Detection of Recovery Patterns in Cluster Systems Using Resource Usage Data
2017 IEEE 22nd Pacific Rim International Symposium on Dependable Computing (PRDC)(2017)
摘要
The failure of large-scale distributed systems such as cluster systems has adverse effects on the performance of high-performance computing applications such as scientific applications. Techniques to handle these failures, such as checkpointing, typically incur a prohibitively high computational cost. To reduce or prevent the occurrences of such failures, system administrators have employed a divide and conquer approach to diagnosing the root-cause of such failures, in order to take corrective or preventive measures. Most times, event logs are the main sources of information about the failures. However, it is also important to be able to predict when the system is recovering to avoid such costly error handling. To this end, we present a novel technique, based on system resource usage information, to detect recovery runs. Our approach uses an unsupervised learning technique, namely change point detection, to predict recovery. We run our approach on data from Ranger Supercomputer System and the results are positive: our approach have an F-measure of 64%.
更多查看译文
关键词
Change point detection,resource usage data,recovery sequence,detection,large-scale HPC systems
AI 理解论文
溯源树
样例
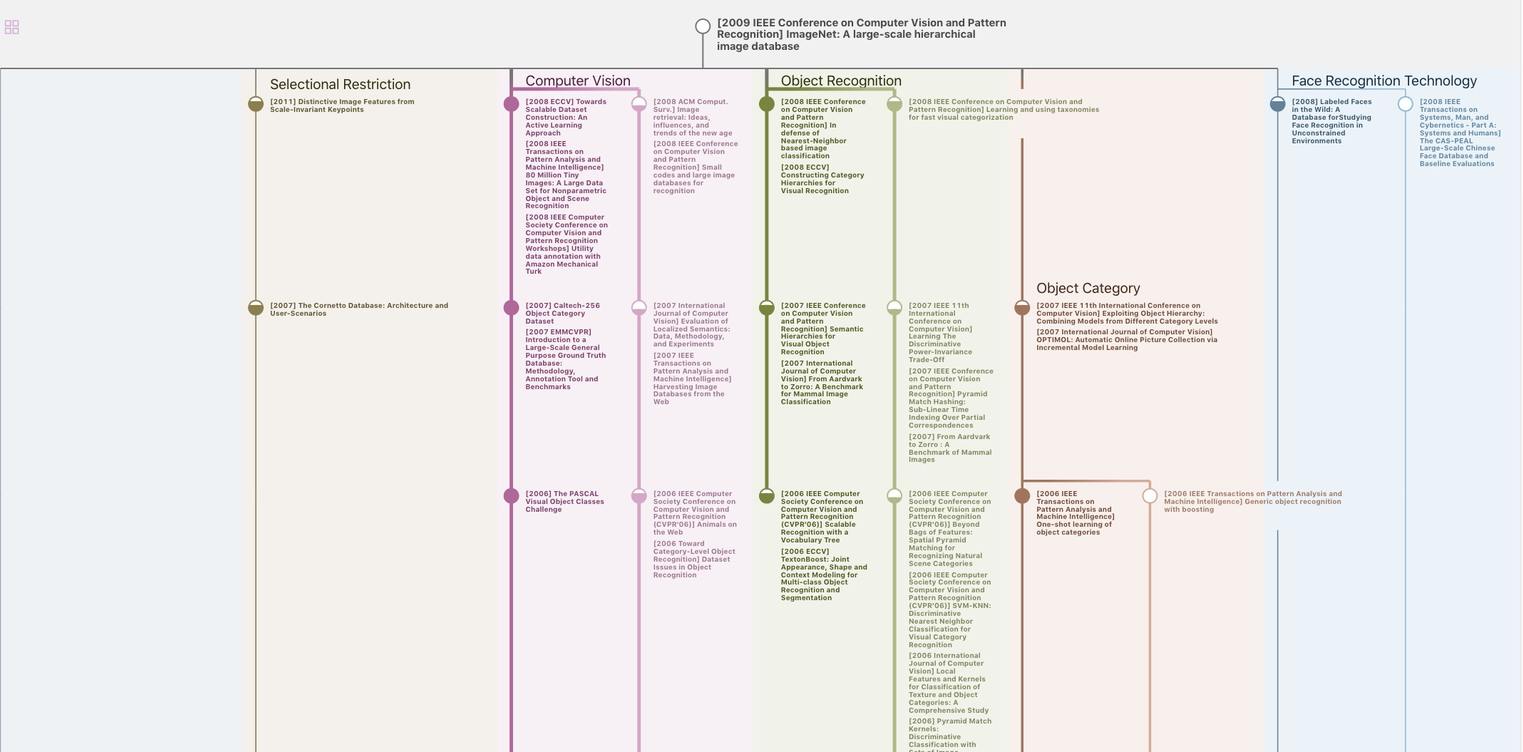
生成溯源树,研究论文发展脉络
Chat Paper
正在生成论文摘要