Measuring Complexity and Predictability of Time Series with Flexible Multiscale Entropy for Sensor Networks.
SENSORS(2017)
摘要
Measurement of time series complexity and predictability is sometimes the cornerstone for proposing solutions to topology and congestion control problems in sensor networks. As a method of measuring time series complexity and predictability, multiscale entropy (MSE) has been widely applied in many fields. However, sample entropy, which is the fundamental component of MSE, measures the similarity of two subsequences of a time series with either zero or one, but without in-between values, which causes sudden changes of entropy values even if the time series embraces small changes. This problem becomes especially severe when the length of time series is getting short. For solving such the problem, we propose flexible multiscale entropy (FMSE), which introduces a novel similarity function measuring the similarity of two subsequences with full-range values from zero to one, and thus increases the reliability and stability of measuring time series complexity. The proposed method is evaluated on both synthetic and real time series, including white noise, 1/f noise and real vibration signals. The evaluation results demonstrate that FMSE has a significant improvement in reliability and stability of measuring complexity of time series, especially when the length of time series is short, compared to MSE and composite multiscale entropy (CMSE). The proposed method FMSE is capable of improving the performance of time series analysis based topology and traffic congestion control techniques.
更多查看译文
关键词
time series,complexity,sample entropy,flexible similarity criterion,flexible multiscale entropy,sensor network organizing,sensor network controlling
AI 理解论文
溯源树
样例
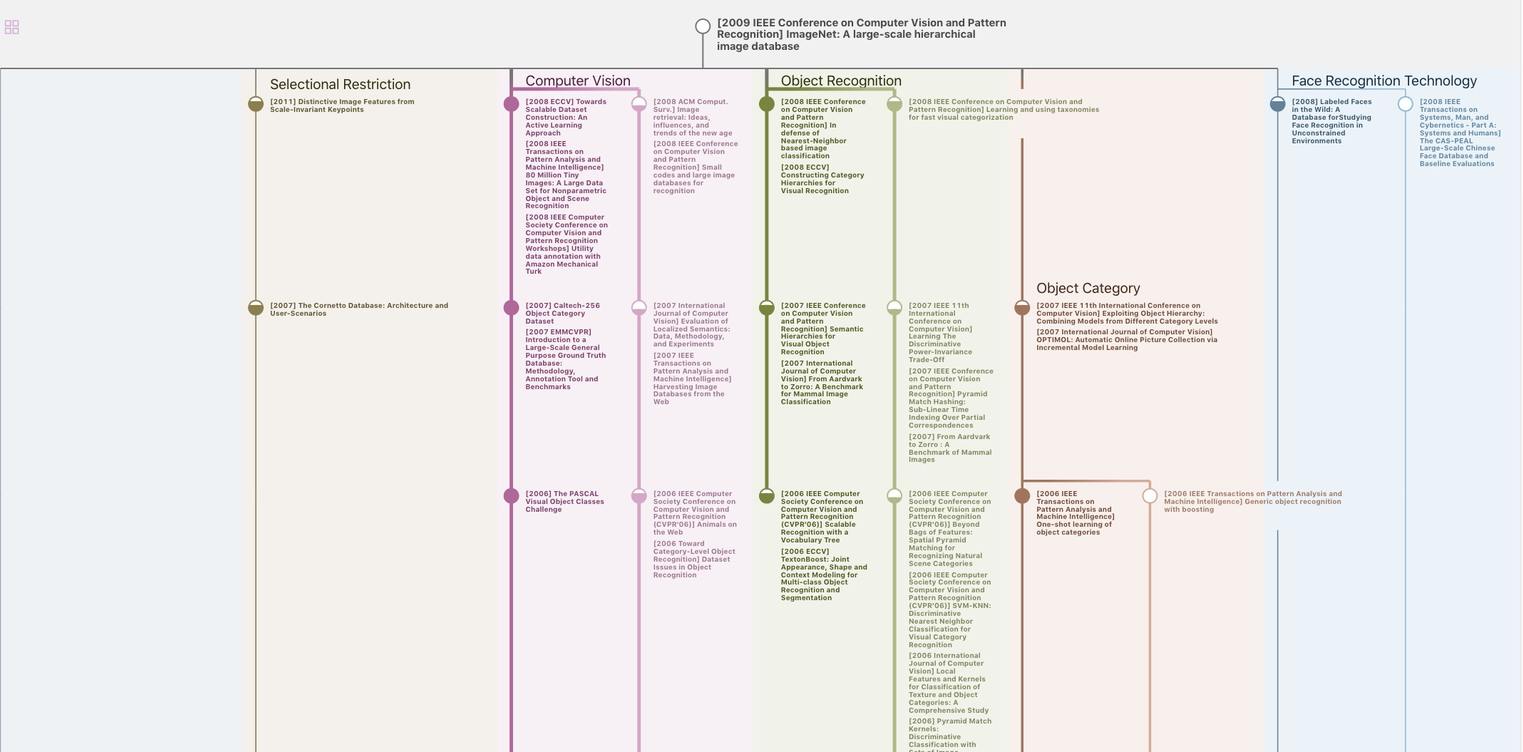
生成溯源树,研究论文发展脉络
Chat Paper
正在生成论文摘要