Hierarchical Zero-Shot Classification With Convolutional Neural Network Features And Semantic Attribute Learning
PROCEEDINGS OF THE FIFTEENTH IAPR INTERNATIONAL CONFERENCE ON MACHINE VISION APPLICATIONS - MVA2017(2017)
摘要
We examine hierarchical approaches to image classification problems that include categories for which we have no training examples. Building on prior work in hierarchical classification that optimizes the trade-off between depth in a tree and accuracy of placement, we compare the performance of multiple formulations of the problem on both previously seen (non-novel) and previously unseen (novel) classes. We use a subset of 150 object classes from the ImageNet ILSVRC2012 data set, for which we have 218 human-annotated semantic attribute labels and for which we compute deep convolutional features using the OVERFEAT network. We quantitatively evaluate several approaches, using input posteriors derived from distances to SVM classifier boundaries as well as input posteriors based on semantic attribute estimation. We find that the relative performances of the methods differ in non novel and novel applications and achieve information gains in novel applications through the incorporation of attribute-based posteriors.
更多查看译文
关键词
hierarchical zero-shot classification,convolutional neural network,semantic attribute learning,image classification,ImageNet ILSVRC2012 data set,human-annotated semantic attribute labels,OVERFEAT network,SVM classifier boundaries,semantic attribute estimation
AI 理解论文
溯源树
样例
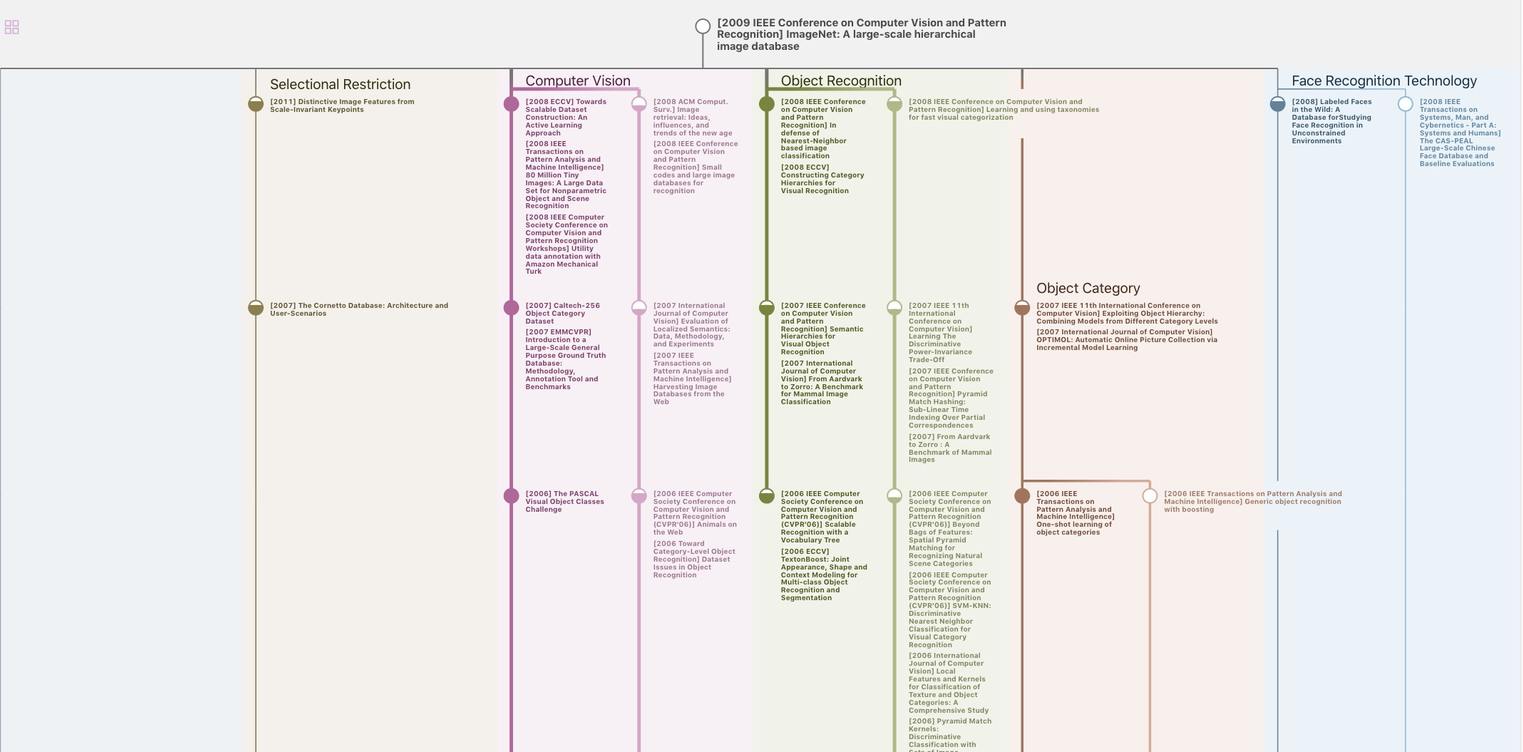
生成溯源树,研究论文发展脉络
Chat Paper
正在生成论文摘要