Manifolds Of Tool-Graspability In The Human Brain
2017 INTERNATIONAL WORKSHOP ON PATTERN RECOGNITION IN NEUROIMAGING (PRNI)(2017)
摘要
Neural representations for object recognition are difficult to construct because vision operates in high-dimensional space. This study aims to develop low-dimensional neural representations ("manifolds") that could contain either rotation or viewpoint information. In our experiments, four rotating tools were used as visual stimuli and brain activity was recorded using functional magnetic resonance imaging. We selected voxels whose signal changes were temporally correlated with the rotation task period and we proposed using principal component analysis to construct low-dimensional manifolds for these selected voxels. We hypothesized that manifolds for these voxels will be "loop-shaped" based on the rotation design featured in the experiment. Our results revealed two types of manifolds: voxels from lower-level visual areas (i.e. occipital pole, occipital fusiform gyrus) showed smooth "two-loops" shaped manifolds, which suggested that they treated those stimuli as rotating bars and they were sensitive to rotations instead of details of objects; voxels from higher-level visual areas didn't show obvious shaped manifolds, but higher-level visual areas (i.e. inferior temporal gyrus, middle temporal gyrus) were able to predict objects' category with accuracies above 0.53 for four-class classification. Our experiments demonstrated that for lower-level visual areas, the proposed manifolds structures could represent neural activities when participants were visualizing rotating tools. The proposed representation structures can shed light on rotation angle decoding. However, the manifolds structures may not be suitable for higher-level visual areas. Future studies should further differentiate the roles of the manifolds structures in lower-level vs. higher-level visual areas.
更多查看译文
关键词
functional magnetic resonance imaging, principal component analysis, pattern classification, manifolds
AI 理解论文
溯源树
样例
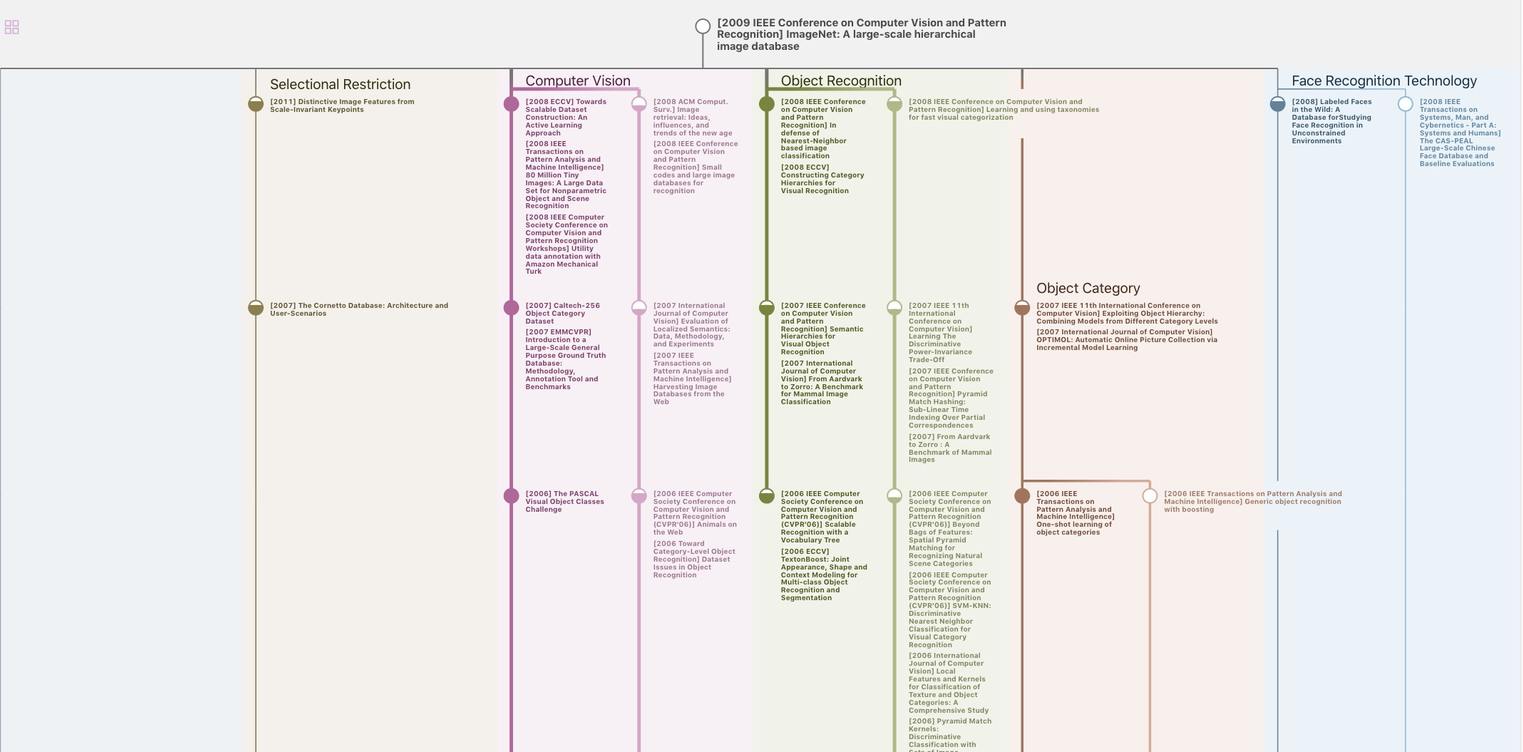
生成溯源树,研究论文发展脉络
Chat Paper
正在生成论文摘要