Using Viterbi And Kalman To Detect Topological Changes In Dynamic Networks
2017 AMERICAN CONTROL CONFERENCE (ACC)(2017)
摘要
This paper addresses the issue of determining the causal link structure of a network of dynamical systems from time series. Such problem is relevant in contexts where time series data is abundant but causal relationships are unknown. Complex systems, where interaction can rarely be derived from first principles constitute a main target of this effort. Applications abound in biology, environmental sciences and interconnected infrastructures, to name a few. Current methods are promising in their ability to determine link structure, and even provide guarantees under qualified assumptions; however, they are limited in their ability to track changes in causal structure over time. Such changes may result from external or unmodeled disruptions, such as cyber attacks or the formation or dissolution of business relationships. In this paper we formulate a model for changing networks and show that it is a generalization of a Hidden Markov Model (HMM). We then provide an algorithm capable of detecting topological changes in dynamical networks, and we compute the probability distributions of transition times.
更多查看译文
关键词
dynamic networks,topological changes detection,causal link structure,time series data,complex systems,biology,environmental sciences,interconnected infrastructures,link structure,hidden Markov model,HMM,probability distributions,transition times,Viterbi,Kalman
AI 理解论文
溯源树
样例
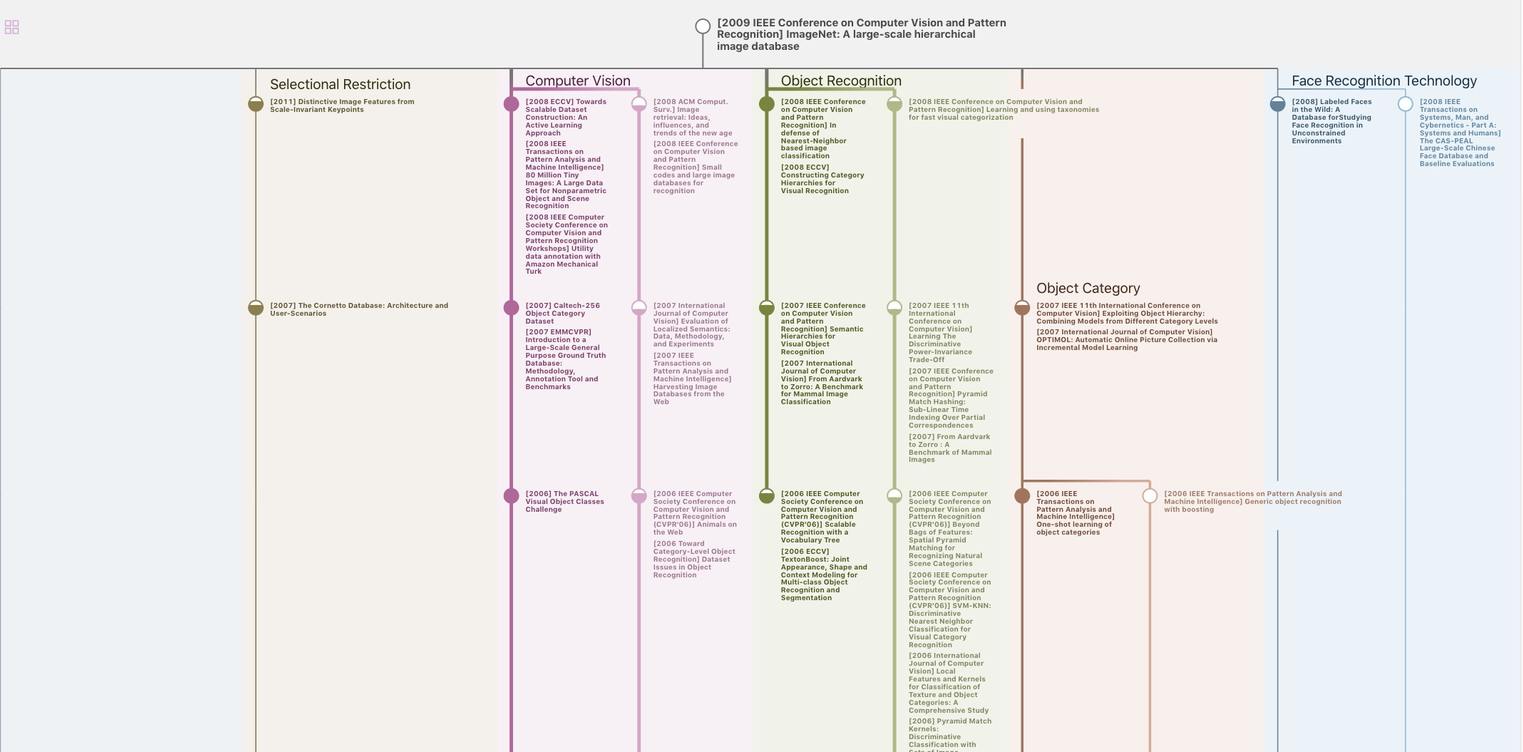
生成溯源树,研究论文发展脉络
Chat Paper
正在生成论文摘要