Pedestrian Prediction by Planning using Deep Neural Networks
2018 IEEE INTERNATIONAL CONFERENCE ON ROBOTICS AND AUTOMATION (ICRA)(2017)
摘要
Accurate traffic participant prediction is the prerequisite for collision avoidance of autonomous vehicles. In this work, we predict pedestrians by emulating their own motion planning. From online observations, we infer a mixture density function for possible destinations. We use this result as the goal states of a planning stage that performs motion prediction based on common behavior patterns. The entire system is modeled as one monolithic neural network and trained via inverse reinforcement learning. Experimental validation on real world data shows the system's ability to predict both, destinations and trajectories accurately.
更多查看译文
关键词
monolithic neural network,inverse reinforcement learning,pedestrian prediction,deep neural networks,collision avoidance,autonomous vehicles,goal-directed planning,mixture density function,motion prediction,convolutional network,traffic participant prediction,trajectories
AI 理解论文
溯源树
样例
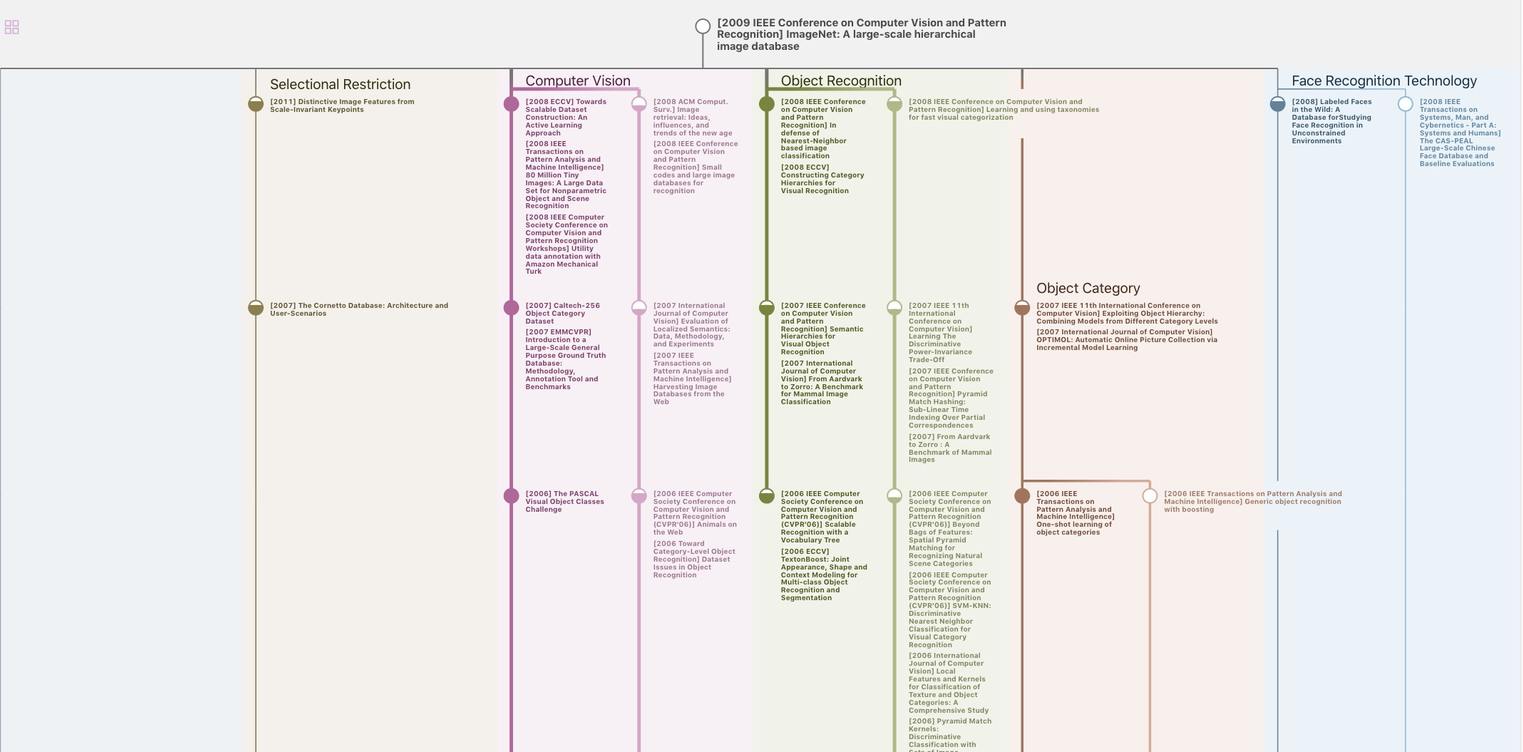
生成溯源树,研究论文发展脉络
Chat Paper
正在生成论文摘要