Learning from Multi-View Multi-Way Data via Structural Factorization Machines.
arXiv: Learning(2018)
摘要
Real-world relations among entities can often be observed and determined by different perspectives/views. For example, the decision made by a user on whether to adopt an item relies on multiple aspects such as the contextual information of the decision, the item»s attributes, the user»s profile and the reviews given by other users. Different views may exhibit multi-way interactions among entities and provide complementary information. In this paper, we introduce a multi-tensor-based approach that can preserve the underlying structure of multi-view data in a generic predictive model. Specifically, we propose structural factorization machines (SFMs) that learn the common latent spaces shared by multi-view tensors and automatically adjust the importance of each view in the predictive model. Furthermore, the complexity of SFMs is linear in the number of parameters, which make SFMs suitable to large-scale problems. Extensive experiments on real-world datasets demonstrate that the proposed SFMs outperform several state-of-the-art methods in terms of prediction accuracy and computational cost.
更多查看译文
关键词
Tensor Factorization, Multi-Way Interaction, Multi-View Learning
AI 理解论文
溯源树
样例
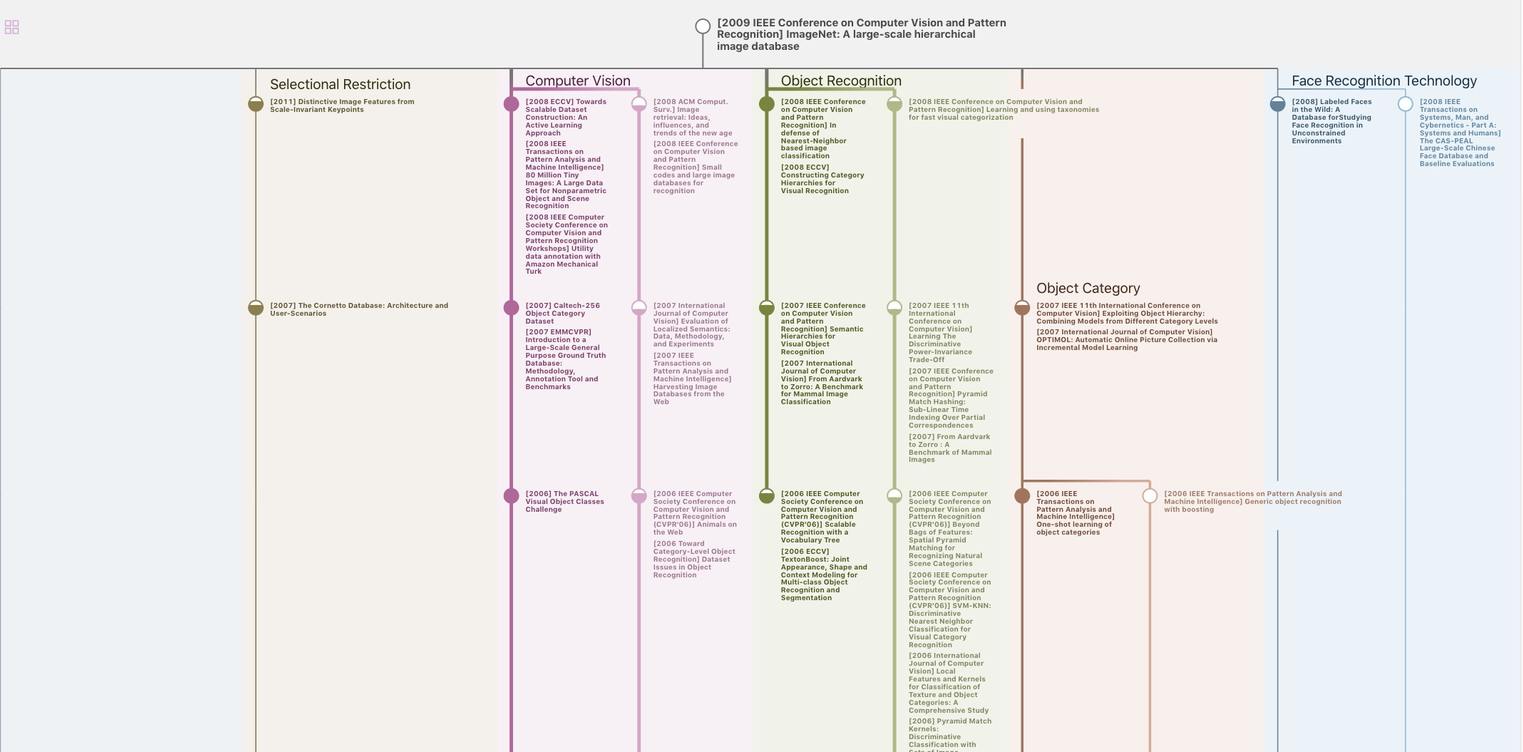
生成溯源树,研究论文发展脉络
Chat Paper
正在生成论文摘要