Ensemble Empirical Mode Decomposition and Sparsity Measurement as Tools Enhancing the Gear Diagnostic Capabilities of Time Synchronous Averaging.
ADVANCES IN DATA SCIENCE AND ADAPTIVE ANALYSIS(2017)
摘要
The paper introduces the concept of exploring the potential of Ensemble Empirical Mode Decomposition (EEMD) and Sparsity Measurement (SM) in enhancing the diagnostic information contained in the Time Synchronous Averaging (TSA) method used in the field of gearbox diagnostics. EEMD was created as a natural improvement of the Empirical Mode Decomposition which suffered from a so-called mode mixing problem. SM is heavily used in the field of ultrasound signal processing as a tool for assessing the degree of sparsity of a signal. A novel process of automatically finding the optimal parameters of EEMD is proposed by incorporating a Form Factor parameter, known from the field of electrical engineering. All these elements are combined and applied on a set of vibration data generated on a 2-stage gearbox under healthy and faulty conditions. The results suggest that combining these methods may increase the robustness of the condition monitoring routine, when compared to the standard TSA used alone.
更多查看译文
关键词
Ensemble empirical mode decomposition,sparsity measurement,form factor,Hilbert-Huang spectrum,gearbox,diagnostics
AI 理解论文
溯源树
样例
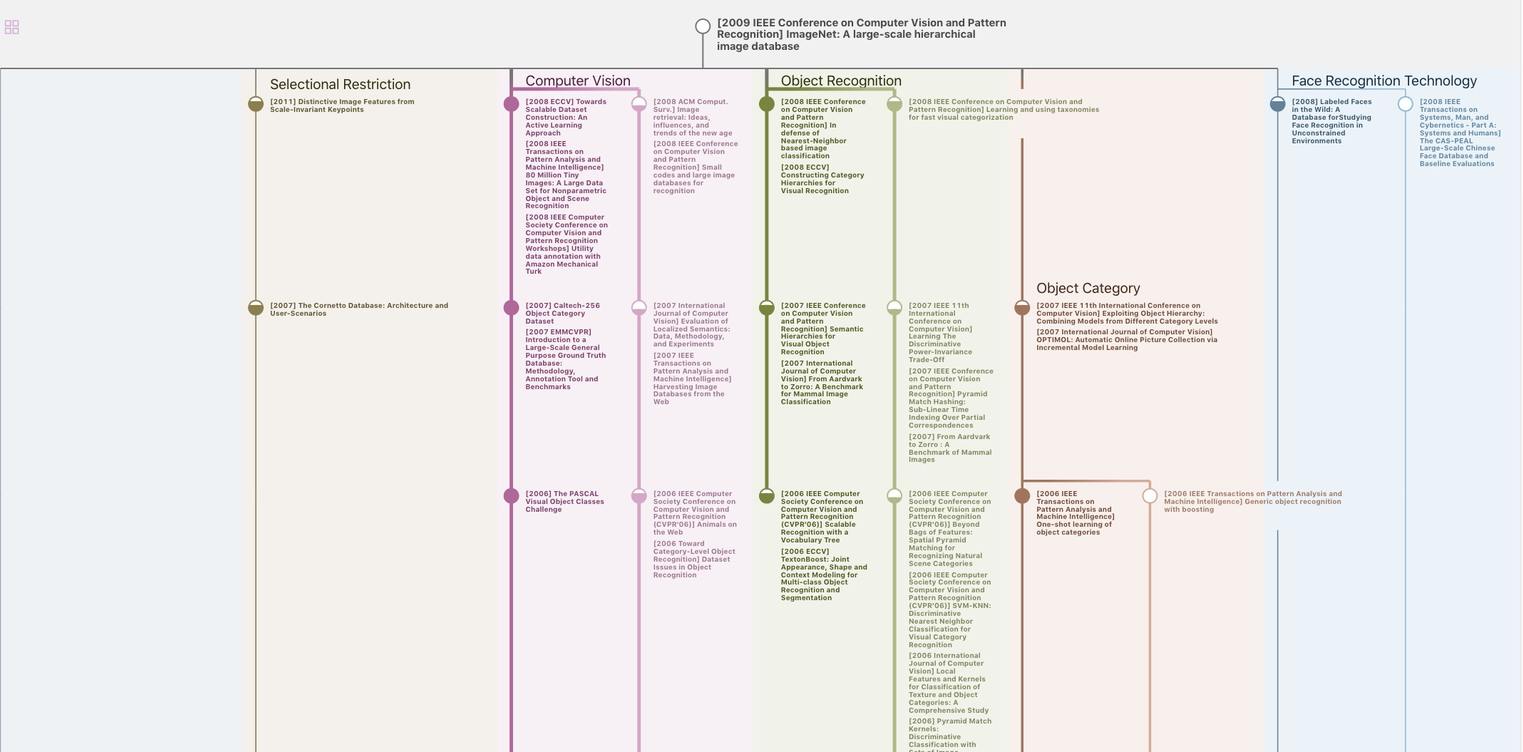
生成溯源树,研究论文发展脉络
Chat Paper
正在生成论文摘要