A Diagonal-Augmented Quasi-Newton Method With Application To Factorization Machines
2017 IEEE INTERNATIONAL CONFERENCE ON ACOUSTICS, SPEECH AND SIGNAL PROCESSING (ICASSP)(2017)
摘要
We present a novel quasi-Newton method for convex optimization, in which the Hessian estimates are based not only on the gradients, but also on the diagonal part of the true Hessian matrix (which can often be obtained with reasonable complexity). The new algorithm is based on the well known Broyden-Fletcher-Goldfarb-Shanno (BFGS) algorithm and has similar complexity. The proposed Diagonal-Augmented BFGS (DA-BFGS) method is shown to be stable and achieves a super-linear convergence rate in a local neighborhood of the optimal argument. Numerical experiments on logistic regression and factorization machines problems showcase that DA-BFGS consistently outperforms the baseline BFGS and Newton algorithms.
更多查看译文
关键词
Quasi-Newton methods, partial Hessian information, factorization machines
AI 理解论文
溯源树
样例
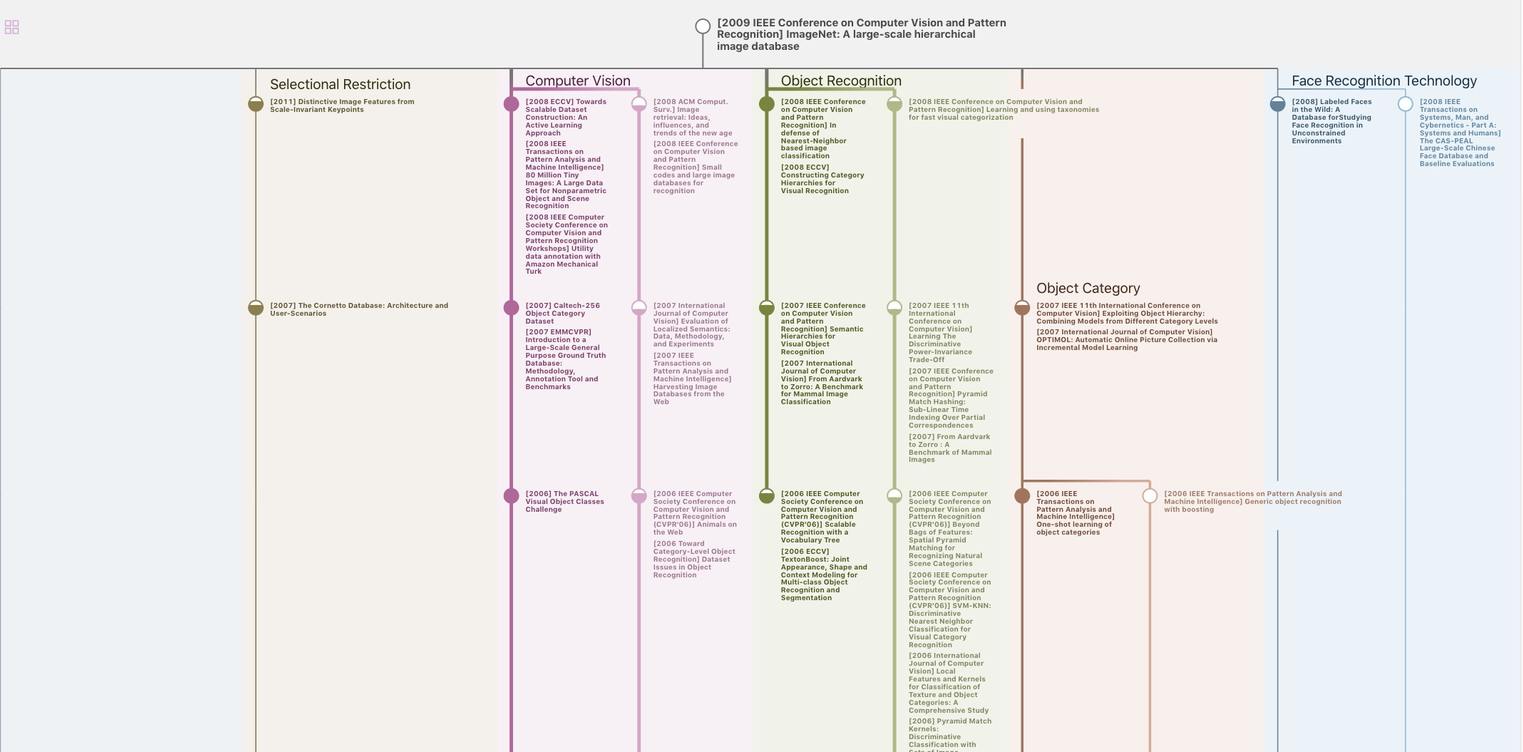
生成溯源树,研究论文发展脉络
Chat Paper
正在生成论文摘要