Partial Hard Thresholding.
IEEE Trans. Information Theory(2017)
摘要
We study iterative algorithms for compressed sensing that have an “orthogonalization” step at each iteration to keep the residual orthogonal to the span of those columns of the measurement matrix that have been selected so far. To unify the design and analysis of such algorithms, we propose a novel partial hard-thresholding (PHT) operator that is similar to the hard thresholding operator but restricts the amount by which the support set can change in one iteration. Using the PHT operator and its properties, we provide a general framework to prove support recovery results for iterative algorithms employing this operator as well as those employing the hard-thresholding operator. Next, based on the PHT operator, we propose a novel family of algorithms. At one end of our family of algorithms lie well-known hard thresholding algorithms iterative thresholding with inversion and hard thresholding pursuit, whereas at the other end, we get a novel algorithm that we call orthogonal matching pursuit with replacement (OMPR). Like the classic greedy algorithm OMP, OMPR too adds exactly one coordinate to the support of the iterate at each iteration based on the correlation with the current residual. However, unlike OMP, OMPR also removes one coordinate from the support. This simple change allows us to prove that OMPR has the best known guarantees for sparse recovery in terms of the restricted isometry property (RIP), a condition on the measurement matrix. In contrast, OMP is known to have very weak performance guarantees under RIP. Finally, we show that most of the existing “orthogonalized” iterative algorithms, such as CoSaMP, subspace pursuit, OMP, can be expressed using the PHT operator. As a pleasing consequence of our novel and generic results for the PHT operator, we provide the tightest known RIP analysis of all of the above-mentioned iterative algorithms: CoSaMP, subspace pursuit, and OMP.
更多查看译文
关键词
Matching pursuit algorithms,Iterative algorithms,Sparse matrices,Classification algorithms,Standards,Minimization
AI 理解论文
溯源树
样例
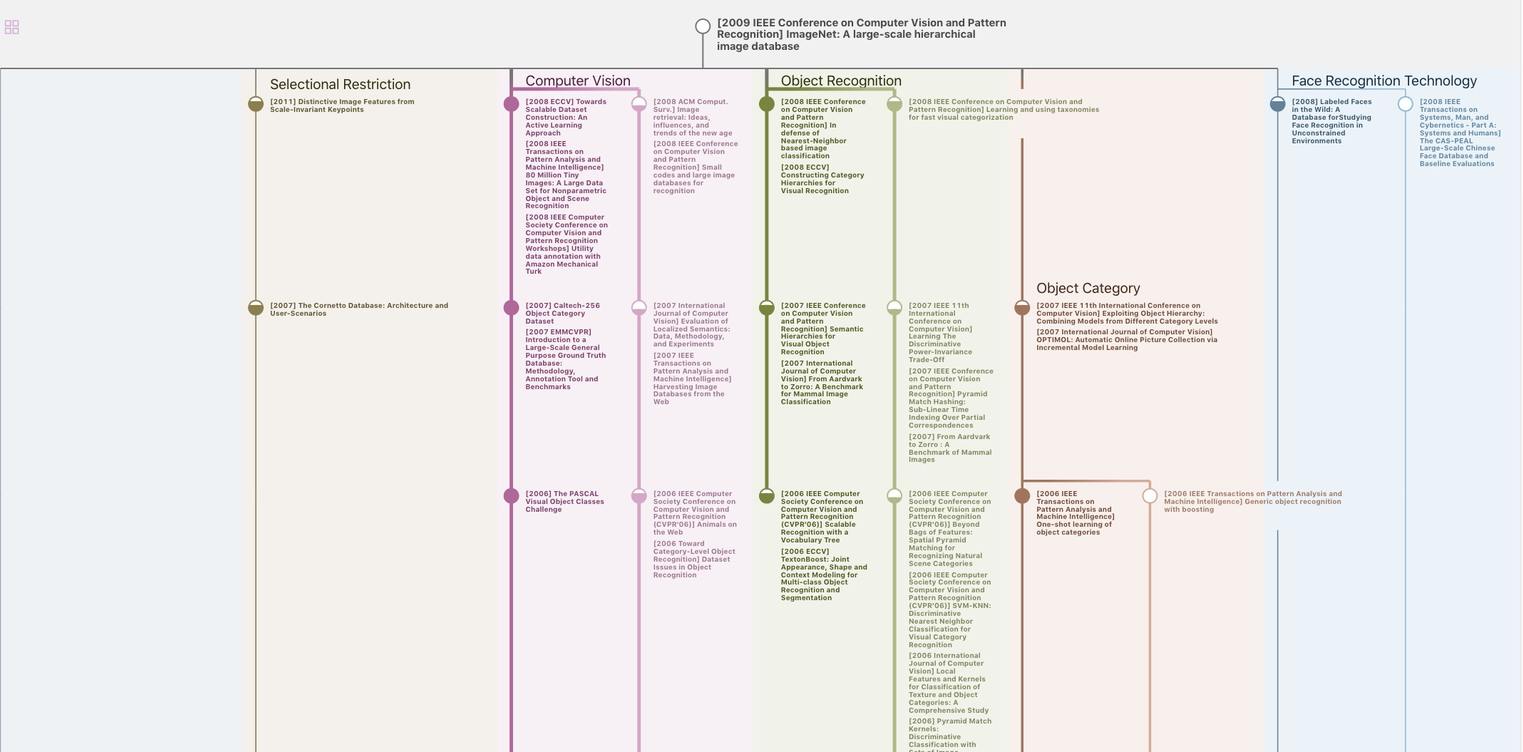
生成溯源树,研究论文发展脉络
Chat Paper
正在生成论文摘要