Identifying Directional Connections In Brain Networks Via Multi-Kernel Granger Models
2017 IEEE INTERNATIONAL CONFERENCE ON ACOUSTICS, SPEECH AND SIGNAL PROCESSING (ICASSP)(2017)
摘要
Granger causality based approaches are popular in unveiling directed interactions among brain regions. The present work advocates a multi-kernel based nonlinear model for obtaining the effective connectivity between brain regions, by wedding the merits of partial correlation in undirected topology identification with the ability of partial Granger causality (PGC) to estimate edge directionality. The premise is that existing linear PGC approaches may be inadequate for capturing certain dependencies, whereas available nonlinear connectivity models lack data adaptability that multi-kernel learning methods can offer. The proposed approach is tested on both synthetic and real resting-state fMRI data, with the former illustrating the gains in directed edge presence detection performance, as compared to existing PGC methods, and with the latter highlighting differences in the estimated test statistics.
更多查看译文
关键词
fMRI, partial Granger causality, partial correlation, kernel-based regression, multiple kernel learning
AI 理解论文
溯源树
样例
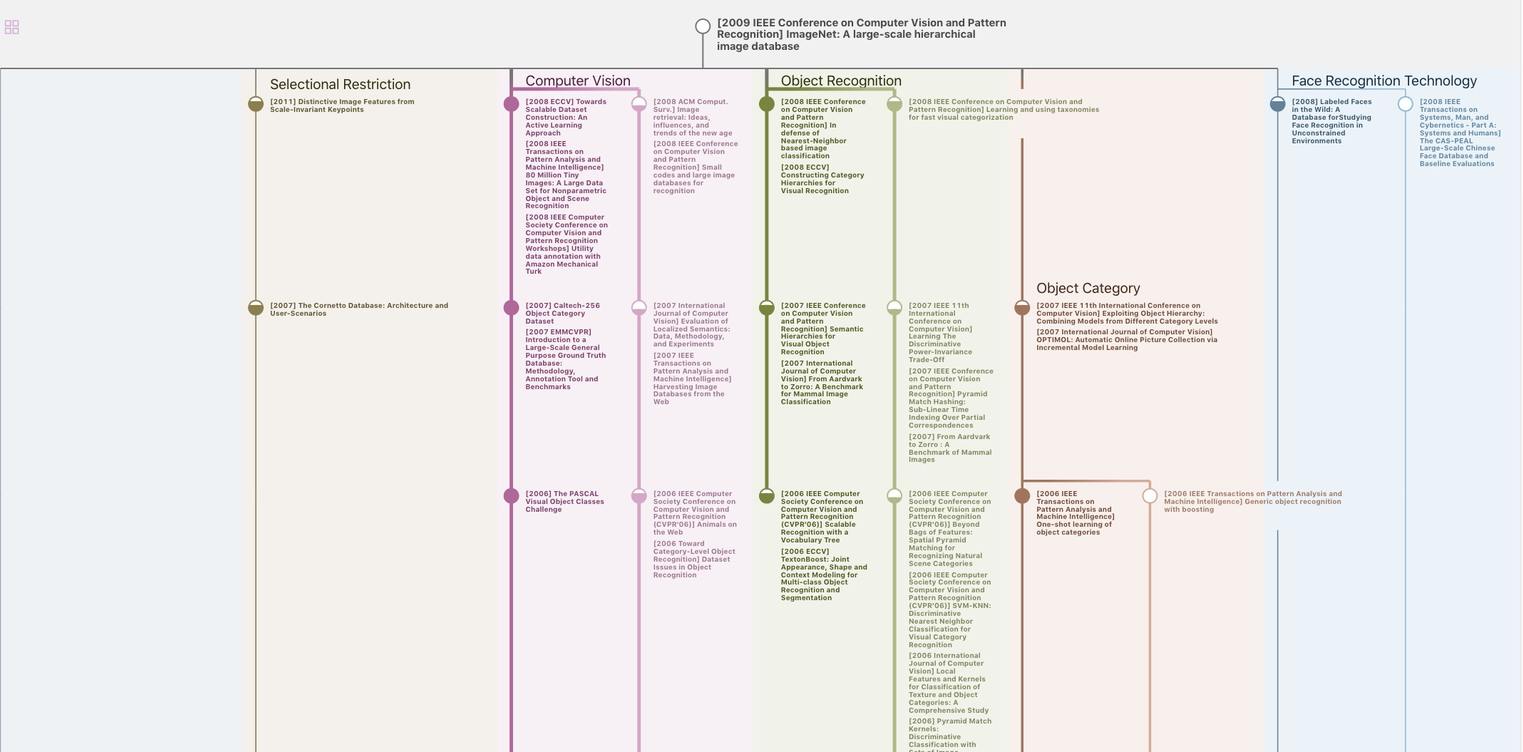
生成溯源树,研究论文发展脉络
Chat Paper
正在生成论文摘要