A Robust Random Forest-Based Approach for Heart Rate Monitoring Using Photoplethysmography Signal Contaminated by Intense Motion Artifacts.
SENSORS(2017)
摘要
The estimation of heart rate (HR) based on wearable devices is of interest in fitness. Photoplethysmography (PPG) is a promising approach to estimate HR due to low cost; however, it is easily corrupted by motion artifacts (MA). In this work, a robust approach based on random forest is proposed for accurately estimating HR from the photoplethysmography signal contaminated by intense motion artifacts, consisting of two stages. Stage 1 proposes a hybrid method to effectively remove MA with a low computation complexity, where two MA removal algorithms are combined by an accurate binary decision algorithm whose aim is to decide whether or not to adopt the second MA removal algorithm. Stage 2 proposes a random forest-based spectral peak-tracking algorithm, whose aim is to locate the spectral peak corresponding to HR, formulating the problem of spectral peak tracking into a pattern classification problem. Experiments on the PPG datasets including 22 subjects used in the 2015 IEEE Signal Processing Cup showed that the proposed approach achieved the average absolute error of 1.65 beats per minute (BPM) on the 22 PPG datasets. Compared to state-of-the-art approaches, the proposed approach has better accuracy and robustness to intense motion artifacts, indicating its potential use in wearable sensors for health monitoring and fitness tracking.
更多查看译文
关键词
heart rate (HR),photoplethysmography (PPG),motion artifacts (MA),random forest
AI 理解论文
溯源树
样例
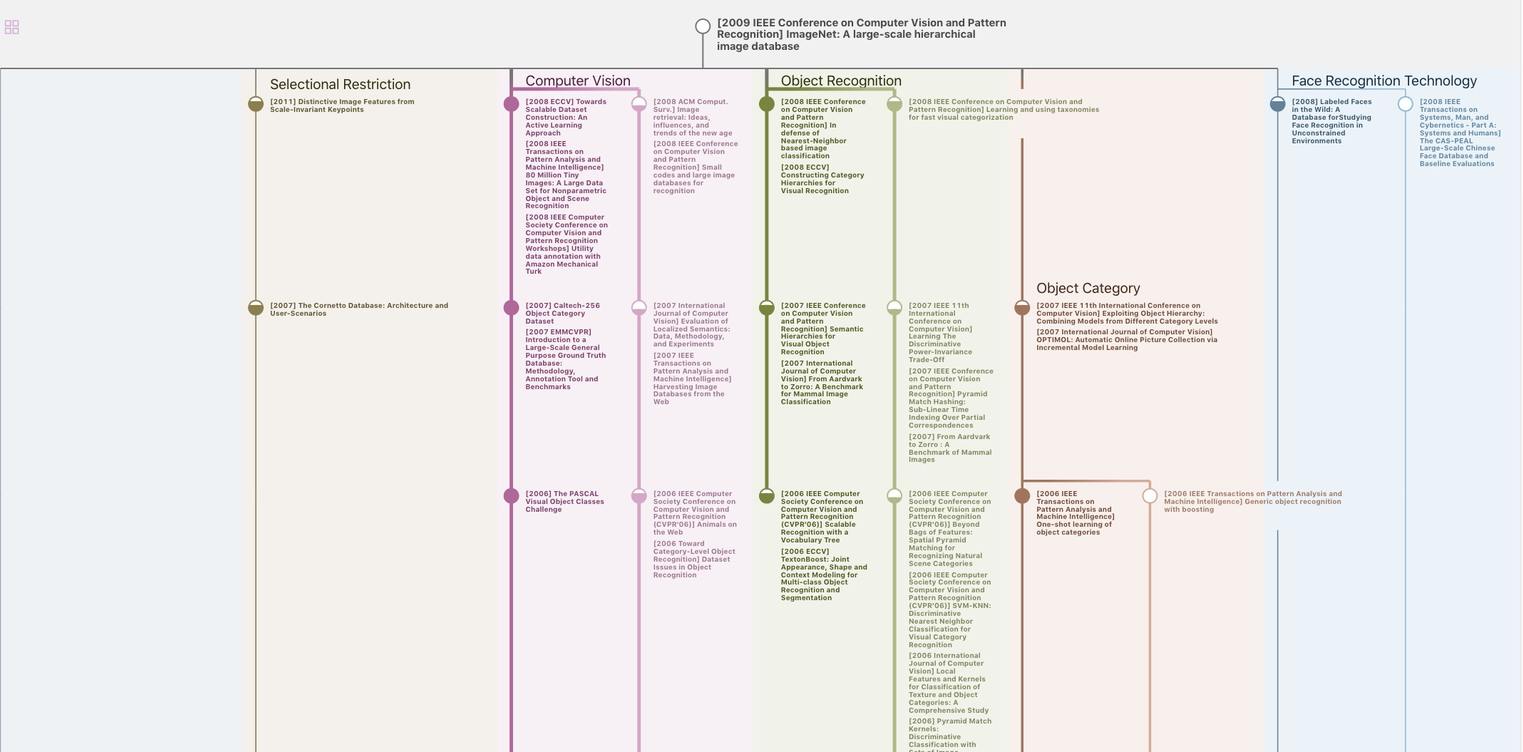
生成溯源树,研究论文发展脉络
Chat Paper
正在生成论文摘要