Two-Dimensional Pca Hashing And Its Extension
2016 23RD INTERNATIONAL CONFERENCE ON PATTERN RECOGNITION (ICPR)(2016)
摘要
Recently, hash algorithms catch amounts of sights in the field of machine learning. Most existing hash methods directly utilize a vector, which can be piped by the column of image matrix, as a unit and adopt some feature extraction functions to project the original data into generally shorter fixedlength values or characters. Then each of these projected real values is quantized or hashed into zero-one bit by a thresholding, such as locality sensitive hashing (LSH) and principal component analysis hashing (PCAH). However, the plain elongation of images may cause the curse of dimensionality. In this paper, different with PCAH method, the two dimensional (2D) images are directly used to extract the feature by two-dimensional principal component analysis (2DPCA) and 2DPCAH performs hashing using these 2DPCA-extracted data. Furthermore, starting with 2DPCA-extracted data, we apply iterative quantization (ITQ) technique, which aims to finding a rotation matrix so as to minimize the quantization error of mapping these data to a zerocentered binary hypercube. The experimental results indicate that 2DPCAH, 2DPCA-RR and 2DPCA-ITQ are competitive compared with traditional PCAH and other classic methods.
更多查看译文
关键词
PCAH,Two dimension,ITQ,Hashing
AI 理解论文
溯源树
样例
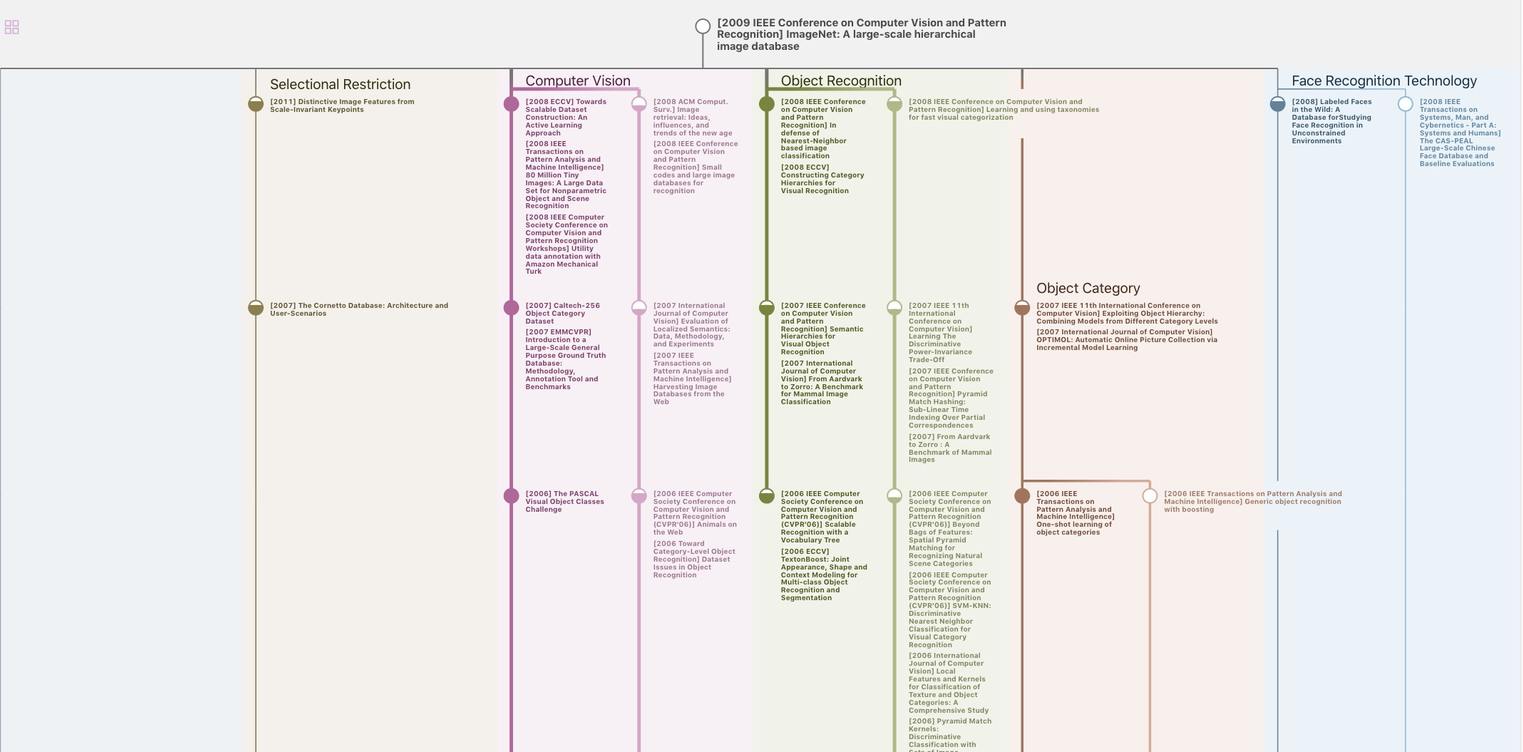
生成溯源树,研究论文发展脉络
Chat Paper
正在生成论文摘要