A Survey on Ensemble Learning for Data Stream Classification.
ACM Comput. Surv.(2017)
摘要
Ensemble-based methods are among the most widely used techniques for data stream classification. Their popularity is attributable to their good performance in comparison to strong single learners while being relatively easy to deploy in real-world applications. Ensemble algorithms are especially useful for data stream learning as they can be integrated with drift detection algorithms and incorporate dynamic updates, such as selective removal or addition of classifiers. This work proposes a taxonomy for data stream ensemble learning as derived from reviewing over 60 algorithms. Important aspects such as combination, diversity, and dynamic updates, are thoroughly discussed. Additional contributions include a listing of popular open-source tools and a discussion about current data stream research challenges and how they relate to ensemble learning (big data streams, concept evolution, feature drifts, temporal dependencies, and others).
更多查看译文
关键词
Ensemble learning,supervised learning,data stream classification
AI 理解论文
溯源树
样例
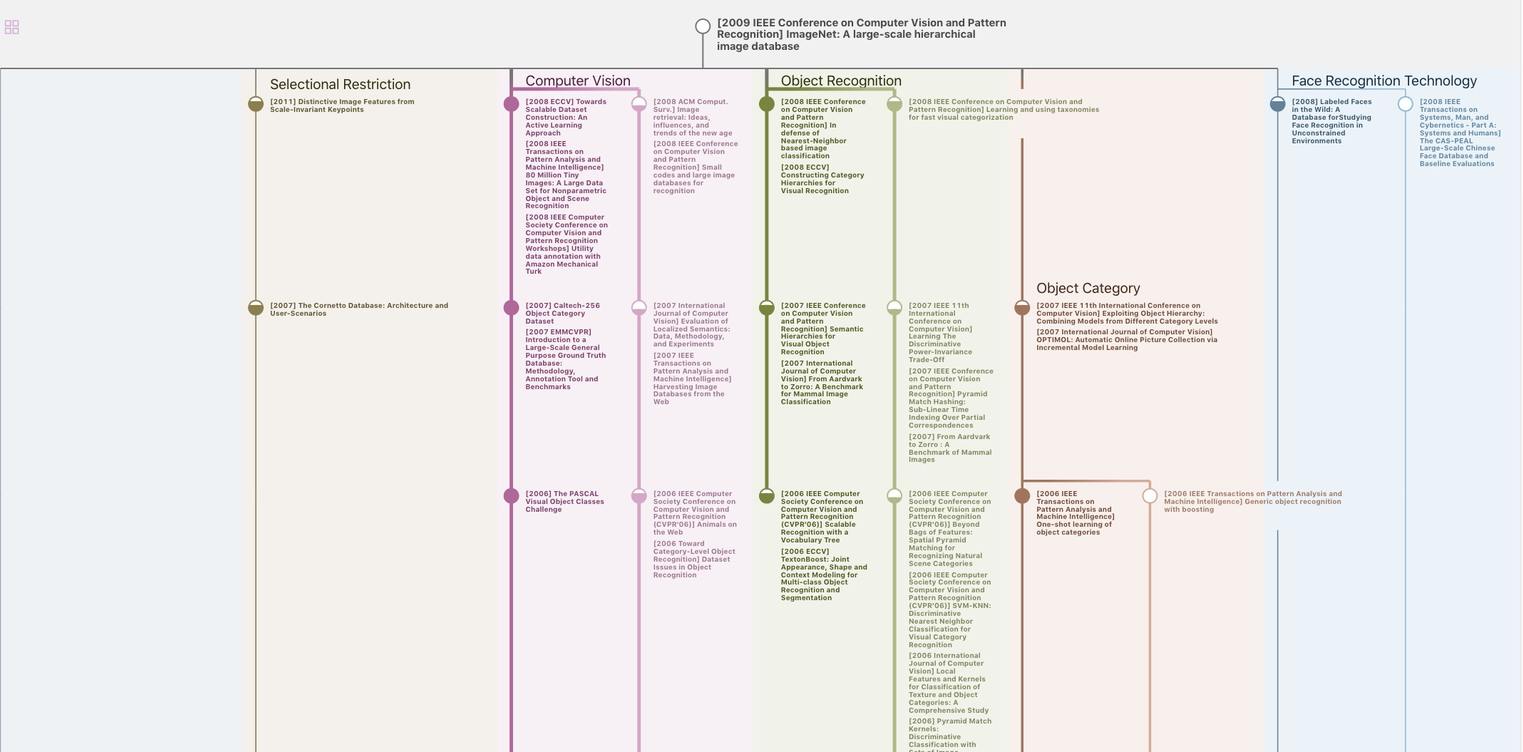
生成溯源树,研究论文发展脉络
Chat Paper
正在生成论文摘要