Privacy Preference Modeling And Prediction In A Simulated Campuswide Iot Environment
2017 IEEE INTERNATIONAL CONFERENCE ON PERVASIVE COMPUTING AND COMMUNICATIONS (PERCOM)(2017)
摘要
With the advent of the Internet of Things (IoT), users are more likely to have privacy concerns since their personal information could be collected, analyzed, and utilized without notice by the networked IoT devices and services. Users may want to control all such activities by explicitly expressing their privacy preferences. However, it is becoming increasingly difficult for users to do so, not only because of the cognitive burden of continuously making privacy decisions for IoT services, but also because IoT devices have no, or only very restricted, user interfaces. Intelligent software helping users make better privacy decisions will be an important component of privacy preserving IoT environments. In order to construct such a component, we aim to verify whether it will be possible to computationally model and predict users' privacy preferences in IoT. To that end, we survey 172 participants in a simulated campuswide IoT environment about their privacy preferences regarding hypothetical personal information tracking scenarios. Then, we cluster the scenarios based on the survey responses, arriving at four clusters with distinct associated privacy preferences. Based on the clustering results, we uncover contextual factors that induce privacy violations in IoT. Finally, we build machine learning models to predict users' privacy decisions, using both contextual information and the corresponding cluster membership as training data. The final trained model shows 77% accuracy in predicting users' decisions whether or not to allow the respective IoT scenario.
更多查看译文
关键词
IoT,privacy,preference modeling,experience sampling,Google Glass,K-modes clustering,conditional inference tree
AI 理解论文
溯源树
样例
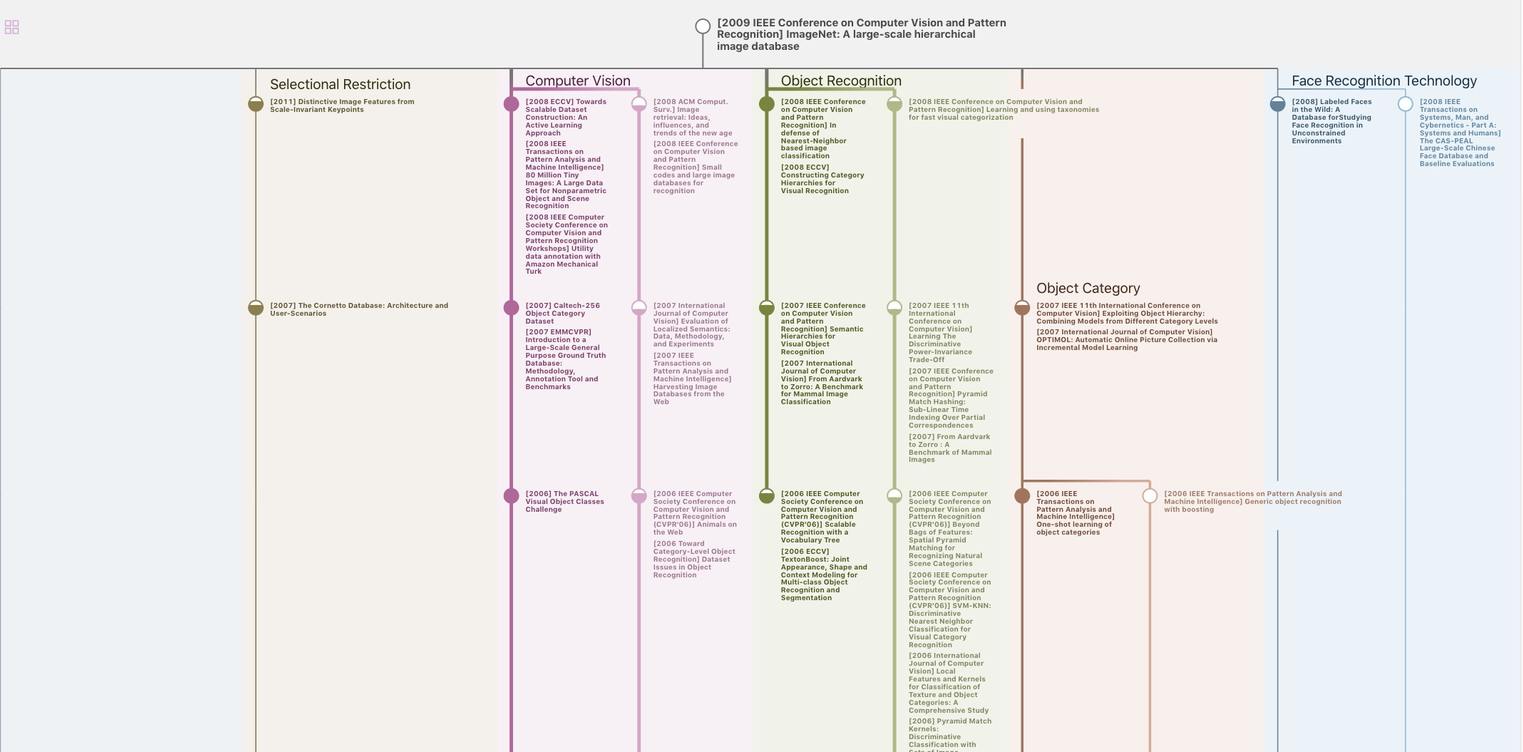
生成溯源树,研究论文发展脉络
Chat Paper
正在生成论文摘要