Improving recommendation accuracy using networks of substitutable and complementary products
2017 INTERNATIONAL JOINT CONFERENCE ON NEURAL NETWORKS (IJCNN)(2017)
摘要
Recommender systems are ubiquitous in applications ranging from e-commerce to social media, helping users to navigate a huge selection of items and to meet a variety of special needs and user tastes. Incorporating contextual knowledge into such systems - such as relational information - has proven to be an effective way to improve recommendation accuracy. A popular line of research aims to model relationships between users, through their connections in a social network. In contrast, we aim to model complex relationships between products, using data based on co-purchase and co-browsing behavior. Modeling such networks presents a variety of challenges, in particular because the features that make two items complementary (or likely to be co-purchased) are far more complex than mere similarity. To model these complex relationships we develop a method based on pairwise ranking and embedding learning to build representations of items based on their co-purchasing and co-browsing statistics. We conduct experiments on Amazon product data to demonstrate that modeling such relationships significantly improves accuracy compared to competitive baselines.
更多查看译文
关键词
recommendation accuracy,substitutable network,complementary products,recommender systems,contextual knowledge,social network,copurchase behavior,cobrowsing behavior,pairwise ranking,embedding learning,item representation,copurchasing statistics,cobrowsing statistics,Amazon product data
AI 理解论文
溯源树
样例
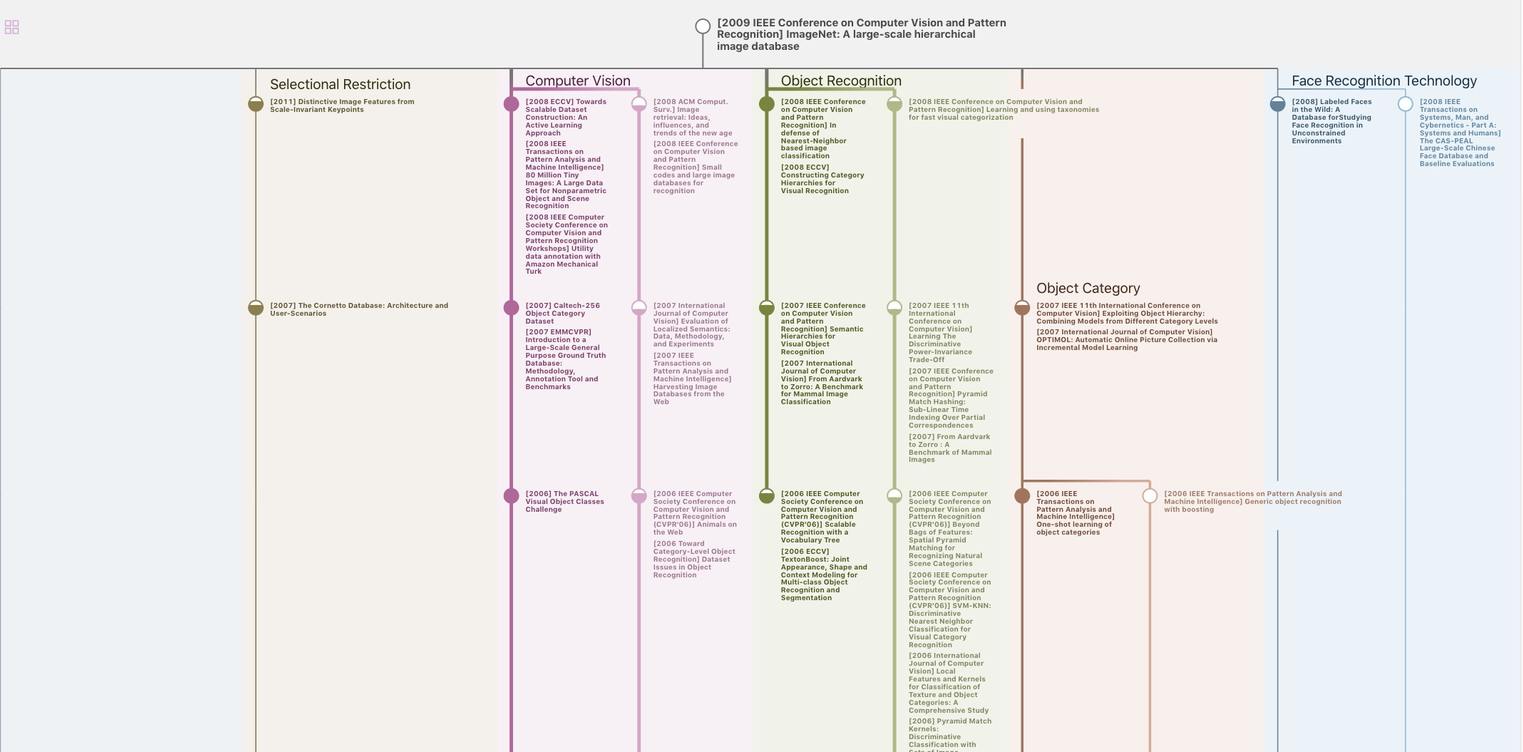
生成溯源树,研究论文发展脉络
Chat Paper
正在生成论文摘要