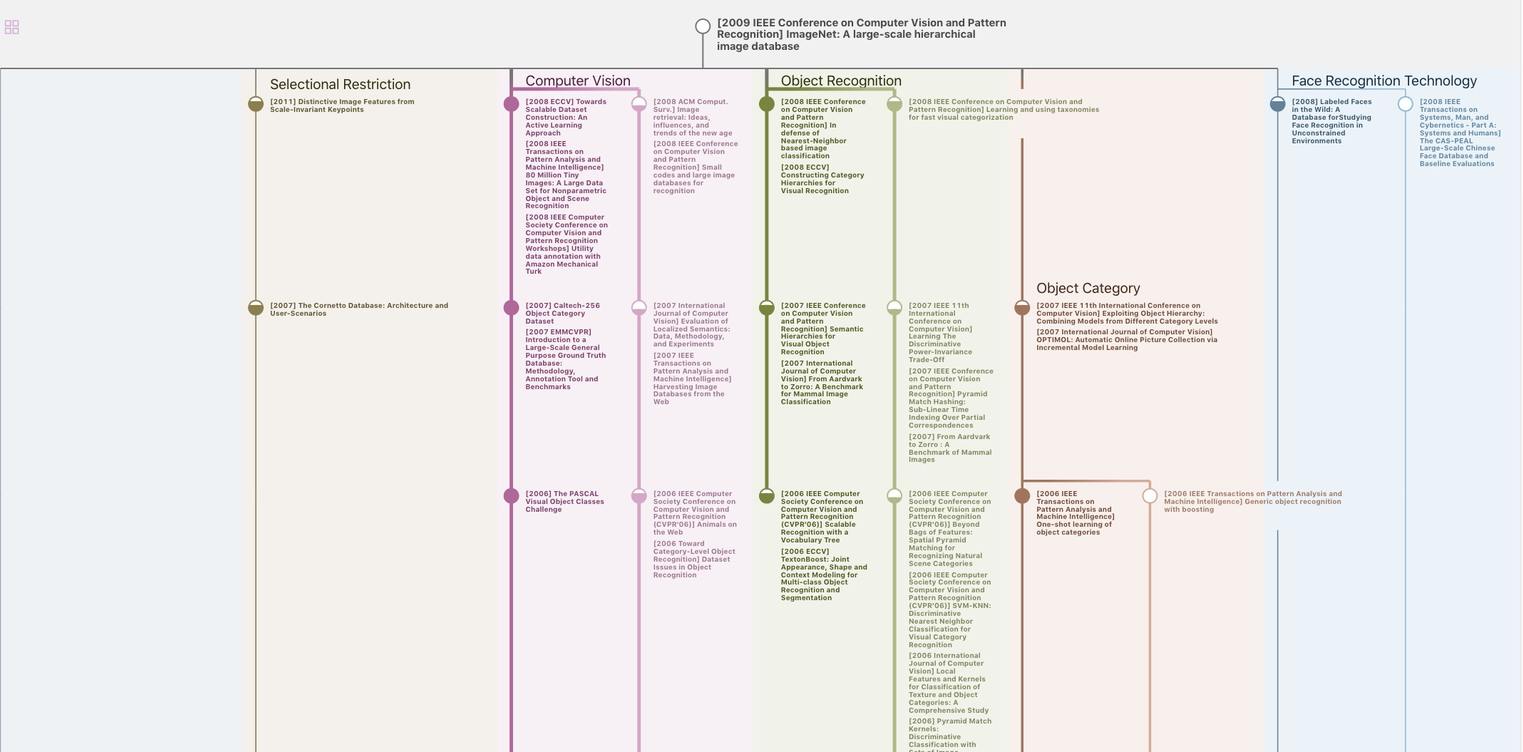
生成溯源树,研究论文发展脉络
Chat Paper
正在生成论文摘要