No-Reference Image Quality Assessment With Deep Convolutional Neural Networks
2016 IEEE INTERNATIONAL CONFERENCE ON DIGITAL SIGNAL PROCESSING (DSP)(2016)
摘要
The state-of-the-art general-purpose no-reference image or video quality assessment (NR-I/VQA) algorithms usually rely on elaborated hand-crafted features which capture the Natural Scene Statistics (NSS) properties. However, designing these features is usually not an easy problem. In this paper, we describe a novel general-purpose NR-IQA framework which is based on deep Convolutional Neural Networks (CNN). Directly taking a raw image as input and outputting the image quality score, this new framework integrates the feature learning and regression into one optimization process, which provides an end-to-end solution to the NR-IQA problem and frees us from designing hand-crafted features. This approach achieves excellent performance on the LIVE dataset and is very competitive with other state-of-the-art NR-IQA algorithms.
更多查看译文
关键词
no-reference image quality assessment,convolutional neural networks,network in network
AI 理解论文
溯源树
样例
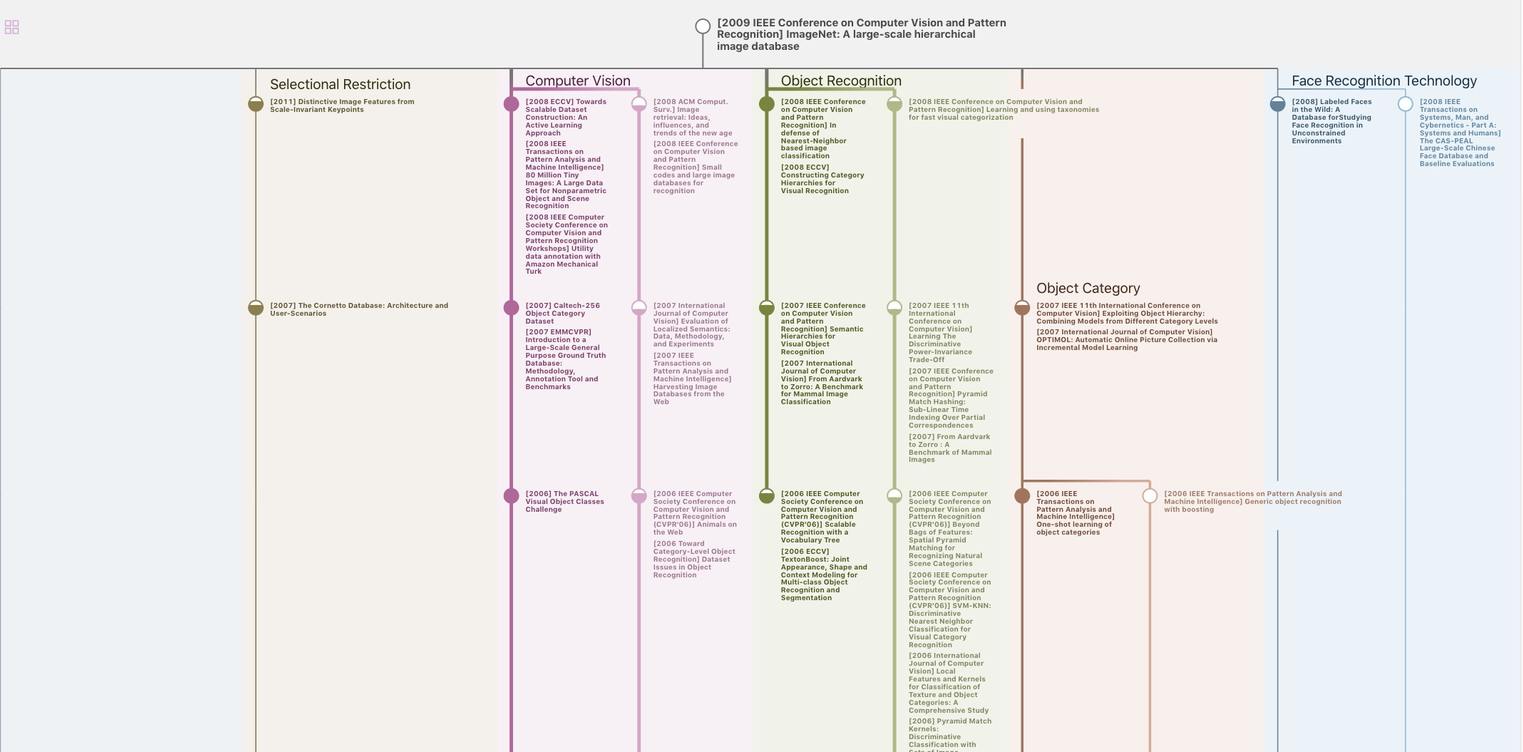
生成溯源树,研究论文发展脉络
Chat Paper
正在生成论文摘要