Joint Sequence Learning and Cross-Modality Convolution for 3D Biomedical Segmentation
2017 IEEE Conference on Computer Vision and Pattern Recognition (CVPR)(2017)
摘要
Deep learning models such as convolutional neural net- work have been widely used in 3D biomedical segmentation and achieve state-of-the-art performance. However, most of them often adapt a single modality or stack multiple modalities as different input channels. To better leverage the multi- modalities, we propose a deep encoder-decoder structure with cross-modality convolution layers to incorporate different modalities of MRI data. In addition, we exploit convolutional LSTM to model a sequence of 2D slices, and jointly learn the multi-modalities and convolutional LSTM in an end-to-end manner. To avoid converging to the certain labels, we adopt a re-weighting scheme and two-phase training to handle the label imbalance. Experimental results on BRATS-2015 show that our method outperforms state-of-the-art biomedical segmentation approaches.
更多查看译文
关键词
joint sequence learning,cross-modality convolution,3D biomedical segmentation,deep learning models,convolutional neural network,single modality,deep convolution encoder-decoder structure,convolutional LSTM,stack multiple modalities,MRI data,convLSTM,reweighting scheme,label imbalance handling,2D slices sequence
AI 理解论文
溯源树
样例
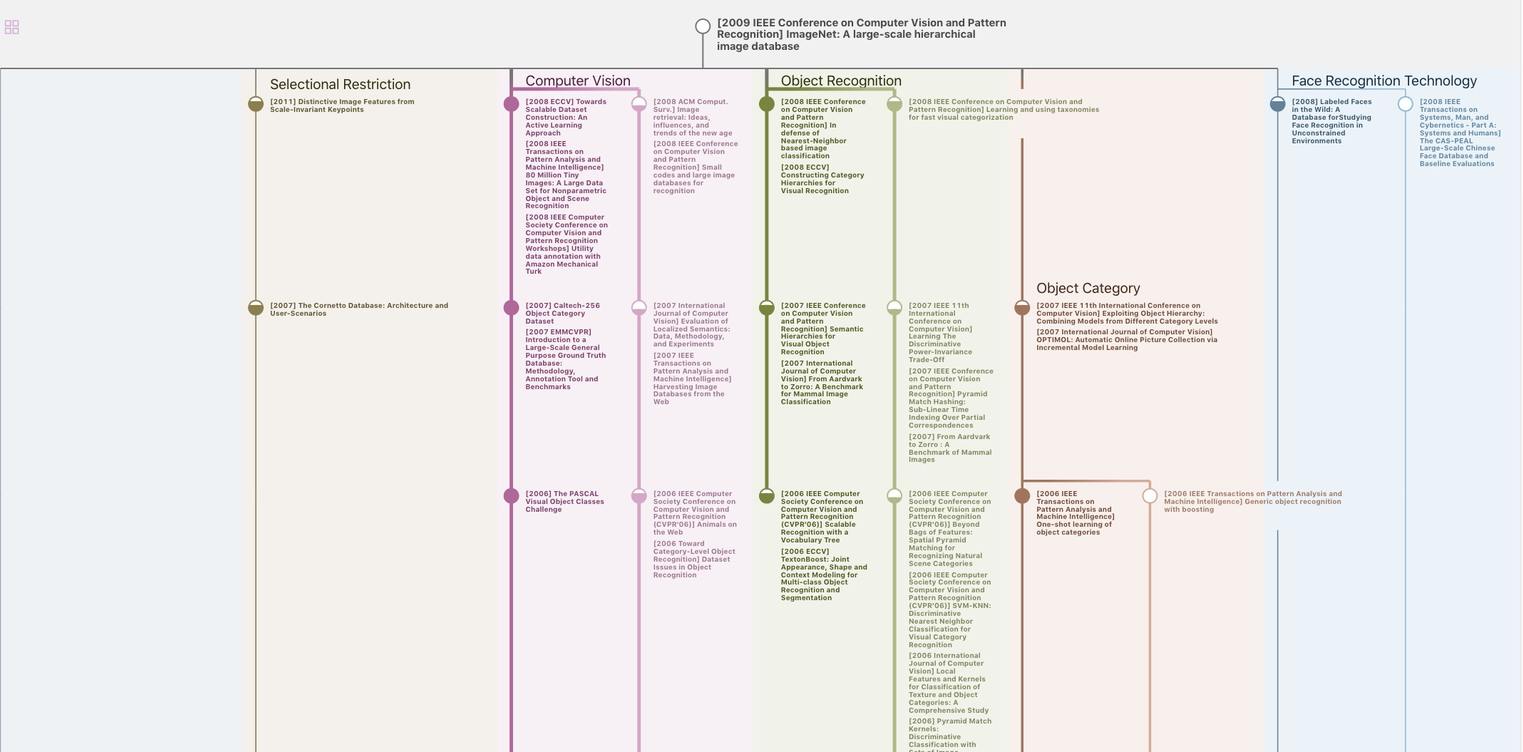
生成溯源树,研究论文发展脉络
Chat Paper
正在生成论文摘要