Improving data provenance reconstruction via a multi-level funneling approach
2016 IEEE 12th International Conference on e-Science (e-Science)(2016)
摘要
The ease with which data can be created, copied, modified, and deleted over the Internet has made it increasingly difficult to determine the source of web data. Data provenance, which provides information about the origin and lineage of a dataset, assists in determining its genuineness and trustworthiness. Several data provenance techniques record provenance when the data is created or modified. However, many existing datasets have no recorded provenance. Provenance Reconstruction techniques attempt to generate an approximate provenance in these datasets. Current reconstruction techniques require timing metadata to reconstruct provenance. In thats paper, we improve our multi-funneling technique, which combines existing techniques, including topic modeling, longest common subsequence, and genetic algorithm to achieve higher accuracy in reconstructing provenance without requiring timing metadata. In addition, we introduce novel funnels that are customized to the provided datasets, which further boosts precision and recall rates. We evaluated our approach with various experiments and compare the results of our approach with existing techniques. Finally, we present lessons learned, including the applicability of our approach to other datasets.
更多查看译文
关键词
data provenance,provenance reconstruction,Latent Dirichlet Allocation,Genetic Algorithm,Longest Common Subsequence,Statistical Re-clustering,Silhouette Coefficient
AI 理解论文
溯源树
样例
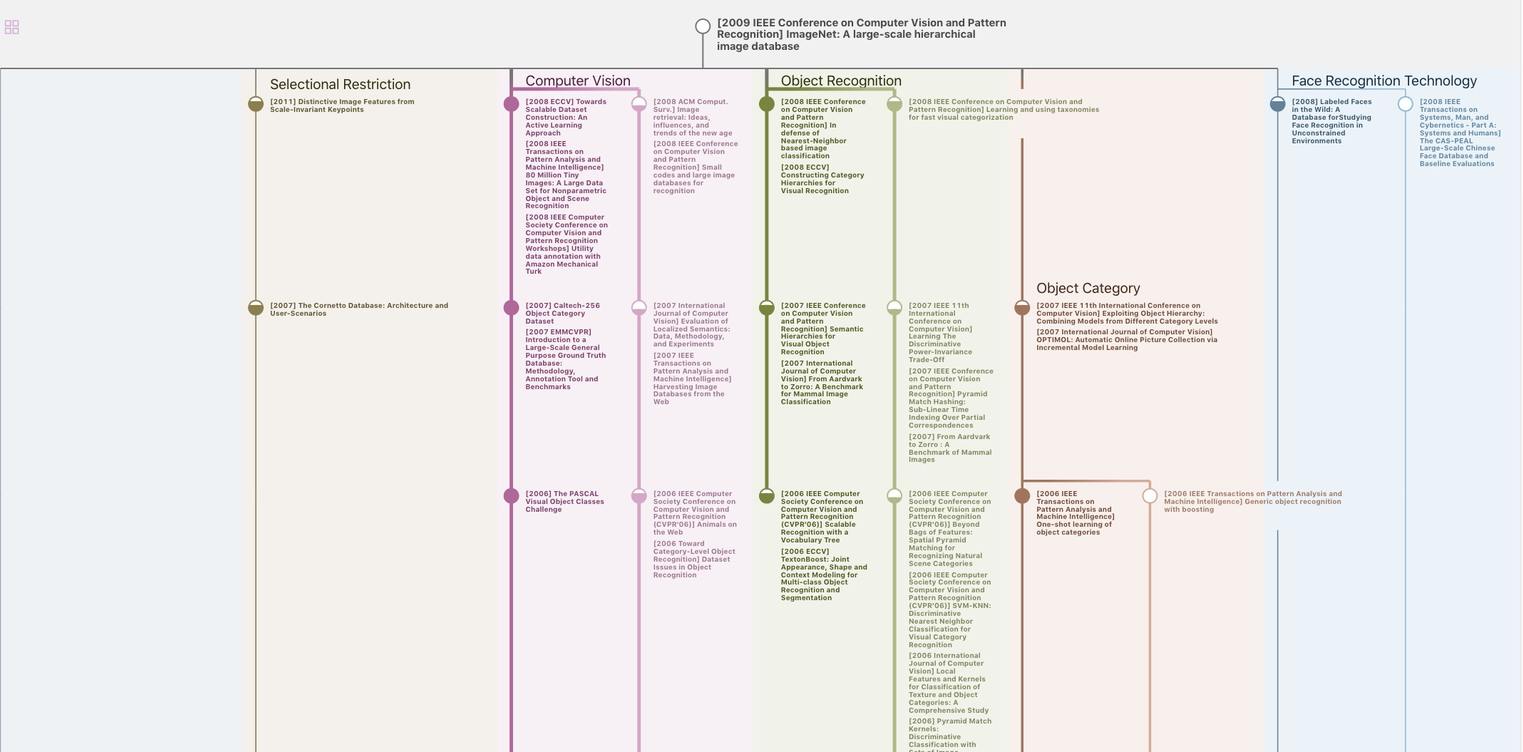
生成溯源树,研究论文发展脉络
Chat Paper
正在生成论文摘要