Joint Training Of Generic Cnn-Crf Models With Stochastic Optimization
COMPUTER VISION - ACCV 2016, PT II(2016)
摘要
We propose a new CNN-CRF end-to-end learning framework, which is based on joint stochastic optimization with respect to both Convolutional Neural Network (CNN) and Conditional Random Field (CRF) parameters. While stochastic gradient descent is a standard technique for CNN training, it was not used for joint models so far. We show that our learning method is (i) general, i. e. it applies to arbitrary CNN and CRF architectures and potential functions; (ii) scalable, i. e. it has a low memory footprint and straightforwardly parallelizes on GPUs; (iii) easy in implementation. Additionally, the unified CNN-CRF optimization approach simplifies a potential hardware implementation. We empirically evaluate our method on the task of semantic labeling of body parts in depth images and show that it compares favorably to competing techniques.
更多查看译文
关键词
Markov Chain Monte Carlo, Depth Image, Convolutional Neural Network, Markov Chain Monte Carlo Method, Conditional Random Field
AI 理解论文
溯源树
样例
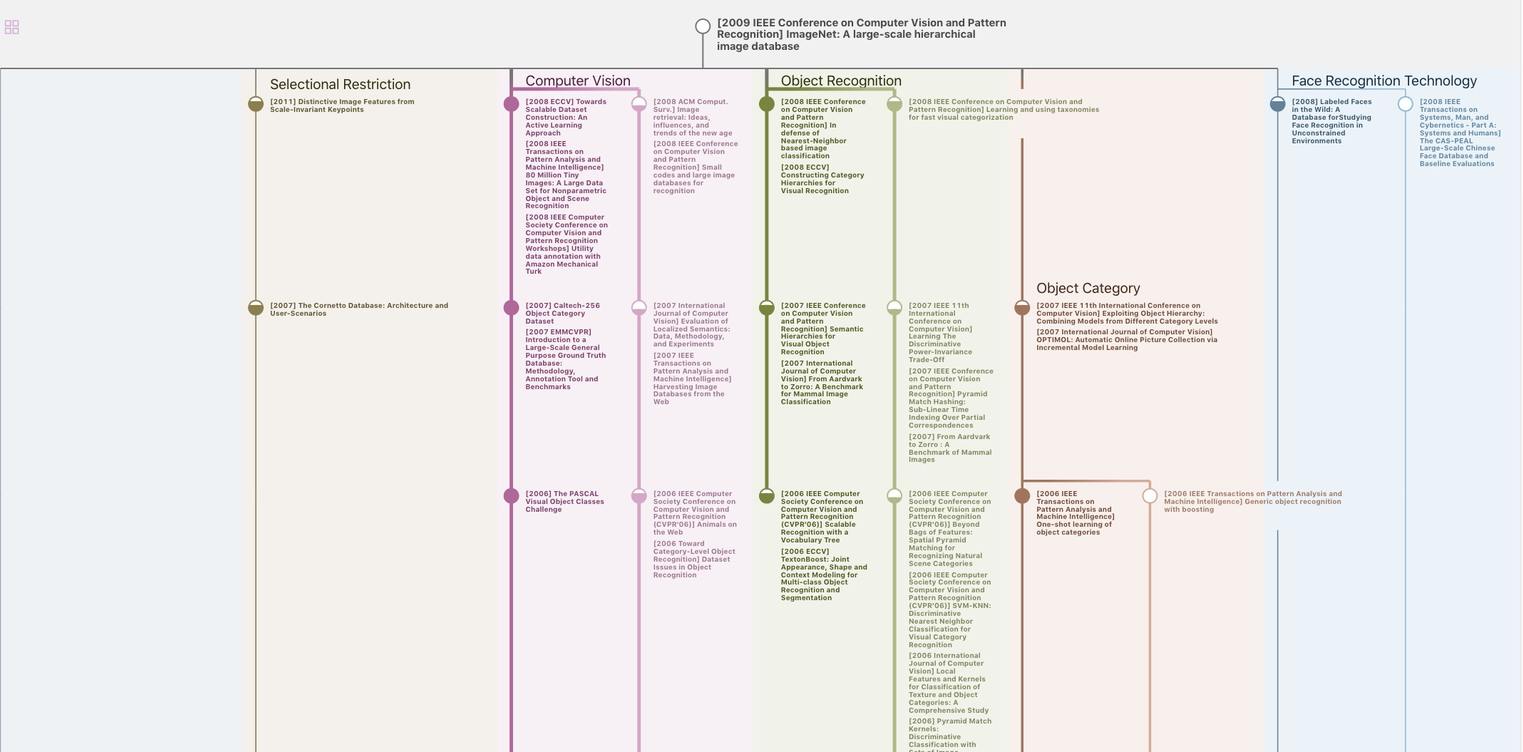
生成溯源树,研究论文发展脉络
Chat Paper
正在生成论文摘要