A Two-Step Cascade Classification Method
2017 INTERNATIONAL JOINT CONFERENCE ON NEURAL NETWORKS (IJCNN)(2017)
摘要
This paper proposes a classification approach in which monolithic and multiple classifier systems are combined in a cascading fashion. The rationale behind that is to deal with the existing trade-off between the need for increasing the accuracy, while reducing the complexity of the classification method. In other words, the idea is to offer an interesting strategy to conciliate the different levels of efforts necessary to deal with easy and hard patterns usually observed in a classification problem. The experimental results have shown that for some problems more than 90% of the instances can be processed in the first step of the cascade, saving efforts by avoiding the use of the second step in which a more complex classification method is used. It means that for some problems the reduction of the classification cost achieved more than 70% when compared to the use of an MCS. In addition to this interesting classification cost reduction, the cascade approach has shown to be able of improving the classification accuracy up to 15.19 percentage points.
更多查看译文
关键词
two-step cascade classification method,monolithic classifier systems,multiple classifier systems,classification method complexity,MCS,classification cost reduction
AI 理解论文
溯源树
样例
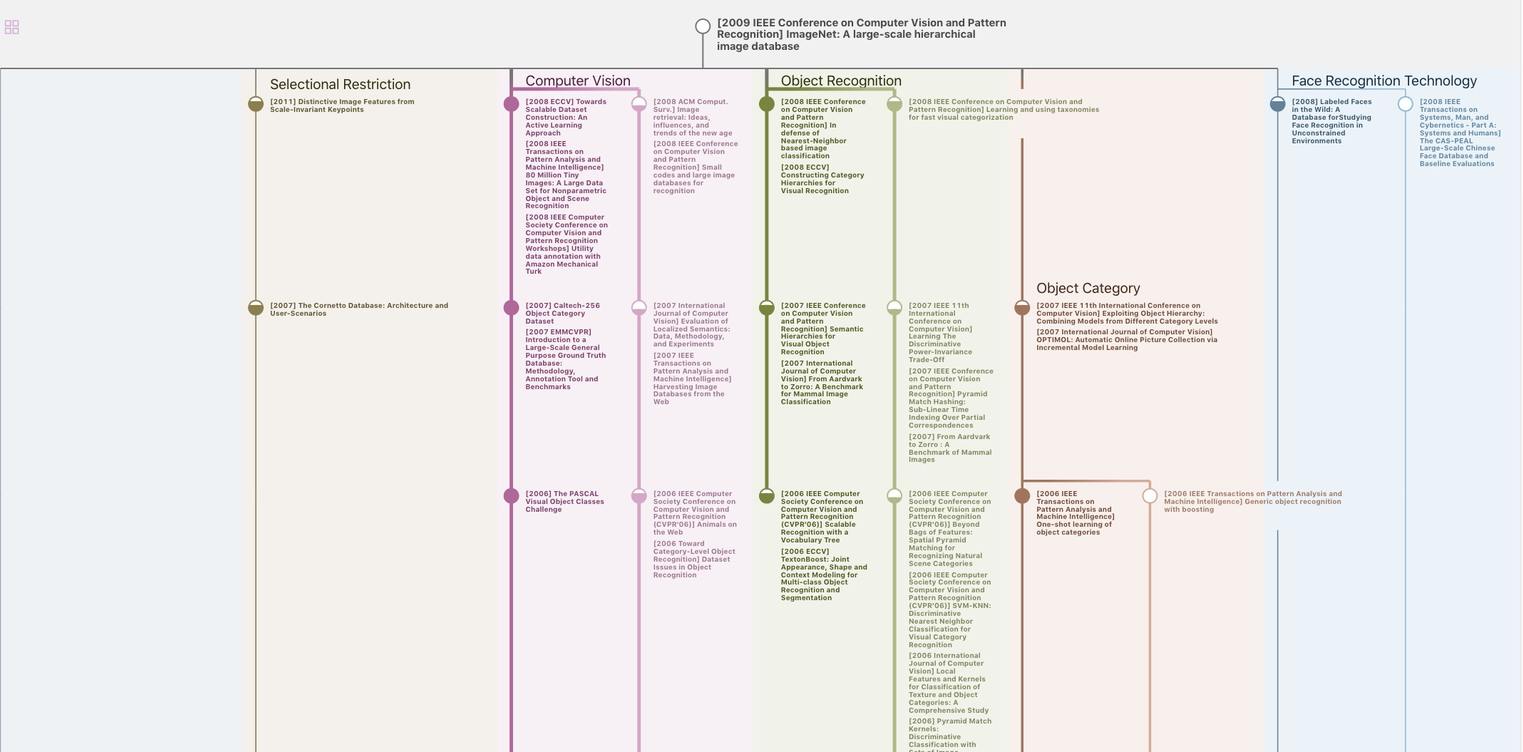
生成溯源树,研究论文发展脉络
Chat Paper
正在生成论文摘要