Improved validation framework and R-package for artificial neural network models.
Environmental Modelling and Software(2017)
摘要
Validation is a critical component of any modelling process. In artificial neural network (ANN) modelling, validation generally consists of the assessment of model predictive performance on an independent validation set (predictive validity). However, this ignores other aspects of model validation considered to be good practice in other areas of environmental modelling, such as residual analysis (replicative validity) and checking the plausibility of the model in relation to a priori system understanding (structural validity). In order to address this shortcoming, a validation framework for ANNs is introduced in this paper that covers all of the above aspects of validation. In addition, the validann R-package is introduced that enables these validation methods to be implemented in a user-friendly and consistent fashion. The benefits of the framework and R-package are demonstrated for two environmental modelling case studies, highlighting the importance of considering replicative and structural validity in addition to predictive validity. A comprehensive validation framework for ANNs is proposed.The validann R-package for implementing the validation framework is introduced.Application of the framework and R-package is demonstrated on two real case studies.Results reveal that predictively valid ANN models may not be credible.Adoption of the framework leads to improvements in overall ANN validity.
更多查看译文
关键词
Artificial neural networks,Multi-layer perceptron,R-package,Structural validation,Replicative validation,Predictive validation
AI 理解论文
溯源树
样例
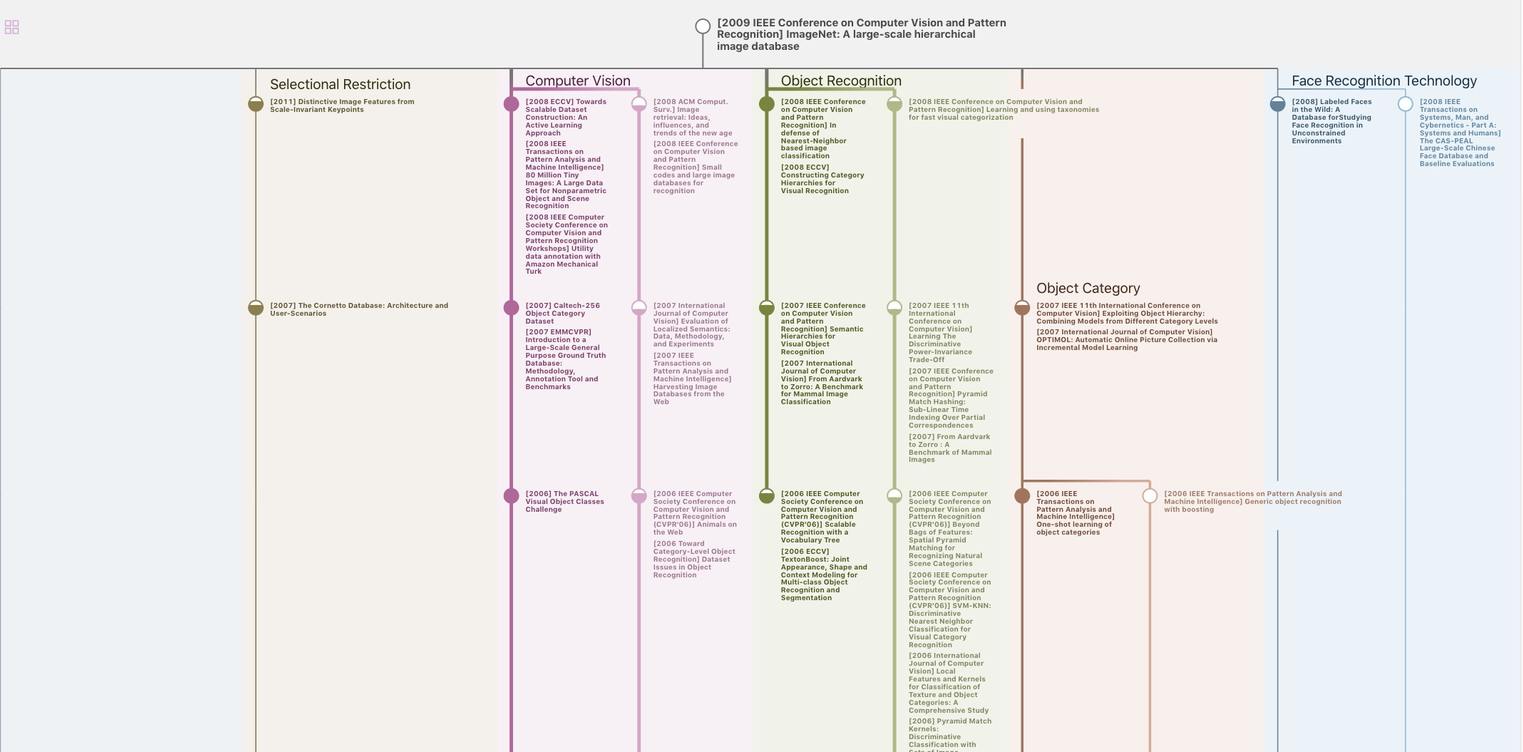
生成溯源树,研究论文发展脉络
Chat Paper
正在生成论文摘要