Medical Data Machine Translation Evaluation Based on Dependency N-Grams.
Lecture Notes in Computer Science(2017)
摘要
Machine Translation is increasingly applied to medical cross-lingual data processing. In order to evaluate the quality of machine translation, automatic evaluation approaches like BLEU and NIST, most of which are n-gram based metrics, are widely used besides costly human evaluation. Current evaluation approaches merely make surface linguistic comparisons between the candidate and reference translations. Further-more the domain features such medical terms, dependent and cohesive relations in and among sentences in medical documents should be taken into account when evaluating translations. However severe noises are imported into the procedure when faulty machine translations are parsed using the current syntactic parsers. Therefore using the noisy parsing results to compare with references affects the improvement of evaluation even though the deep processing is incorporated. To lessen noises as well as grasp the main meaning of a sentence, the paper proposes to extract the dependency n-grams only based on dependency parsing of reference translations. Dependency n-grams are stemmed and extended according to linguistic rules and then viewed as the key points for quality evaluation. The score of candidate translation is computed according to the count of dependency n-grams loose matching. Also the penalty of short translation and the clip count of the highest frequency of dependency n-grams are incorporated in the final score of the candidate. Experiments on our translation datasets show the evaluation based on dependency n-grams significantly outperforms the metric of BLEU and NSIT. This approach is also significantly better than the related research which employs dependency relation parsing in evaluation.
更多查看译文
关键词
Medical data,Machine Translation Quality Evaluation,Dependency relation parsing,Dependency n-grams
AI 理解论文
溯源树
样例
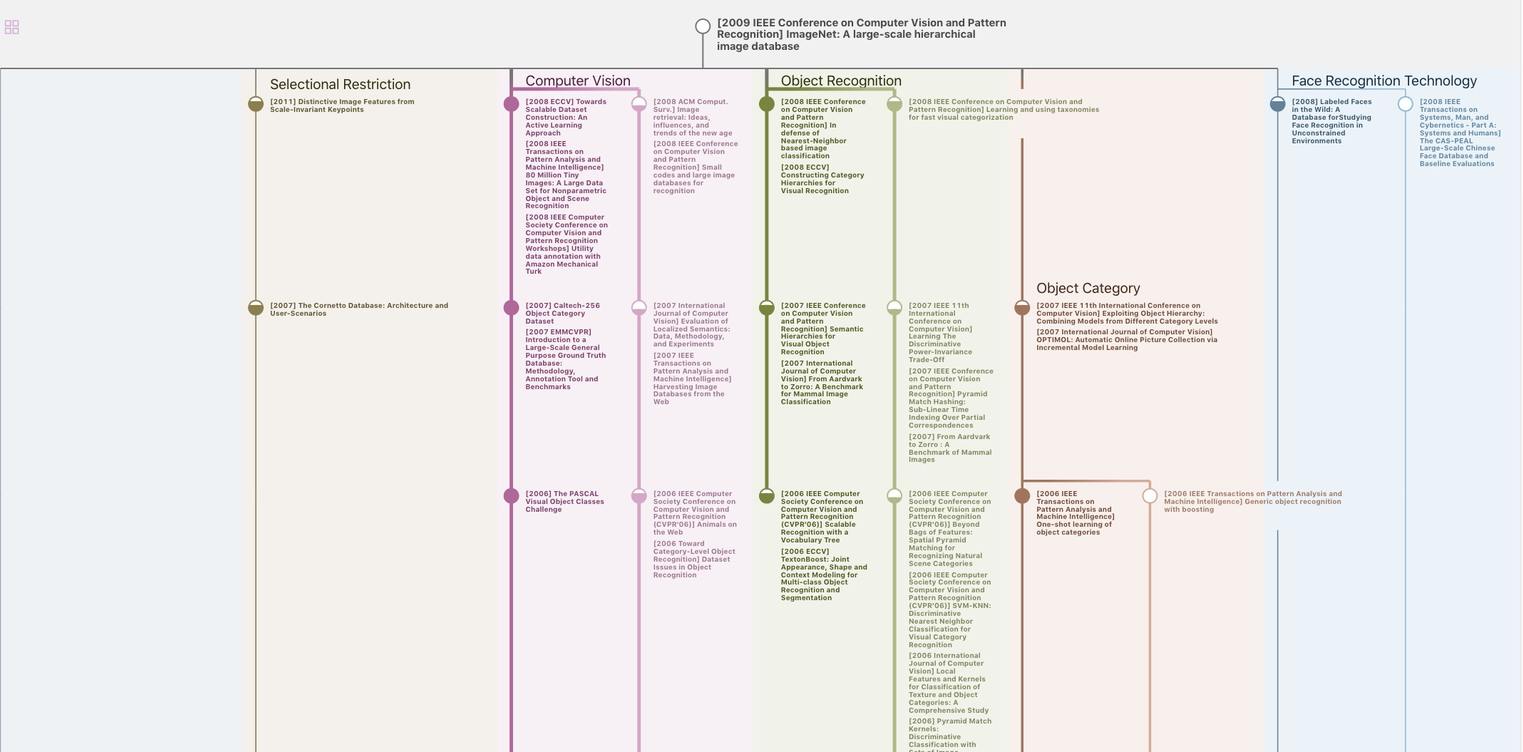
生成溯源树,研究论文发展脉络
Chat Paper
正在生成论文摘要