Candidate Oil Spill Detection In Slar Data A Recurrent Neural Network-Based Approach
ICPRAM: PROCEEDINGS OF THE 6TH INTERNATIONAL CONFERENCE ON PATTERN RECOGNITION APPLICATIONS AND METHODS(2017)
摘要
Intentional oil pollution damages marine ecosystems. Therefore, society and governments require maritime surveillance for early oil spill detection. The fast response in the detection process helps to identify the offenders in the vast majority of cases. Nowadays, it is a human operator whom is trained for carrying out oil spill detection. Operators usually use image processing techniques and data analysis from optical, thermal or radar acquired from aerial vehicles or spatial satellites. The current trend is to automate the oil spill detection process so that this can filter candidate oil spill from an aircraft as a decision support system for human operators. In this work, a robust and automated system for candidate oil spill based on Recurrent Neural Network (RNN) is presented. The aim is to provide a faster identification of candidate oil spills from SLAR scanned sequences. So far, the majority of the research works about oil spill detection are focused on the classification between real oil spills and look-alikes, and they use SAR or optical images but not SLAR data. Moreover, the overall decision is usually taken by an operator, mainly due to the wide variety of types of look-alikes which cause false positives in the detection process using traditional NN. This work provides a RRN-based approach for candidate oil spill detection using SLAR data in contrast with the traditional Multilayer Perceptron Neural Network (MLP). The system is tested with time series data acquired from a SLAR sensor mounted on an aircraft. It achieves a success rate in detecting of 97%.
更多查看译文
关键词
Oil Spill Detection, Maritime Surveillance, SLAR Remote Sensing, RNN, LSTM, Classification
AI 理解论文
溯源树
样例
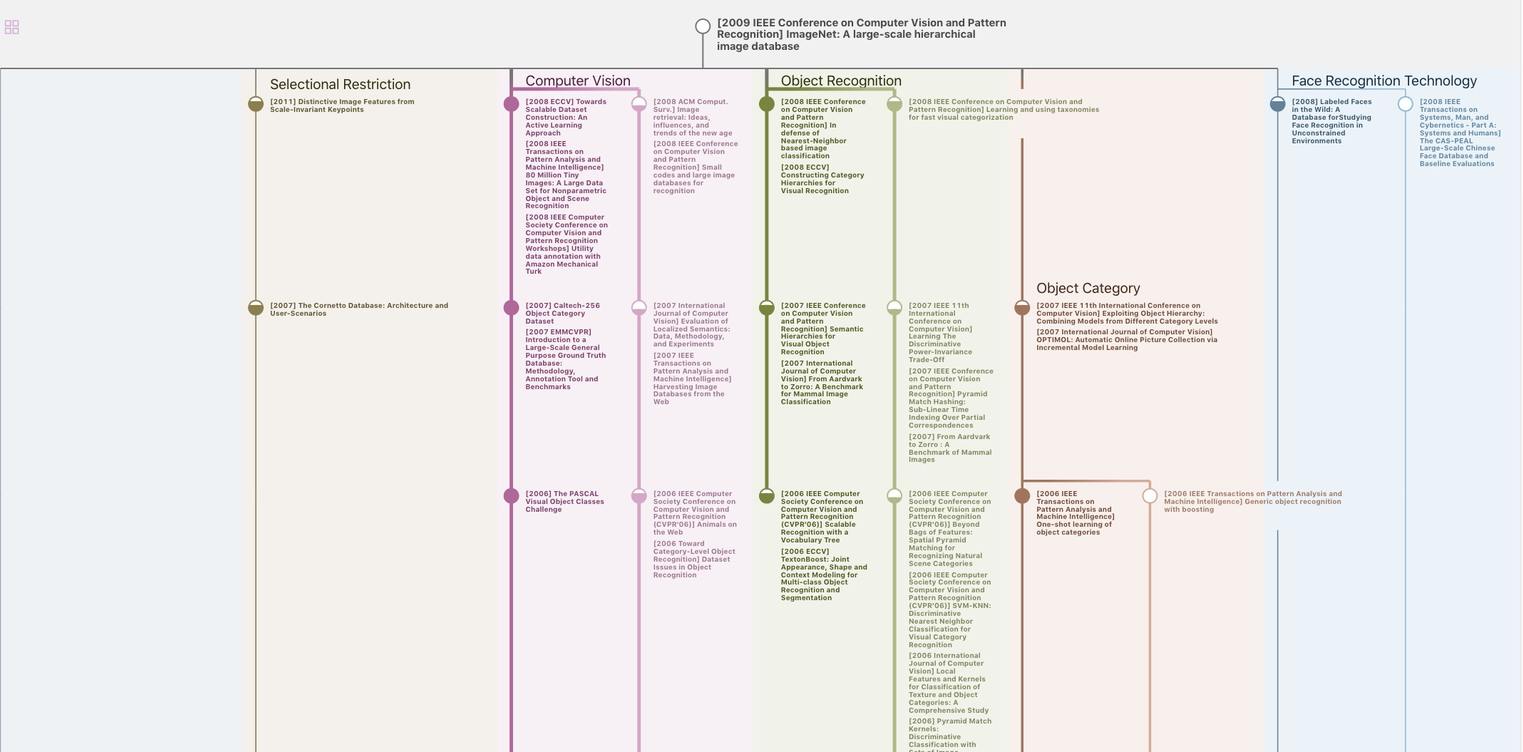
生成溯源树,研究论文发展脉络
Chat Paper
正在生成论文摘要