Two-temperature logistic regression based on the Tsallis divergence
22ND INTERNATIONAL CONFERENCE ON ARTIFICIAL INTELLIGENCE AND STATISTICS, VOL 89(2017)
摘要
We develop a variant of multiclass logistic regression that is significantly more robust to noise. The algorithm has one weight vector per class and the surrogate loss is a function of the linear activations (one per class). The surrogate loss of an example with linear activation vector 𝐚 and class c has the form -log_t_1exp_t_2 (a_c - G_t_2(𝐚)) where the two temperatures t_1 and t_2 ”temper” the log and exp, respectively, and G_t_2(𝐚) is a scalar value that generalizes the log-partition function. We motivate this loss using the Tsallis divergence. Our method allows transitioning between non-convex and convex losses by the choice of the temperature parameters. As the temperature t_1 of the logarithm becomes smaller than the temperature t_2 of the exponential, the surrogate loss becomes ”quasi convex”. Various tunings of the temperatures recover previous methods and tuning the degree of non-convexity is crucial in the experiments. In particular, quasi-convexity and boundedness of the loss provide significant robustness to the outliers. We explain this by showing that t_1 < 1 caps the surrogate loss and t_2 >1 makes the predictive distribution have a heavy tail. We show that the surrogate loss is Bayes-consistent, even in the non-convex case. Additionally, we provide efficient iterative algorithms for calculating the log-partition value only in a few number of iterations. Our compelling experimental results on large real-world datasets show the advantage of using the two-temperature variant in the noisy as well as the noise free case.
更多查看译文
AI 理解论文
溯源树
样例
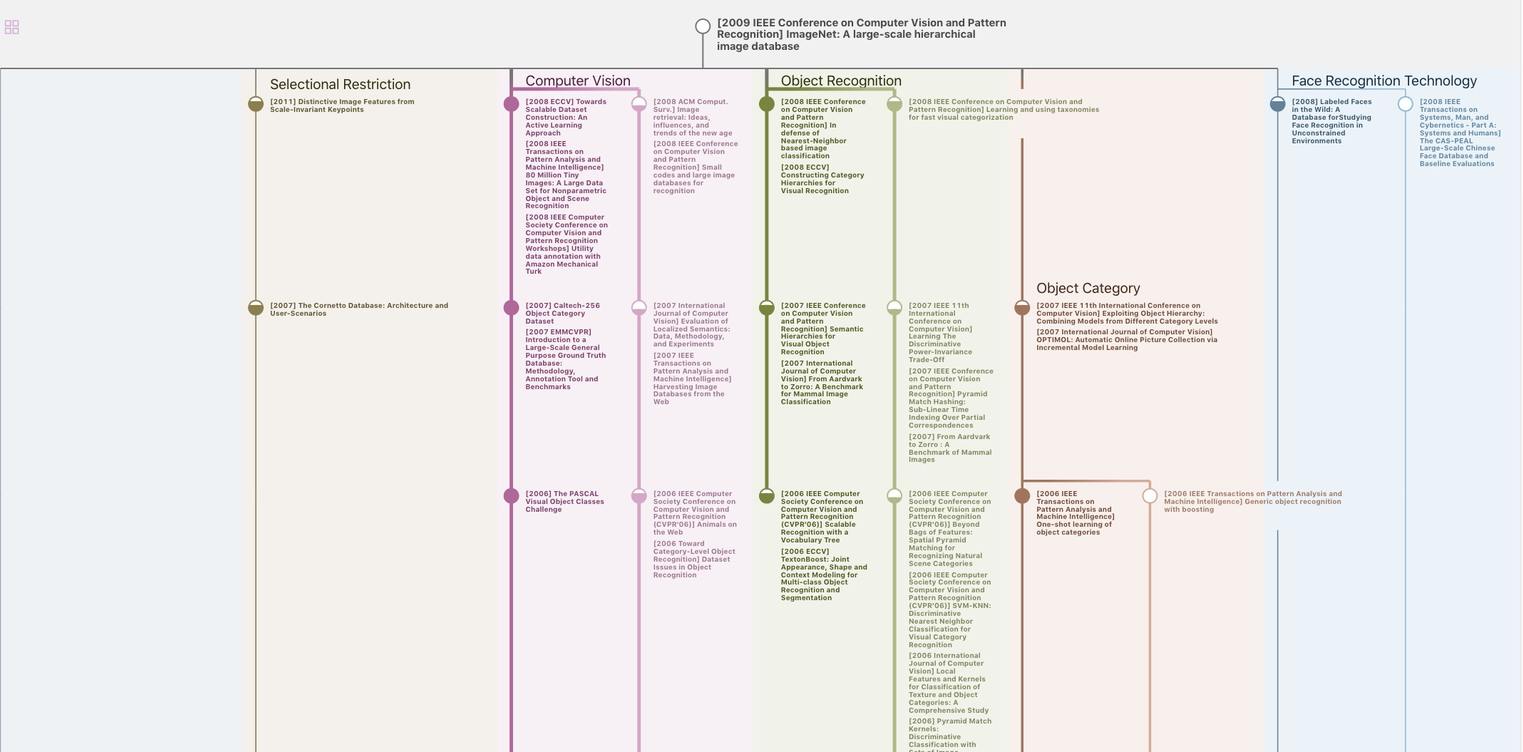
生成溯源树,研究论文发展脉络
Chat Paper
正在生成论文摘要