Concept Drift Learning With Alternating Learners
2017 INTERNATIONAL JOINT CONFERENCE ON NEURAL NETWORKS (IJCNN)(2017)
摘要
Data-driven predictive analytics are in use today across a number of industrial applications, but further integration is hindered by the requirement of similarity among model training and test data distributions. This paper addresses the need of learning from possibly nonstationary data streams, or under concept drift, a commonly seen phenomenon in practical applications. A simple dual-learner ensemble strategy, alternating learners framework, is proposed. A long-memory model learns stable concepts from a long relevant time window, while a short-memory model learns transient concepts from a small recent window. The difference in prediction performance of these two models is monitored and induces an alternating policy to select, update and reset the two models. The method features an online updating mechanism to maintain the ensemble accuracy, and a concept-dependent trigger to focus on relevant data. Through empirical studies the method demonstrates effective tracking and prediction when the steaming data carry abrupt and/or gradual changes.
更多查看译文
关键词
Online learning, streaming data analysis, alternating learners, ensemble learning, model management
AI 理解论文
溯源树
样例
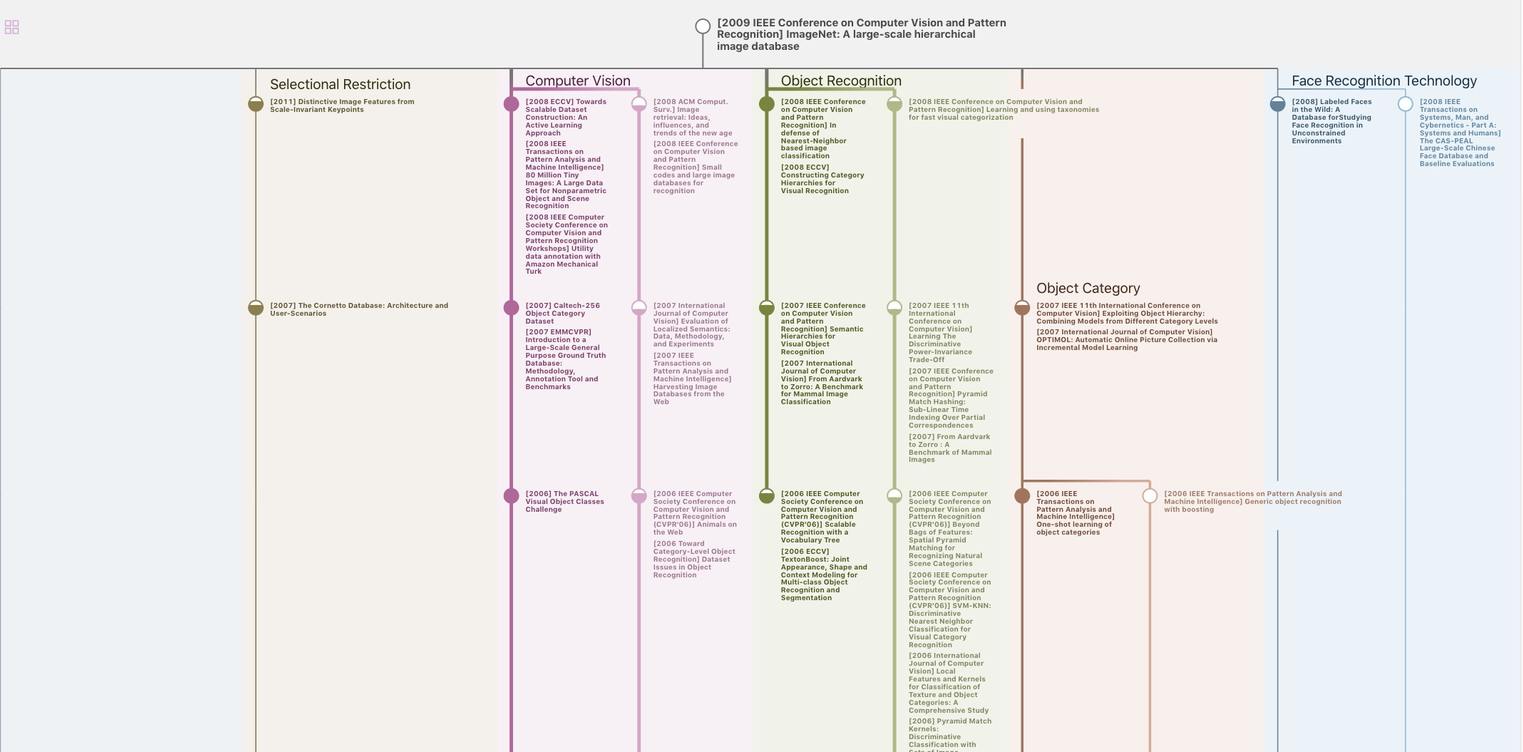
生成溯源树,研究论文发展脉络
Chat Paper
正在生成论文摘要