Neural Network-Based Full-Reference Image Quality Assessment
2016 PICTURE CODING SYMPOSIUM (PCS)(2016)
摘要
This paper presents a full-reference (FR) image quality assessment (IQA) method based on a deep convolutional neural network (CNN). The CNN extracts features from distorted and reference image patches and estimates the perceived quality of the distorted ones by combining and regressing the feature vectors using two fully connected layers. The CNN consists of 12 convolution and max-pooling layers; activation is done by a rectifier activation function (ReLU). The overall IQA score is computed by aggregating the patch quality estimates. Three different feature combination methods and two aggregation approaches are proposed and evaluated in this paper. Experiments are performed on the LIVE and TID2013 databases. On both databases linear Pearson correlations superior to state-of-the-art IQA methods are achieved.
更多查看译文
关键词
full-reference image quality assessment,FR IQA method,convolutional neural network,CNN,reference image patches,feature vectors,max-pooling layers,rectifier activation function,ReLU,IQA score,feature combination methods,aggregation approaches,LIVE databases,TID2013 databases,databases linear Pearson correlations
AI 理解论文
溯源树
样例
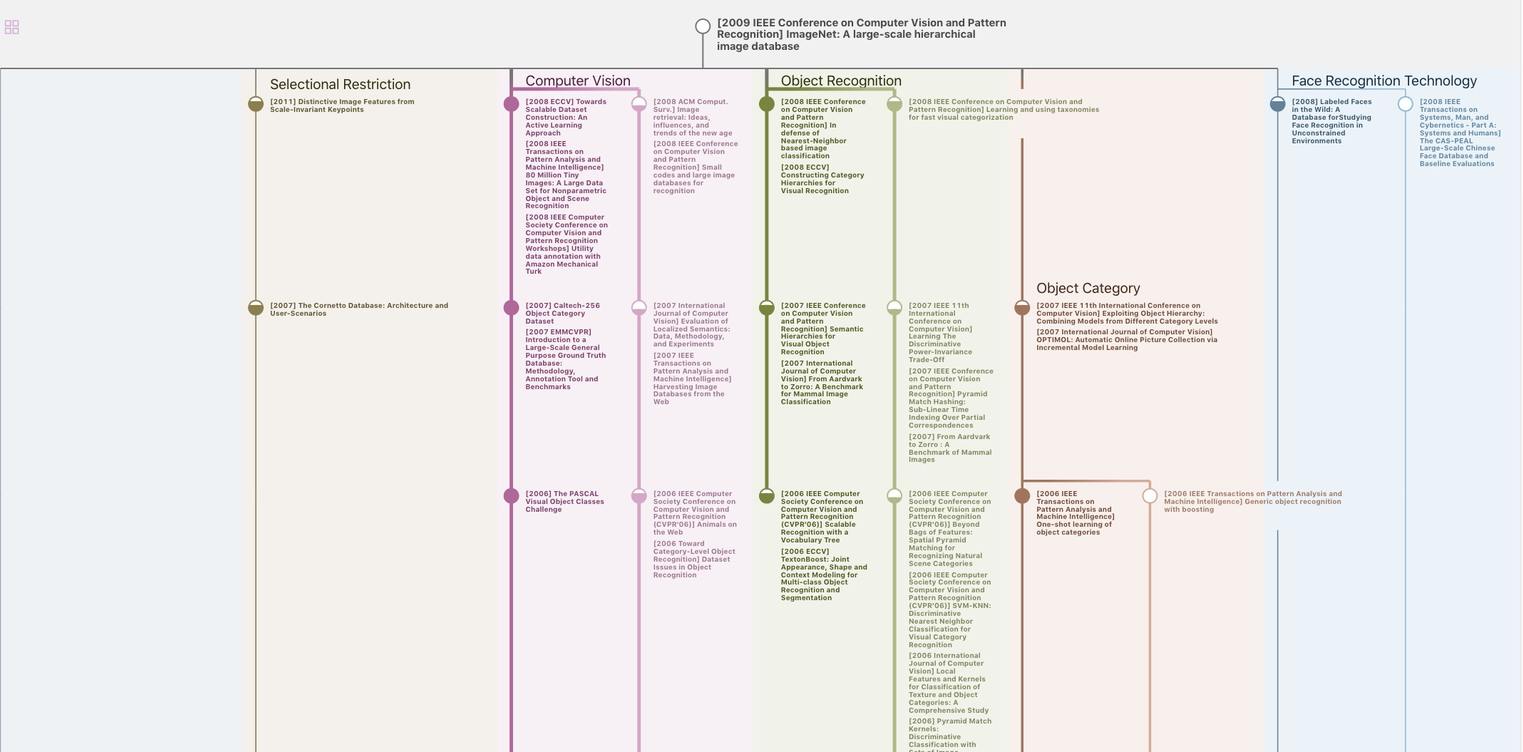
生成溯源树,研究论文发展脉络
Chat Paper
正在生成论文摘要