Crowded Scene Understanding by Deeply Learned Volumetric Slices.
IEEE Trans. Circuits Syst. Video Techn.(2017)
摘要
Crowd video analysis is one of the hallmark tasks of crowded scene understanding. While we observe a tremendous progress in image-based tasks with the rise of convolutional neural networks (CNNs), performance on video analysis has not (yet) attained the same level of success. In this paper, we introduce intuitive but effective temporal-aware crowd motion channels by uniformly slicing the video volume from different dimensions. Multiple CNN structures with different data-fusion strategies and weight-sharing schemes are proposed to learn the connectivity both spatially and temporally from these motion channels. To well demonstrate our deep model, we construct a new large-scale Who do What at someWhere crowd data set with 10 000 videos from 8257 crowded scenes, and build an attribute set with 94 attributes. Extensive experiments on crowd video attribute prediction demonstrate the effectiveness of our novel method over the state-of-the-art.
更多查看译文
关键词
World Wide Web,Neural networks,Feature extraction,Surveillance,Semantics,Image segmentation,Motion segmentation
AI 理解论文
溯源树
样例
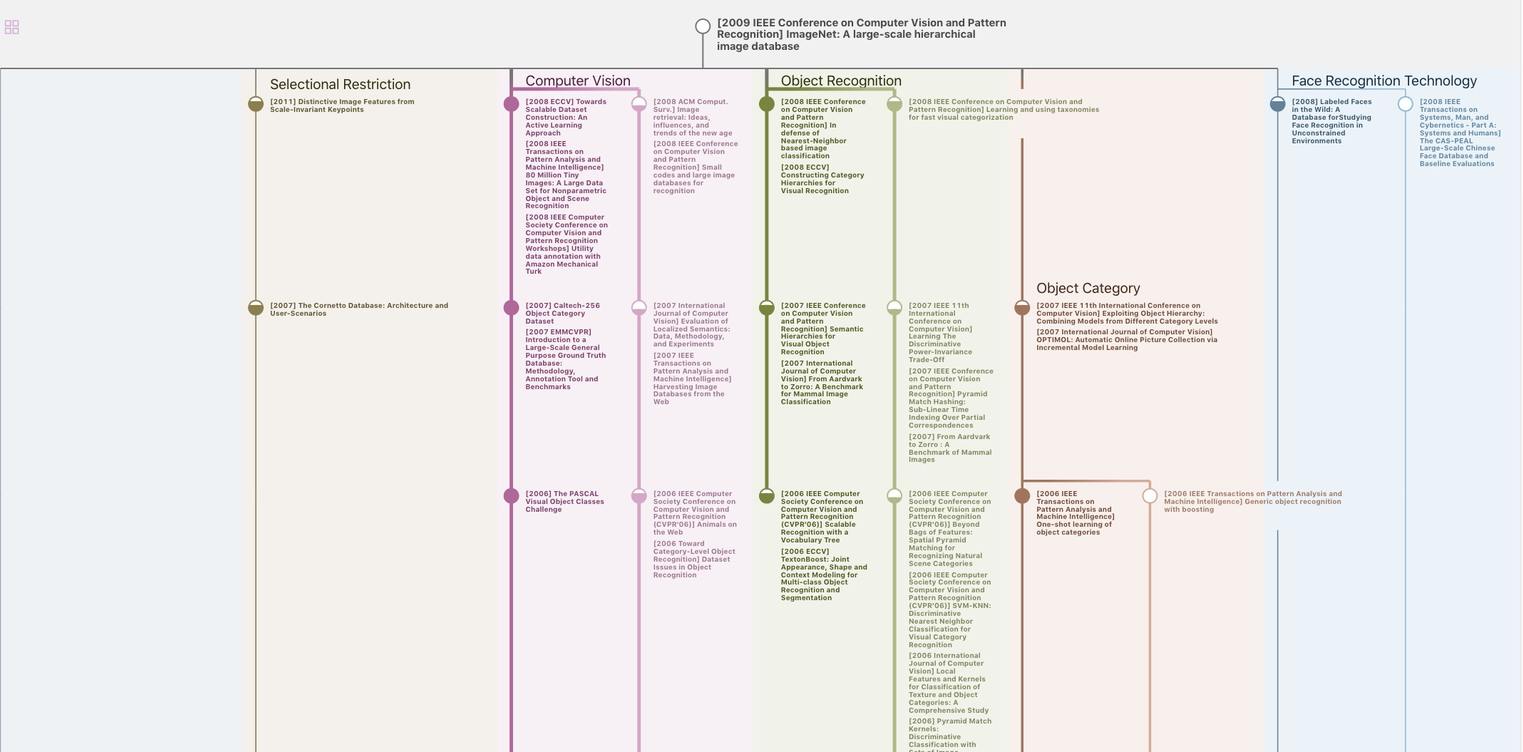
生成溯源树,研究论文发展脉络
Chat Paper
正在生成论文摘要