Classification data using outlier detection method in Wireless sensor networks
2017 13TH INTERNATIONAL WIRELESS COMMUNICATIONS AND MOBILE COMPUTING CONFERENCE (IWCMC)(2017)
摘要
For classification data, we use Wireless sensor networks (WSNs) as hardware for collecting data from harsh environments and controlling important events in phenomena. To evaluate the quality of a sensor and its network, we use the accuracy of sensor readings as surely one of the most important measures. Therefore, for anomalous measurement, real time detection is required to guarantee the quality of data collected by these networks. In this case, the task amounts to create a useful model based on KPCA to recognize data as normal or outliers. On account of the attractive capability, KPCA-based methods have been extensively investigated, and have shown excellent performance. So, to extract relevant feature for classification and to prevent from the events, we use KPCA based on Mahalanobis kernel as a preprocessing step. In the original space, the totality of computation is done thus saving computing time. Then the classification was done on real Intel Berkeley data collecting from urban area. Compared to a standard KPCA, the results show that our method are specially designed to be used in the field of wireless sensor networks (WSNs).
更多查看译文
关键词
component, Classsification data, Wireless Sensor Networks (WSNs), Mahalanobis kernel, Kernel Principal Component Analysis (KPCA), Outlier Detection
AI 理解论文
溯源树
样例
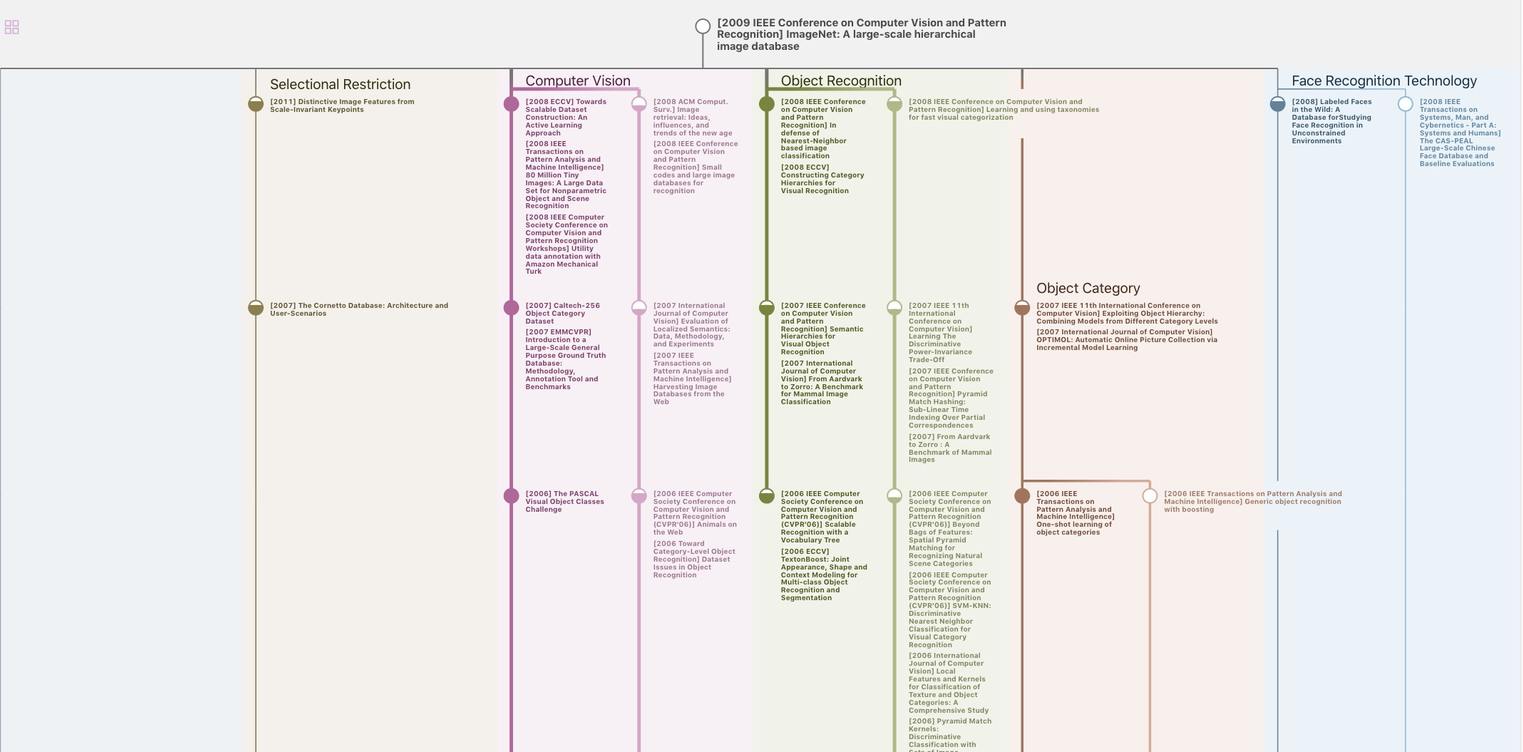
生成溯源树,研究论文发展脉络
Chat Paper
正在生成论文摘要