Calibration of a two-state pitch-wise HMM method for note segmentation in Automatic Music Transcription systems.
CoRR(2017)
摘要
Many methods for automatic music transcription involves a multi-pitch estimation method that estimates an activity score for each pitch. A second processing step, called note segmentation, has to be performed for each pitch in order to identify the time intervals when the notes are played. In this study, a pitch-wise two-state on/off firstorder Hidden Markov Model (HMM) is developed for note segmentation. A complete parametrization of the HMM sigmoid function is proposed, based on its original regression formulation, including a parameter alpha of slope smoothing and beta? of thresholding contrast. A comparative evaluation of different note segmentation strategies was performed, differentiated according to whether they use a fixed threshold, called "Hard Thresholding" (HT), or a HMM-based thresholding method, called "Soft Thresholding" (ST). This evaluation was done following MIREX standards and using the MAPS dataset. Also, different transcription scenarios and recording natures were tested using three units of the Degradation toolbox. Results show that note segmentation through a HMM soft thresholding with a data-based optimization of the {alpha,beta} parameter couple significantly enhances transcription performance.
更多查看译文
AI 理解论文
溯源树
样例
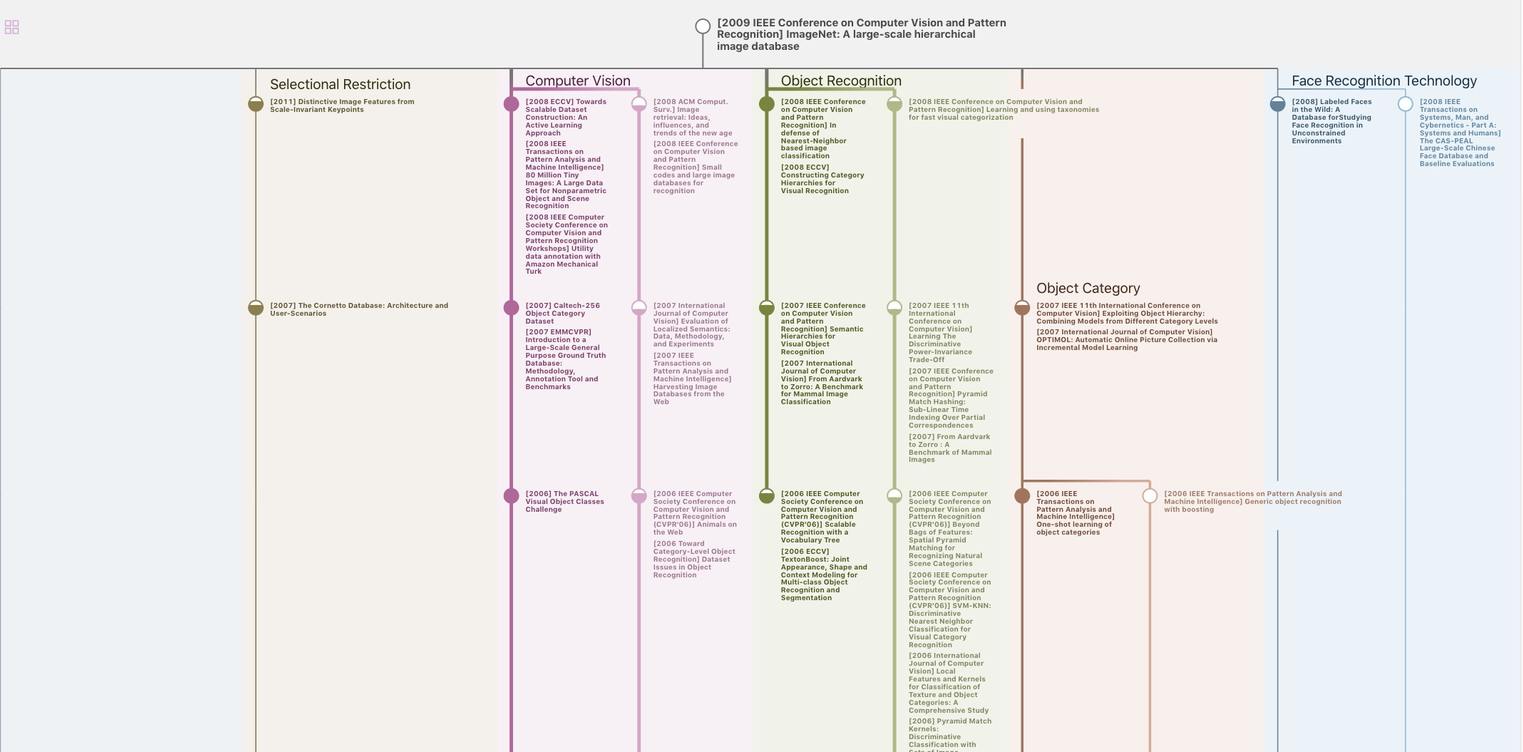
生成溯源树,研究论文发展脉络
Chat Paper
正在生成论文摘要