A Symmetric Sparse Representation Based Band Selection Method for Hyperspectral Imagery Classification.
REMOTE SENSING(2016)
摘要
A novel Symmetric Sparse Representation (SSR) method has been presented to solve the band selection problem in hyperspectral imagery (HSI) classification. The method assumes that the selected bands and the original HSI bands are sparsely represented by each other, i.e., symmetrically represented. The method formulates band selection into a famous problem of archetypal analysis and selects the representative bands by finding the archetypes in the minimal convex hull containing the HSI band points (i.e., one band corresponds to a band point in the high-dimensional feature space). Without any other parameter tuning work except the size of band subset, the SSR optimizes the band selection program using the block-coordinate descent scheme. Four state-of-the-art methods are utilized to make comparisons with the SSR on the Indian Pines and PaviaU HSI datasets. Experimental results illustrate that SSR outperforms all four methods in classification accuracies (i.e., Average Classification Accuracy (ACA) and Overall Classification Accuracy (OCA)) and three quantitative evaluation results (i.e., Average Information Entropy (AIE), Average Correlation Coefficient (ACC) and Average Relative Entropy (ARE)), whereas it takes the second shortest computational time. Therefore, the proposed SSR is a good alternative method for band selection of HSI classification in realistic applications.
更多查看译文
关键词
symmetric sparse representation,archetypal analysis,classification,hyperspectral imagery,band selection
AI 理解论文
溯源树
样例
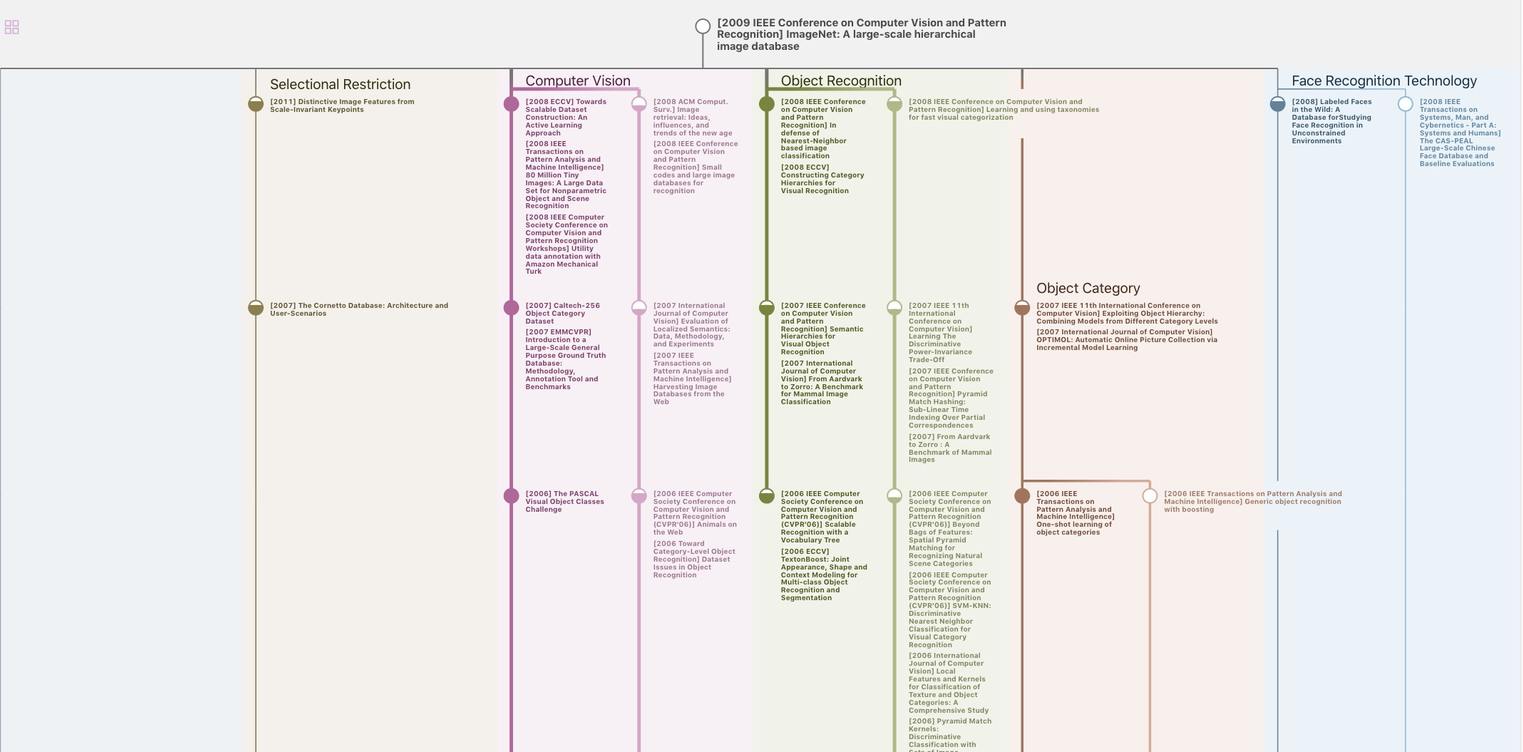
生成溯源树,研究论文发展脉络
Chat Paper
正在生成论文摘要