Object Segmentation Using Low-Rank Representation with Multiple Block-Diagonal Priors
International Conference on Pattern Recognition(2016)
摘要
This paper addresses the problem of segmenting objects for natural images by leveraging multiple segmentation methods. Existing image segmentation algorithms mostly partition the image into some coherent segments instead of extracting the object entirely. We observe that basic elements (e.g., superpixels) in the common segment produced by many methods are highly-correlated - they generally belong to the same object and lead to a low-rank structure. To make use of this information, this paper presents a novel approach to learn a low-rank affinity by leveraging various algorithms for segmenting the objects. First, starting from the segments produced using various algorithms, the label information for basic elements of an image, segmentation-to-superpixel label, is generated. Second, the derived labels are used to construct the multiple block-diagonal priors which are integrated into the subsequent affinity learning process. Finally the segmentation result is achieved by applying the spectral clustering technique on the obtained affinity matrix. Comprehensive experiments on MSRC, Alpert's and Berkeley segmentation datasets validate that our proposed approach achieves superior results as compared to each individual method. Moreover, our method is shown to be competitive in comparison to the state-of-the-art methods.
更多查看译文
关键词
object segmentation,low-rank representation,multiple block-diagonal priors,natural image segmentation,low-rank affinity structure,image segmentation-to-superpixel label generation,affinity learning process,spectral clustering technique
AI 理解论文
溯源树
样例
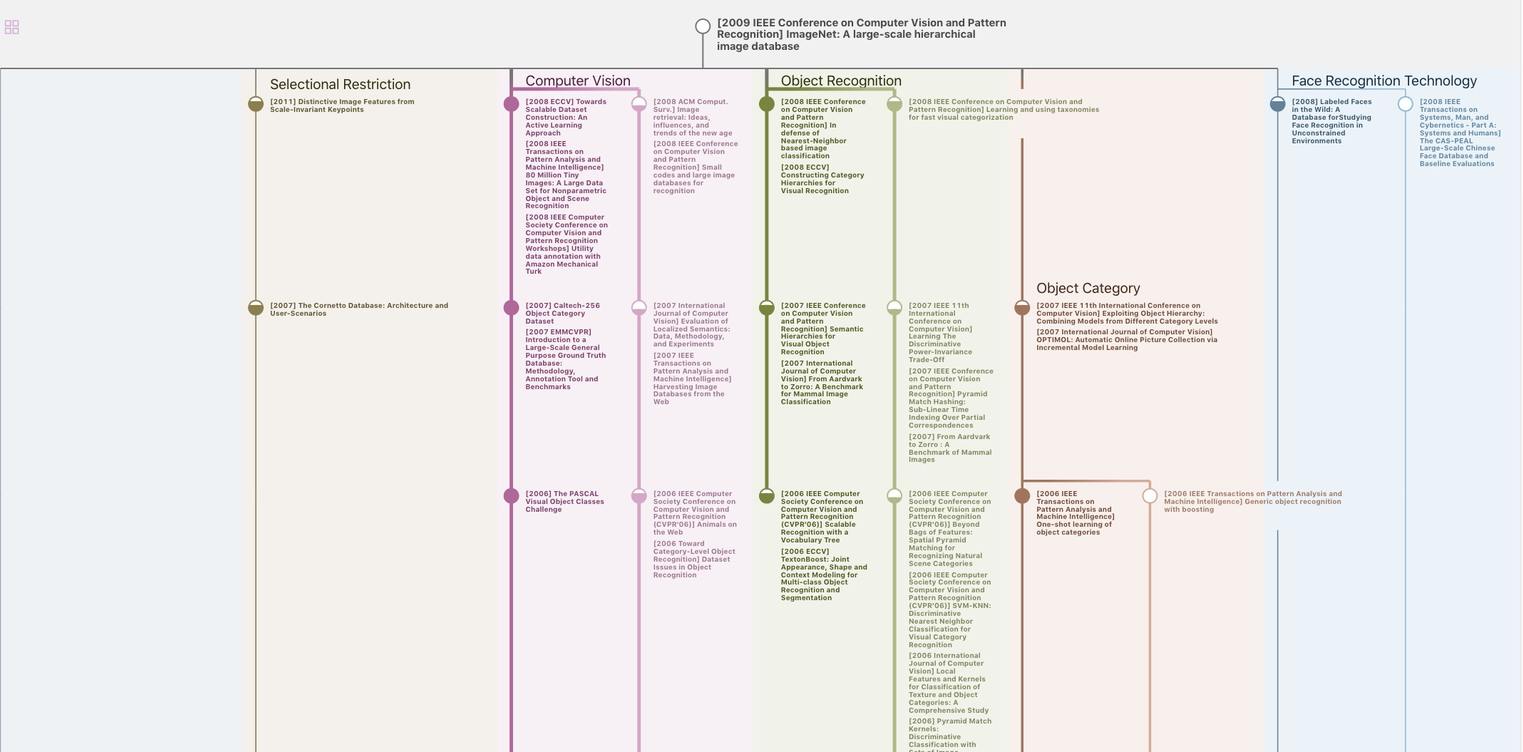
生成溯源树,研究论文发展脉络
Chat Paper
正在生成论文摘要