Initialization of 3D pose graph optimization using Lagrangian duality
ICRA(2017)
摘要
Pose Graph Optimization (PGO) is the de facto choice to solve the trajectory of an agent in Simultaneous Localization and Mapping (SLAM). The Maximum Likelihood Estimation (MLE) for PGO is a non-convex problem for which no known technique is able to guarantee a globally optimal solution under general conditions. In recent years, Lagrangian duality has proved suitable to provide good, frequently tight relaxations of the hard PGO problem through convex Semidefinite Programming (SDP). In this work, we build from the state-of-the-art Lagrangian relaxation [1] and contribute a complete recovery procedure that, given the (tractable) optimal solution of the relaxation, provides either the optimal MLE solution if the relaxation is tight, or a remarkably good feasible guess if the relaxation is non-tight, which occurs in specially challenging PGO problems (very noisy observations, low graph connectivity, etc.). In the latter case, when used for initialization of local iterative methods, our approach outperforms other state-of-the-art approaches converging to better solutions. We support our claims with extensive experiments.
更多查看译文
关键词
3D pose graph optimization,Lagrangian duality,PGO,simultaneous localization and mapping,SLAM,maximum likelihood estimation,MLE,convex semidefinite programming,SDP,local iterative methods
AI 理解论文
溯源树
样例
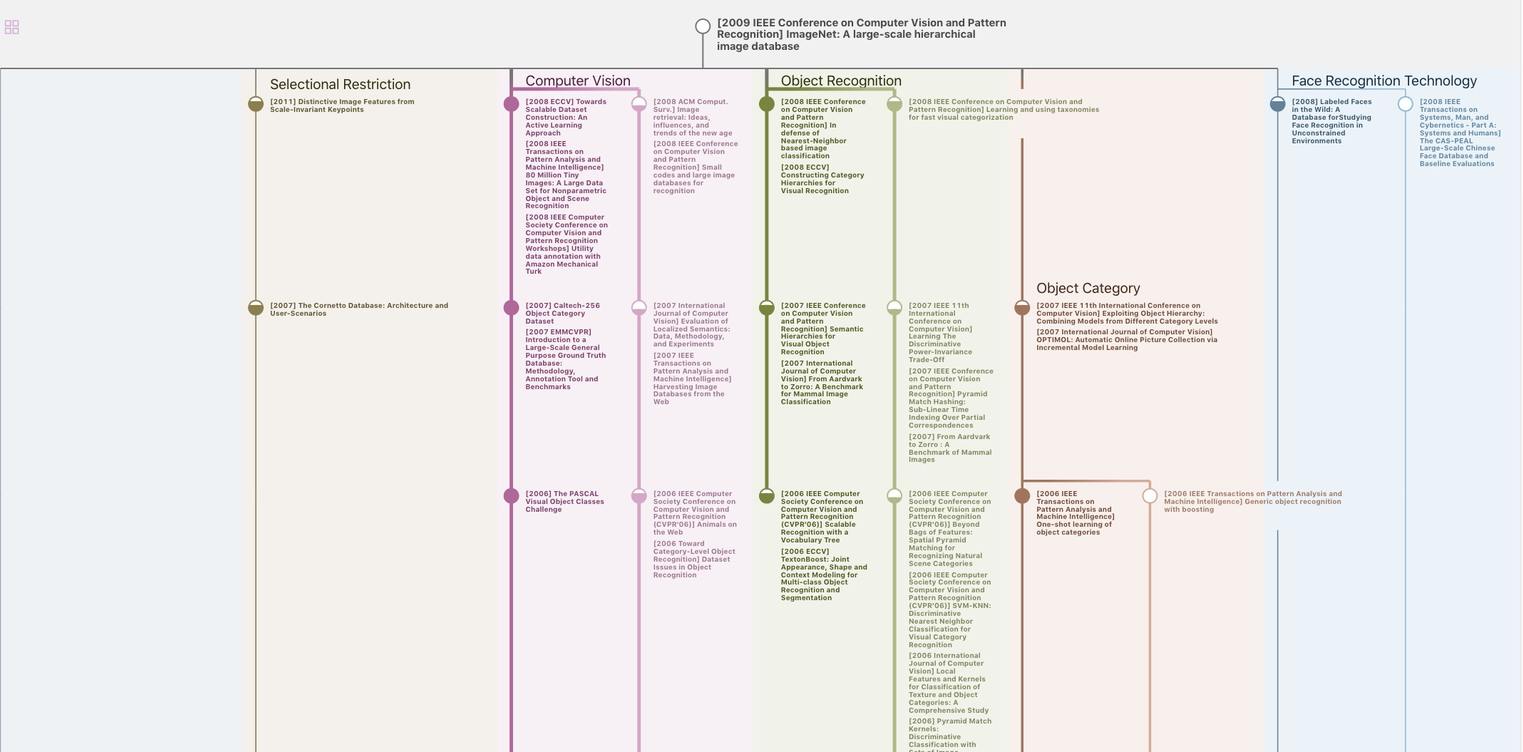
生成溯源树,研究论文发展脉络
Chat Paper
正在生成论文摘要