Identifying Abnormal Network Alterations Common to Traumatic Brain Injury and Alzheimer’s Disease Patients Using Functional Connectome Data
MLMI(2015)
摘要
The objective of this study is to determine if patients with traumatic brain injury (TBI) have similar pathological changes in brain network organization as patients with Alzheimer’s disease (AD) using functional connectome data reconstructed from resting-state fMRI (rsfMRI). To achieve our objective a novel machine learning technique is proposed that uses a top-down reverse engineering approach to identify abnormal network alterations in functional connectome data that are common to patients with AD and TBI. In general, if the proposed machine learning approach classifies a TBI connectome as AD, then this suggests a common network pathology exists in the connectomes of AD and TBI. The advantage of proposed machine learning technique is two-fold: 1) existing longitudinal TBI imaging data is not required, and 2) the potential risk of a TBI patient converting to AD later in life does not require a lengthy and potentially expensive longitudinal imaging study. Experiments are provided that show the AD pathology learned by our connectome-based machine learning technique is able to correctly identify TBI patients with 80% accuracy. In summary, this research may lead to early interventions that can dramatically increase the quality of life for TBI patients who may convert to AD.
更多查看译文
AI 理解论文
溯源树
样例
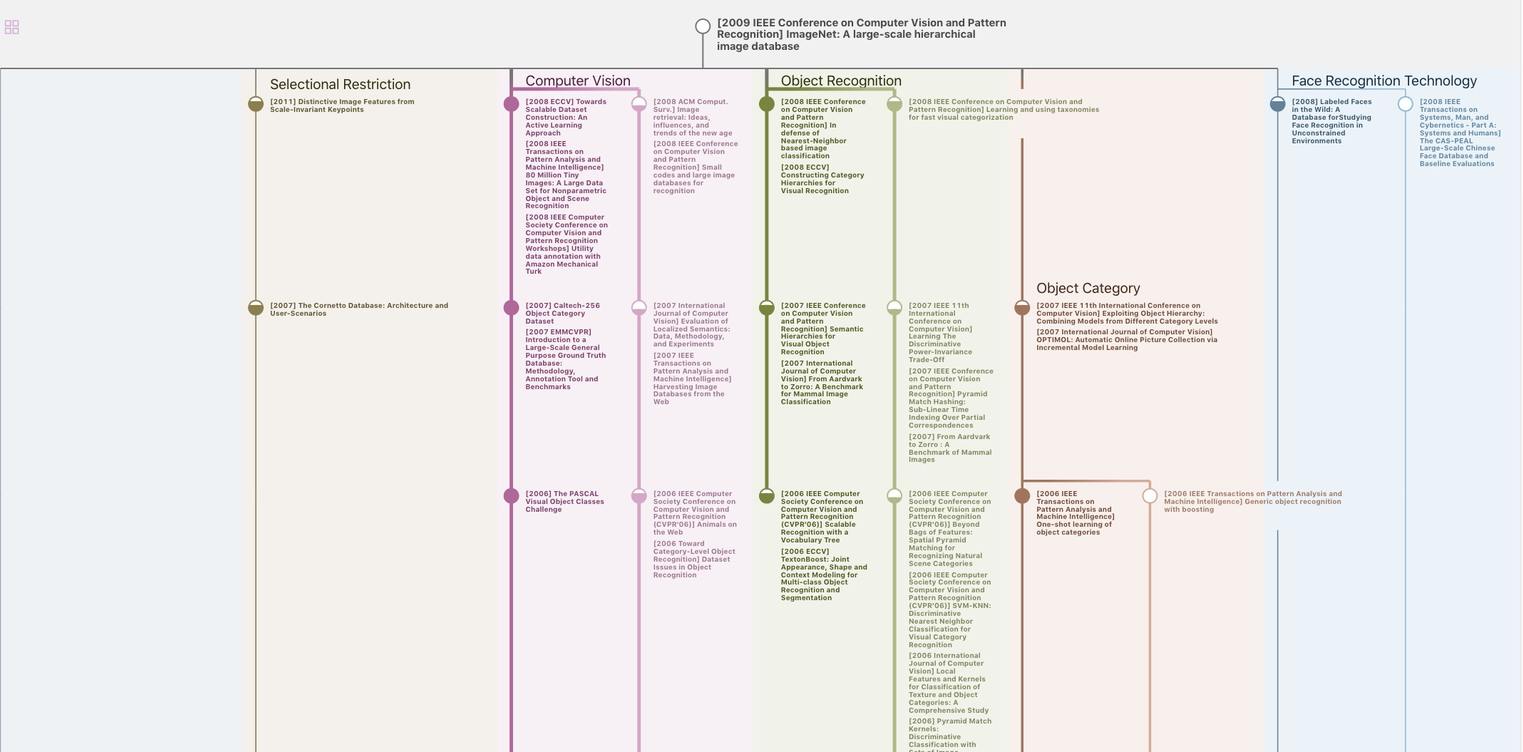
生成溯源树,研究论文发展脉络
Chat Paper
正在生成论文摘要