Answer set programming for non-stationary Markov decision processes
Applied Intelligence(2017)
摘要
Non-stationary domains, where unforeseen changes happen, present a challenge for agents to find an optimal policy for a sequential decision making problem. This work investigates a solution to this problem that combines Markov Decision Processes (MDP) and Reinforcement Learning (RL) with Answer Set Programming (ASP) in a method we call ASP(RL). In this method, Answer Set Programming is used to find the possible trajectories of an MDP, from where Reinforcement Learning is applied to learn the optimal policy of the problem. Results show that ASP(RL) is capable of efficiently finding the optimal solution of an MDP representing non-stationary domains.
更多查看译文
关键词
Non-determinism,Markov decision processes,Answer set programming,Action languages
AI 理解论文
溯源树
样例
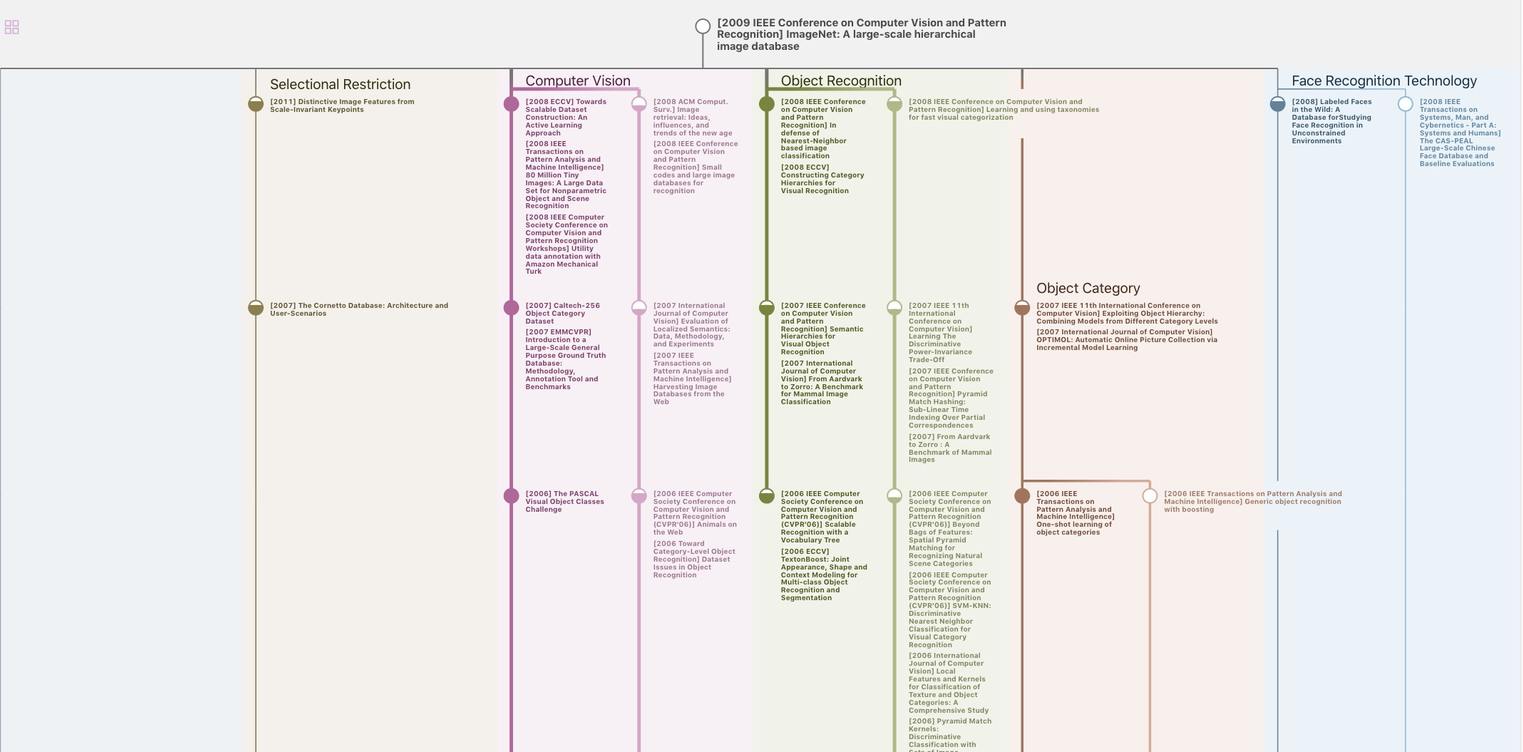
生成溯源树,研究论文发展脉络
Chat Paper
正在生成论文摘要