Privacy Preserving Anonymity For Periodical Srs Data Publishing
2017 IEEE 33RD INTERNATIONAL CONFERENCE ON DATA ENGINEERING (ICDE 2017)(2017)
摘要
Nowadays, spontaneous reporting systems (SRS) have been widely established to collect adverse drug events for ADR detection and analysis, e.g., the FDA Adverse Event Reporting System (FAERS). The SRS data are provided to the researchers, even open to the public, to foster the research of ADR detection and analysis. Normally, SRS data contains personal information and some sensitive value such as indication. It is necessary to de-identify the SRS data for preventing the disclosure of individual privacy before the data are published. Although there have been many different privacy preserving models and anonymization methods in the literature, they are not suitable for protecting SRS data from disclosure due to some features of SRS data. As such, we previously have proposed a privacy model called MS(k, theta*)-bounding and the associated algorithm MS-Anonymization. In the real world, the SRS data is dynamically growing and needs to be published periodically, which thwarts our single-release-focus method, i.e., MS(k, theta*)bounding. In this paper, we present some potential attacks on periodically published SRS data and propose a new privacy model called PPMS(k, theta*)-bounding and the associated PPMS-Anonymization algorithm. Experimental results on selected FAERS datasets show that our new method can prevent privacy disclosure from the attacks in periodical data publishing scenario with reasonable sacrifice of data utility and acceptable deviation to the strength of ADR signals.
更多查看译文
关键词
adverse drug reaction,data anonymization,incremental data publishing,privacy preserving data publishing,spontaneous reporting system
AI 理解论文
溯源树
样例
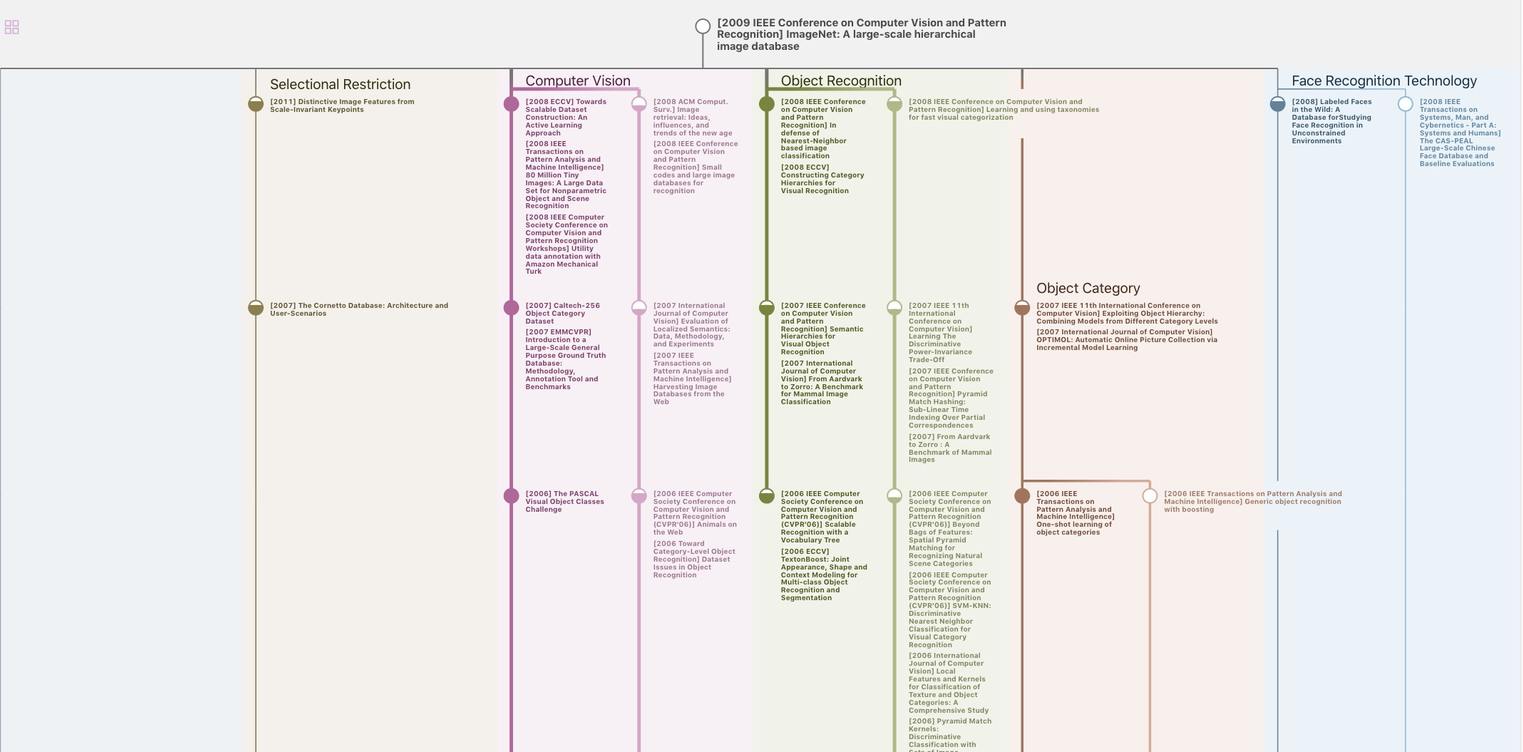
生成溯源树,研究论文发展脉络
Chat Paper
正在生成论文摘要