Integrated Machine Learning Approaches for Predicting Ischemic Stroke and Thromboembolism in Atrial Fibrillation.
PubMed(2016)
摘要
Atrial fibrillation (AF) is a common cardiac rhythm disorder, which increases the risk of ischemic stroke and other thromboembolism (TE). Accurate prediction of TE is highly valuable for early intervention to AF patients. However, the prediction performance of previous TE risk models for AF is not satisfactory. In this study, we used integrated machine learning and data mining approaches to build 2-year TE prediction models for AF from Chinese Atrial Fibrillation Registry data. We first performed data cleansing and imputation on the raw data to generate available dataset. Then a series of feature construction and selection methods were used to identify predictive risk factors, based on which supervised learning methods were applied to build the prediction models. The experimental results show that our approach can achieve higher prediction performance (AUC: 0.71~0.74) than previous TE prediction models for AF (AUC: 0.66~0.69), and identify new potential risk factors as well.
更多查看译文
AI 理解论文
溯源树
样例
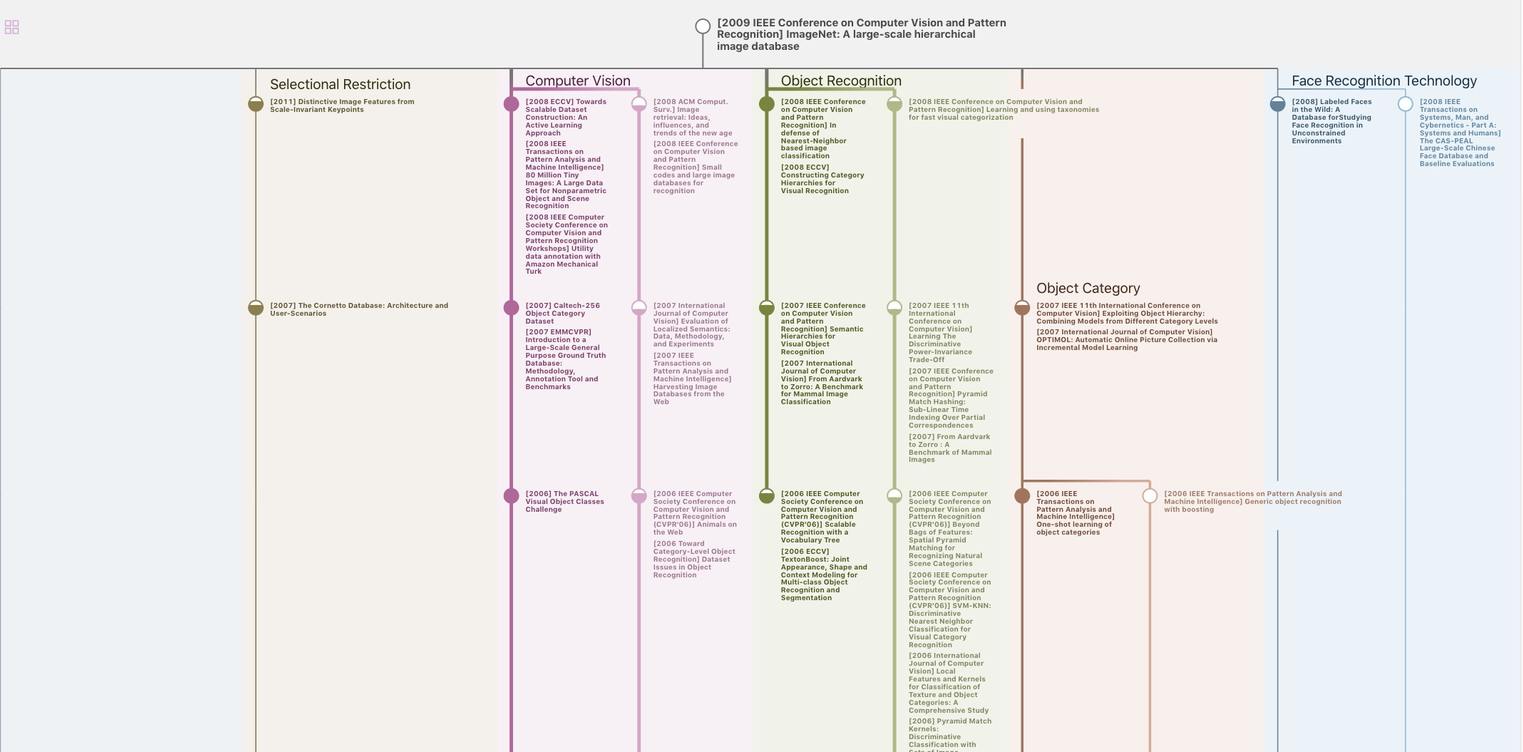
生成溯源树,研究论文发展脉络
Chat Paper
正在生成论文摘要