Pose-Robust Face Verification By Exploiting Competing Tasks
2017 IEEE WINTER CONFERENCE ON APPLICATIONS OF COMPUTER VISION (WACV 2017)(2017)
摘要
In this paper, we propose a pose-robust metric learning framework for unconstrained face verification by jointly optimizing face and pose verification tasks. We learn a joint model for these two tasks and explicitly discourage the information sharing between pose and identity verification metrics so as to mitigate the information contained in the pose verification task leading to making the identity metrics for face verification more pose-robust. Specifically, we use the joint Bayesian metric learning framework to learn the metrics for both tasks and enforce an orthogonal regularization constraint on the learned projection matrices for the two tasks. The pose labels used for training the joint model are automatically estimated and do not require extra annotations. An efficient stochastic gradient descent (SGD) algorithm is used to solve the optimization problem. We conduct extensive experiments on three challenging unconstrained face datasets and show promising results compared to state-of-the-art methods.
更多查看译文
关键词
pose-robust face verification,pose-robust metric learning,unconstrained face verification,joint Bayesian metric learning,orthogonal regularization constraint,projection matrices,stochastic gradient descent algorithm,SGD algorithm,optimization problem
AI 理解论文
溯源树
样例
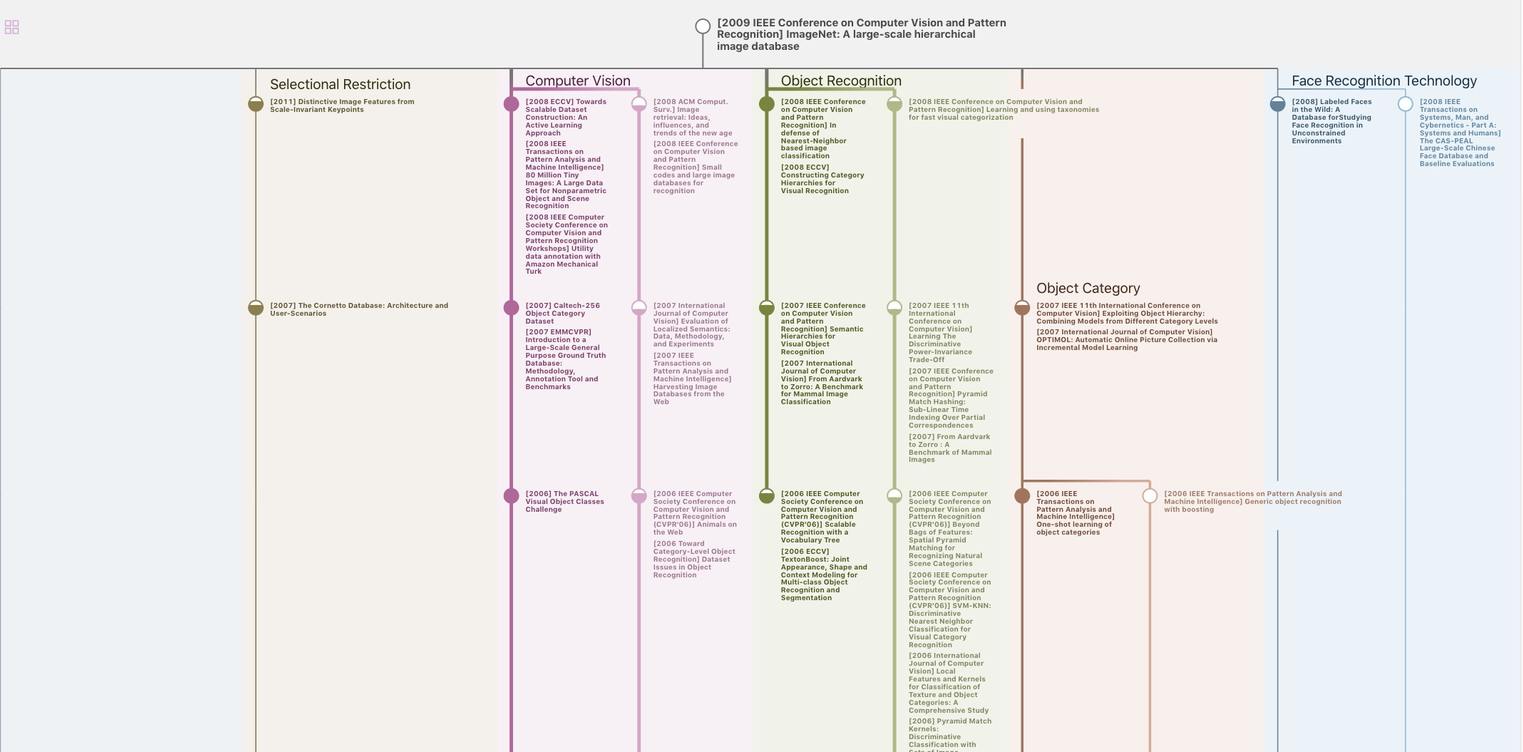
生成溯源树,研究论文发展脉络
Chat Paper
正在生成论文摘要