Combining CMA-ES and MOEA/DD for many-objective optimization.
CEC(2017)
摘要
Multi-objective Estimation of Distribution Algorithms (MOEDAS) have been successfully applied to solve Multi-objective Optimization Problems (MOPs) since they are able to model dependencies between variables of the problem and then sample new solutions to guide the search to promising areas. A state-of-the-art optimizer for single-objective continuous functions that also uses probabilistic modeling is the Covariance Matrix Adaptation Evolution Strategy (CMA-ES). Different variants of CMA-ES have been proposed for MOPs however most of them are based on Pareto dominance as the main selection criterion. Recently, a new multi-objective CMA-ES called MOEA/D-CMA was proposed combining the strengths of CMA-ES with those of the multi-objective evolutionary algorithm based on decomposition (MOEA/D). Nowadays, however, researchers on MOEAs agree that combining Pareto and decomposition can be beneficial for the search on MOPs. As a result, a new MOEA has been proposed, called MOEA/DD. This algorithm modifies the MOEA/D by including a new Pareto dominance update mechanism that brings more diversity into the search. In this study, we extend the MOEA/D-CMA by replacing its update mechanism by the one of MOEA/DD. The hypothesis is that this update mechanism will improve the performance of MOEA/D-CMA as it improved MOEA/D. MOEA/D-CMA and MOEA/DD-CMA are implemented and evaluated through an experimental study. The experimental study involves two well-known families of benchmark problems whose objective numbers scale from two to fifteen. Then, an extensive statistical analysis of the results is made to extract sound, statistically supported conclusions about the performance of the algorithms as the number of objectives scales.
更多查看译文
关键词
CMA-ES, MOEA/D, dominance and decomposition, multi-objective, Gaussian model
AI 理解论文
溯源树
样例
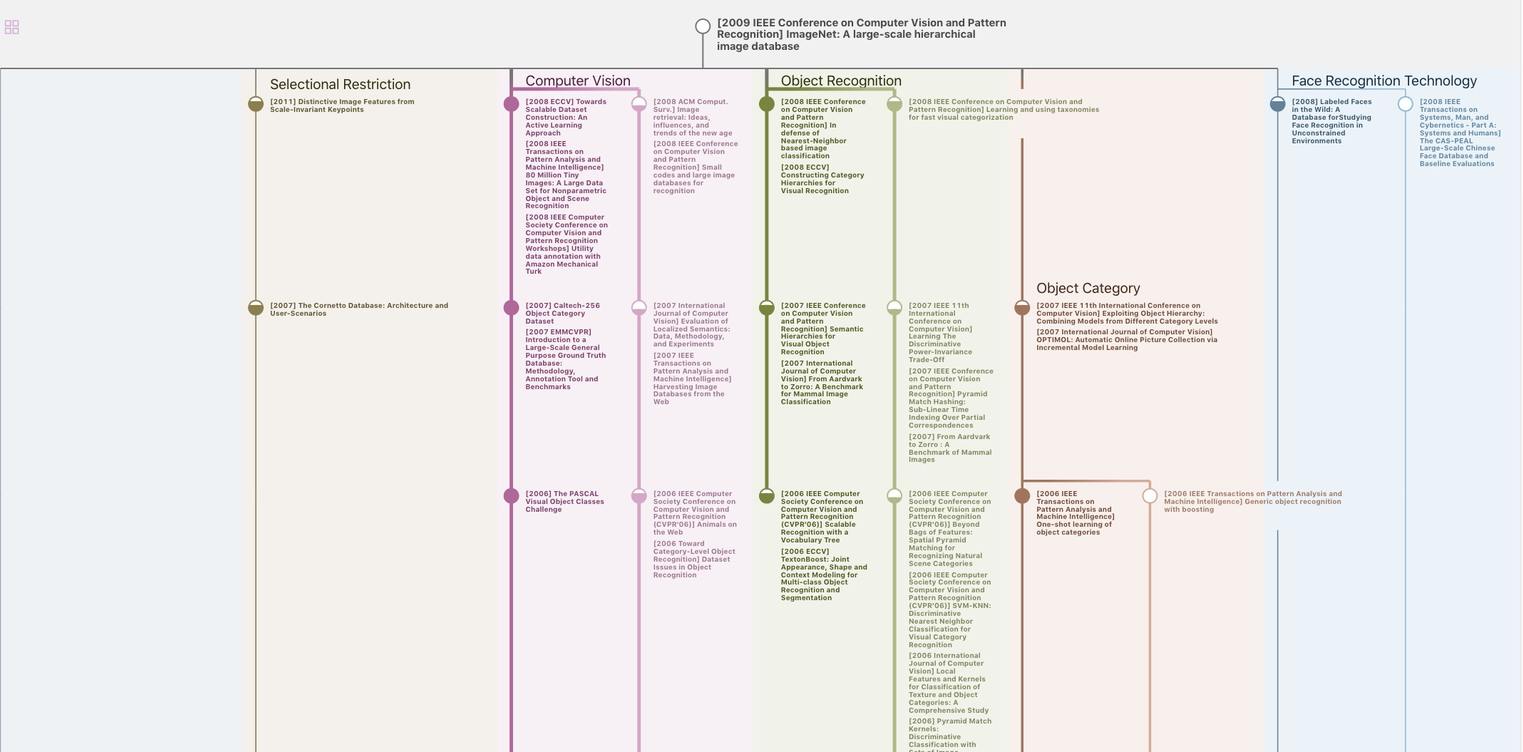
生成溯源树,研究论文发展脉络
Chat Paper
正在生成论文摘要