Scaling Up Ensemble Of Adaptations For Classification By Approximate Nearest Neighbor Retrieval
CASE-BASED REASONING RESEARCH AND DEVELOPMENT, ICCBR 2017(2017)
摘要
Acquisition of case adaptation knowledge is a classic challenge for case-based reasoning. A promising response is learning adaptation rules from cases in the case base, using the case difference heuristic (CDH). In previous research we presented Ensembles of Adaptations for Regression (EAR), an approach that uses a CDH-based method to generate adaptation rules and then exploits the availability of multiple learned rules to apply ensemble-based adaptation. We extended EAR to classification tasks, with Ensembles of Adaptations for Classification (EAC), which showed promising accuracy results. EAR and EAC are practical for standard case bases, but become computationally expensive for large case bases and large ensembles, primarily due to retrieval cost. This paper presents research on scaling up EAC by integrating it with EACH, a new method for efficient approximate retrieval that extends locality-sensitive hashing retrieval to categorical features. Experimental results support the ability of the EAC with EACH (Ensemble of Adaptations for Classifications Hashing) to maintain accuracy while increasing efficiency. In addition, EACH could be applied as a standalone method to provide scalable approximate nearest neighbor retrieval in other CBR retrieval contexts.
更多查看译文
关键词
Case adaptation learning, Case difference heuristic, Ensemble of adaptations for classification, Locality sensitive hashing, Value difference metric
AI 理解论文
溯源树
样例
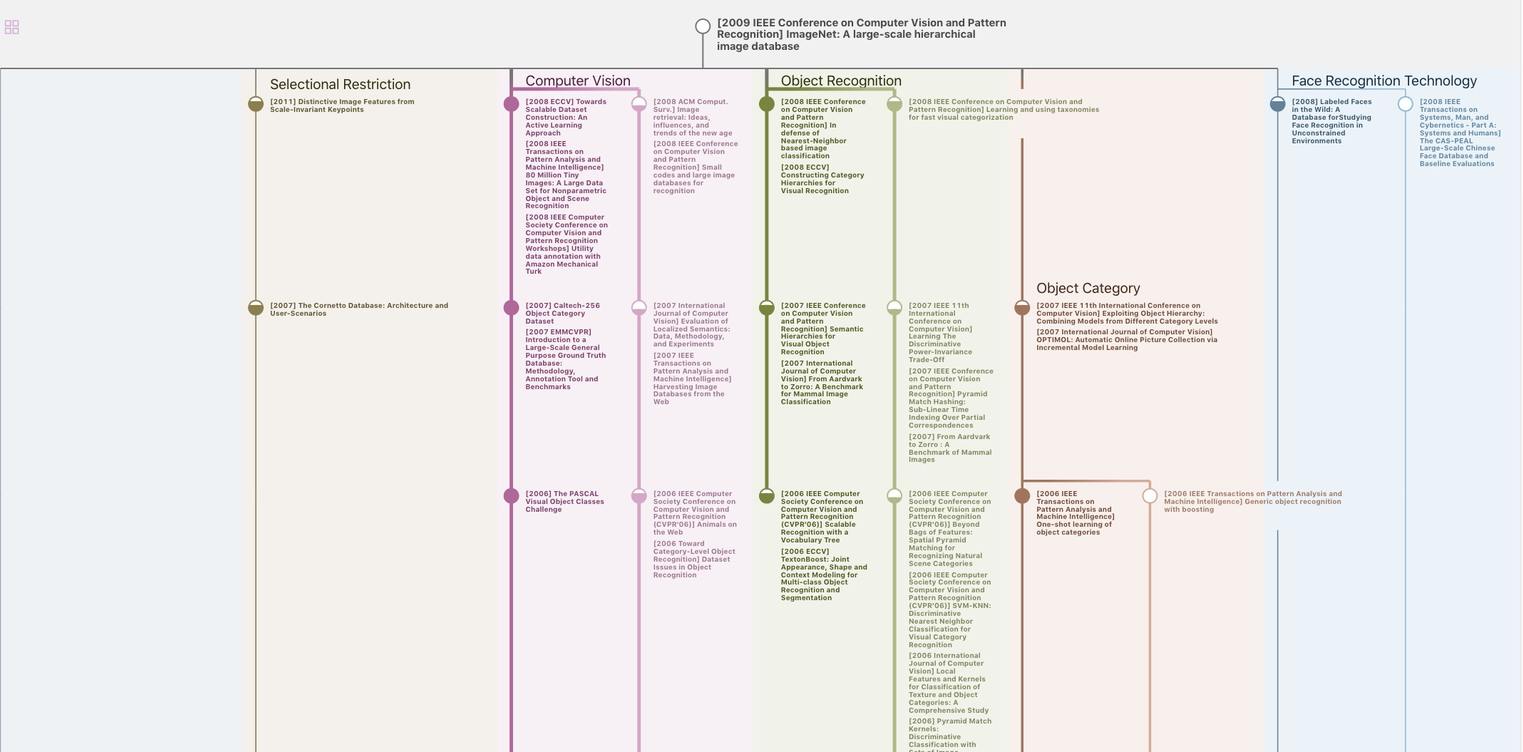
生成溯源树,研究论文发展脉络
Chat Paper
正在生成论文摘要