Hypergraph Embedding for Spatial-Spectral Joint Feature Extraction in Hyperspectral Images.
REMOTE SENSING(2017)
摘要
The fusion of spatial and spectral information in hyperspectral images (HSIs) is useful for improving the classification accuracy. However, this approach usually results in features of higher dimension and the curse of the dimensionality problem may arise resulting from the small ratio between the number of training samples and the dimensionality of features. To ease this problem, we propose a novel algorithm for spatial-spectral feature extraction based on hypergraph embedding. Firstly, each HSI pixel is regarded as a vertex and the joint of extended morphological profiles (EMP) and spectral features is adopted as the feature associated with the vertex. A hypergraph is then constructed by the K-Nearest-Neighbor method, in which each pixel and its most K relevant pixels are linked as one hyperedge to represent the complex relationships between HSI pixels. Secondly, the hypergraph embedding model is designed to learn a low dimensional feature with the reservation of geometric structure of HSI. An adaptive hyperedge weight estimation scheme is also introduced to preserve the prominent hyperedges by the regularization constraint on the weight. Finally, the learned low-dimensional features are fed to the support vector machine (SVM) for classification. The experimental results on three benchmark hyperspectral databases are presented. They highlight the importance of spatial-spectral joint features embedding for the accurate classification of HSI data. The weight estimation is better for further improving the classification accuracy. These experimental results verify the proposed method.
更多查看译文
关键词
feature extraction,hypergraph learning,morphological profiles,hyperedge weight estimation
AI 理解论文
溯源树
样例
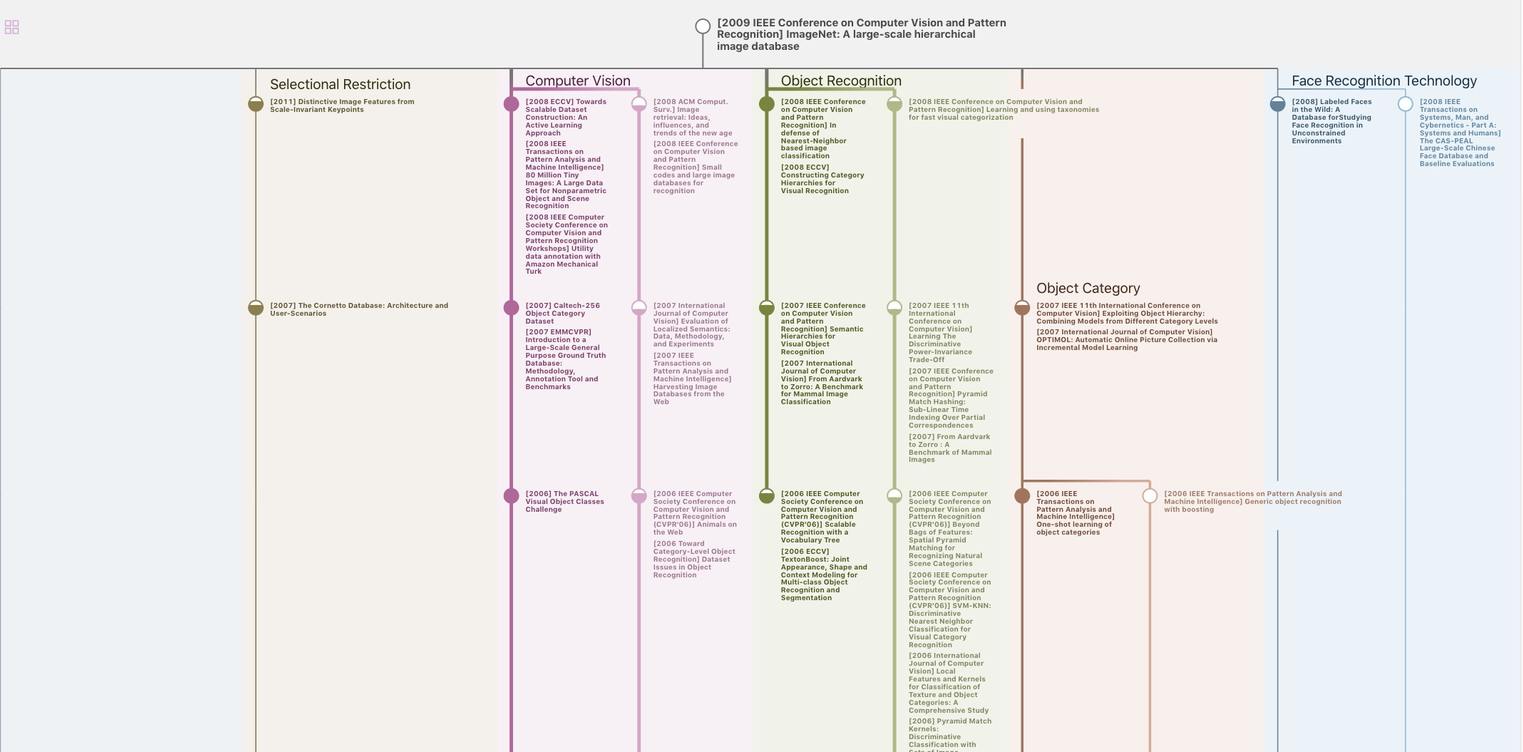
生成溯源树,研究论文发展脉络
Chat Paper
正在生成论文摘要