Image Semantic Distance Metric Learning Approach For Large-Scale Automatic Image Annotation
IOTBD: PROCEEDINGS OF THE INTERNATIONAL CONFERENCE ON INTERNET OF THINGS AND BIG DATA(2016)
摘要
Learning an effective semantic distance measure is very important for the practical application of image analysis and pattern recognition. Automatic image annotation (AIA) is a task of assigning one or more semantic concepts to a given image and a promising way to achieve more effective image retrieval and analysis. Due to the semantic gap between low-level visual features and high-level image semantic, the performances of some image distance metric learning (IDML) algorithms only using low-level visual features is not satisfactory. Since there is the diversity and complexity of large-scale image dataset, only using visual similarity to learn image distance is not enough. To solve this problem, in this paper, the semantic labels of the training image set participate into the image distance measure learning. The experimental results confirm that the proposed image semantic distance metric learning (ISDML) can improve the efficiency of large-scale AIA approach and achieve better annotation performance than the other state-of-the art AIA approaches.
更多查看译文
关键词
Large-scale Automatic Image Annotation,Image Semantic Distance Metric Learning,Improve Performance,Semantic Similarity
AI 理解论文
溯源树
样例
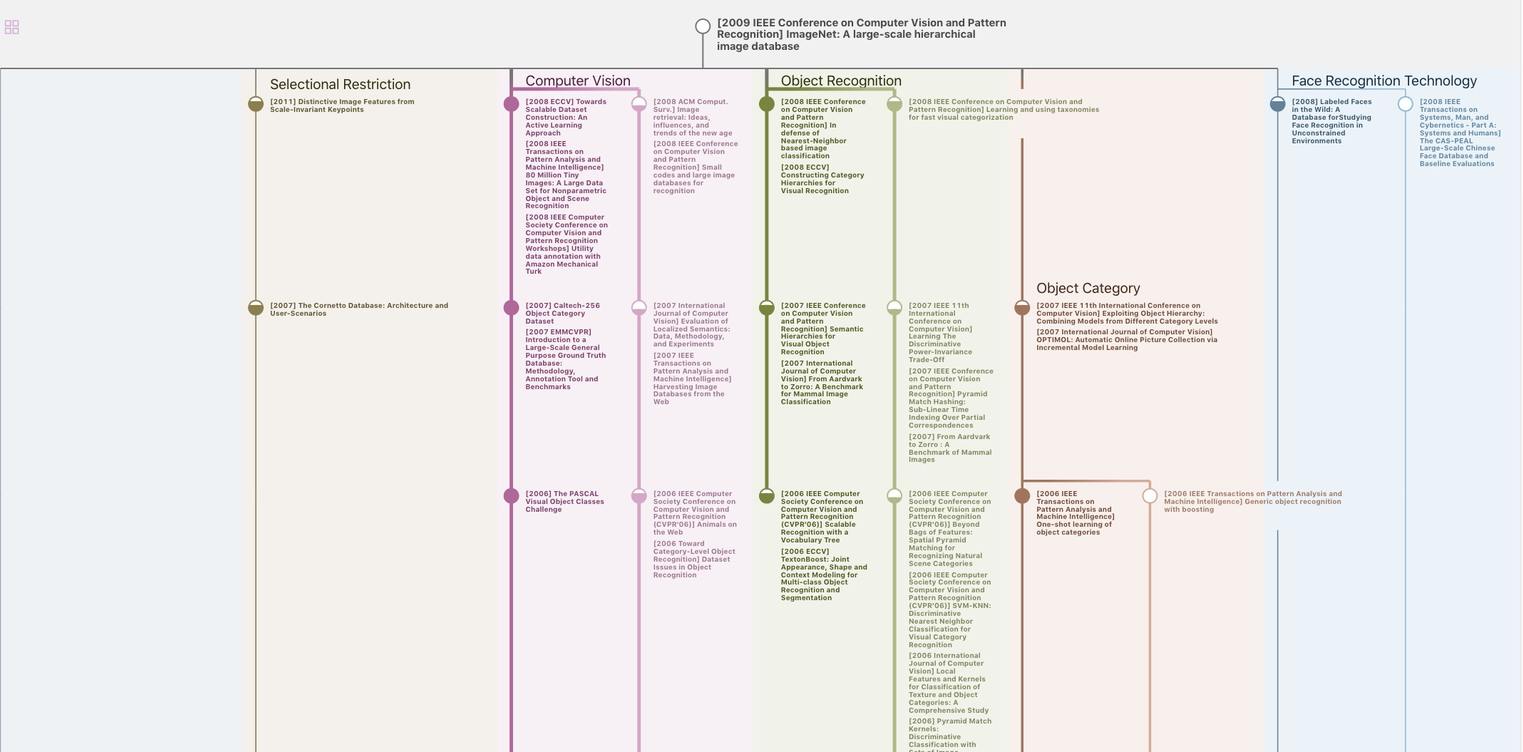
生成溯源树,研究论文发展脉络
Chat Paper
正在生成论文摘要