Probabilistic Multi-Agent Plan Recognition as Planning (P-Maprap): Recognizing Teams, Goals, and Plans from Action Sequences.
ICAART: PROCEEDINGS OF THE 9TH INTERNATIONAL CONFERENCE ON AGENTS AND ARTIFICIAL INTELLIGENCE, VOL 2(2017)
摘要
We extend Multi-agent Plan Recognition as Planning (MAPRAP) to Probabilistic MAPRAP (P-MAPRAP), which probabilistically identifies teams and their goals from limited observations of on-going individual agent actions and a description of actions and their effects. These methods do not rely on plan libraries, as such are infeasibly large and complex in multi-agent domains. Both MAPRAP and P-MAPRAP synthesize plans tailored to hypothesized team compositions and previous observations. Where MAPRAP prunes team-goal interpretations on optimality grounds, P-MAPRAP directs its search base on a likelihood ranking of interpretations, thus effectively reducing the synthesis effort needed without compromising recognition. We evaluate performance in scenarios that vary the number of teams, agent counts, initial states, goals, and observation errors, assuming equal base-rates. We measure accuracy, precision, and recall online to evaluate early stage recognition. Our results suggest that compared to MAPRAP, P-MAPRAP exhibits improved speed and recognition accuracy.
更多查看译文
关键词
Multi-Agent Systems,Plan Recognition
AI 理解论文
溯源树
样例
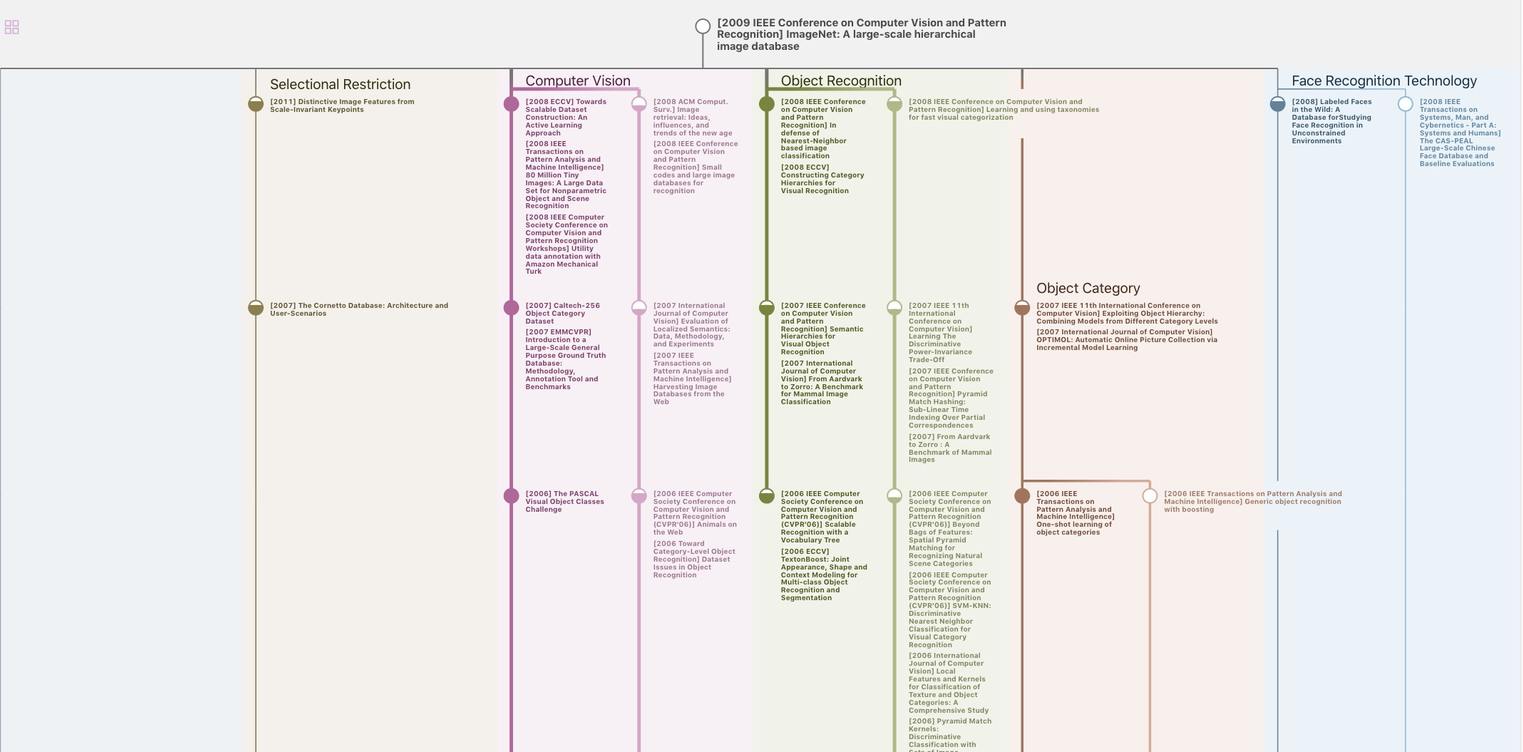
生成溯源树,研究论文发展脉络
Chat Paper
正在生成论文摘要