A Bayesian Part-Based Approach To 3d Human Pose And Camera Estimation
2016 23RD INTERNATIONAL CONFERENCE ON PATTERN RECOGNITION (ICPR)(2016)
摘要
We present a Bayesian framework for estimating 3D human pose and camera from a single RGB image. We develop a generative model where a 3D pose is rendered onto an image (via the camera), which then generates a detection probability map for each body part. We represent a human pose with a set of 3D cylinders in space, one for each body part, and we place kinematic and self-intersection priors on the model. Importantly, we use a graphics engine (e.g., OpenGL) to render the pose, and use its built-in capabilities for color blending to efficiently compute the likelihood of the model given the observed probability maps, which are obtained by running a convolutional neural network classifier on a test image. We explore the space of 3D poses and camera configurations via the Hybrid Monte Carlo algorithm, with sampling moves designed specifically for this problem. We train the parameters of our prior and likelihood distributions using annotated poses from the CMU mocap database, and test our algorithm on two benchmark datasets, where we compare performance against state-of-the-art methods. Additionally, we demonstrate the flexibility of our framework by incorporating a likelihood function for depth images and showing the associated performance gains.
更多查看译文
关键词
Bayesian part-based approach,3D human pose estimation,camera estimation,single RGB image,detection probability map,3D cylinders,self-intersection priors,graphics engine,probability maps,convolutional neural network classifier,hybrid Monte Carlo algorithm,likelihood distributions,CMU mocap database
AI 理解论文
溯源树
样例
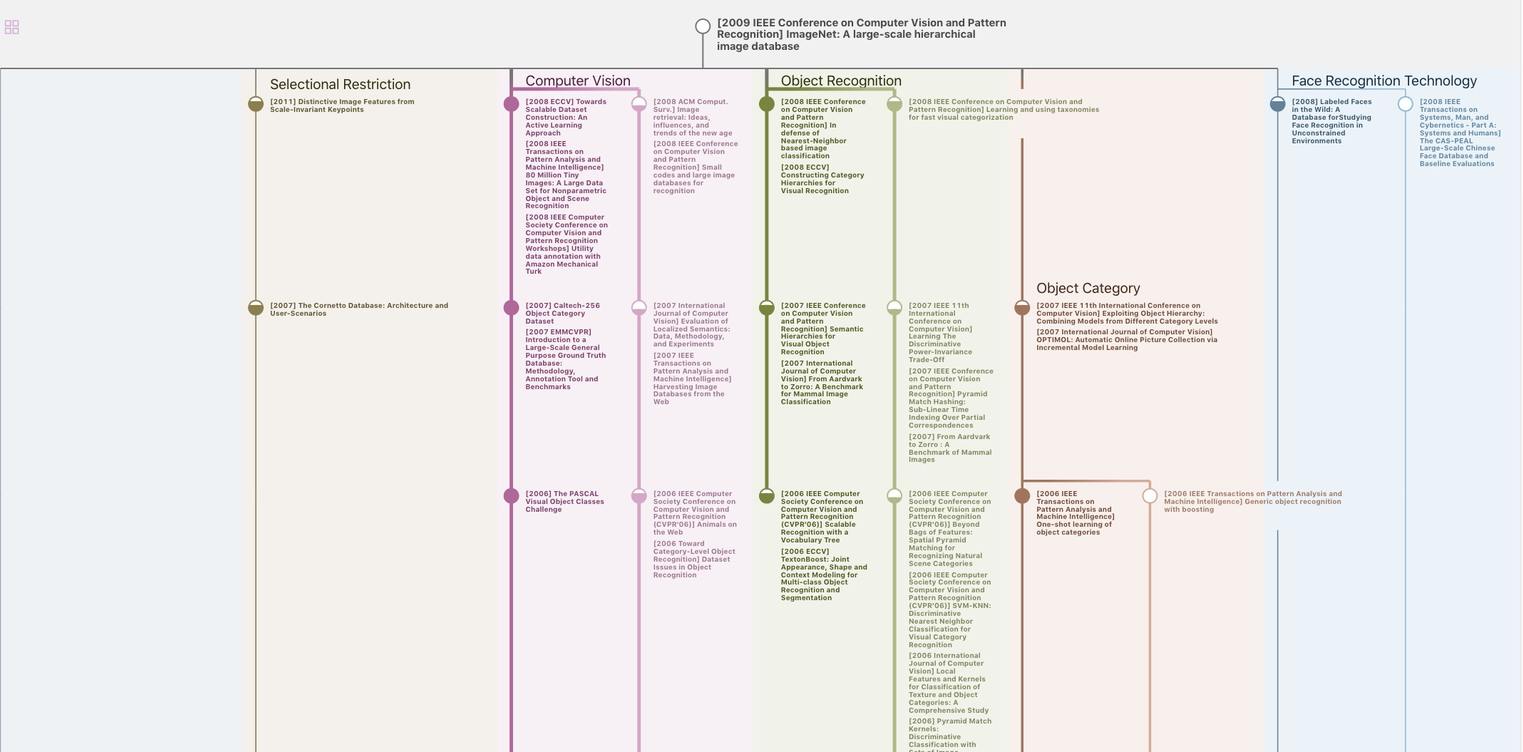
生成溯源树,研究论文发展脉络
Chat Paper
正在生成论文摘要