Dependency-Tree Based Convolutional Neural Networks for Aspect Term Extraction.
PAKDD(2017)
摘要
Aspect term extraction is one of the fundamental subtasks in aspect-based sentiment analysis. Previous work has shown that sentences’ dependency information is critical and has been widely used for opinion mining. With recent success of deep learning in natural language processing (NLP), recurrent neural network (RNN) has been proposed for aspect term extraction and shows the superiority over feature-rich CRFs based models. However, because RNN is a sequential model, it can not effectively capture tree-based dependency information of sentences thus limiting its practicability. In order to effectively exploit sentences’ dependency information and leverage the effectiveness of deep learning, we propose a novel dependency-tree based convolutional stacked neural network (DTBCSNN) for aspect term extraction, in which tree-based convolution is introduced over sentences’ dependency parse trees to capture syntactic features. Our model is an end-to-end deep learning based model and it does not need any human-crafted features. Furthermore, our model is flexible to incorporate extra linguistic features to further boost the model performance. To substantiate, results from experiments on SemEval2014 Task4 datasets (reviews on restaurant and laptop domain) show that our model achieves outstanding performance and outperforms the RNN and CRF baselines.
更多查看译文
关键词
Aspect term extraction, Dependency information, Tree-based convolution, Deep learning
AI 理解论文
溯源树
样例
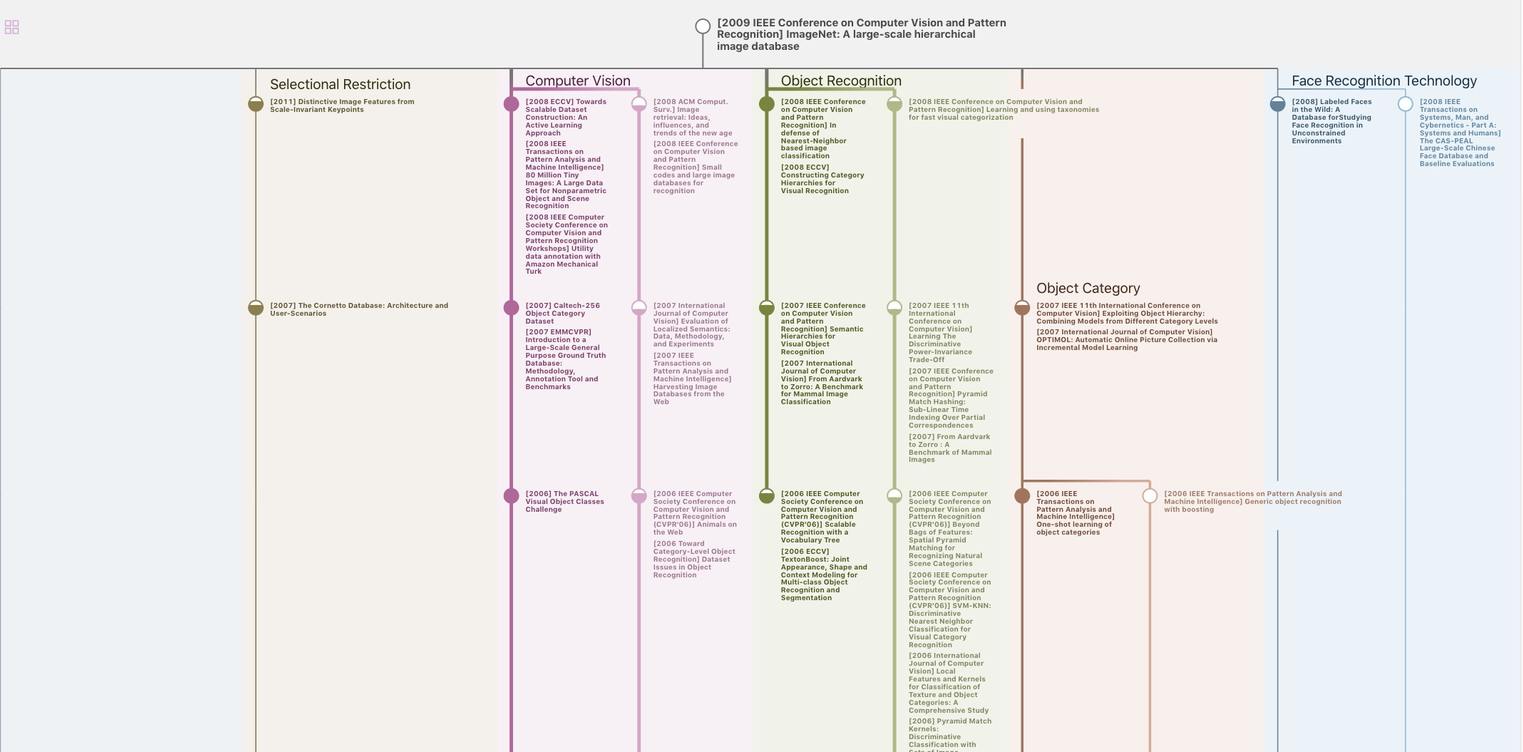
生成溯源树,研究论文发展脉络
Chat Paper
正在生成论文摘要