Crowddqs: Dynamic Question Selection In Crowdsourcing Systems
SIGMOD'17: PROCEEDINGS OF THE 2017 ACM INTERNATIONAL CONFERENCE ON MANAGEMENT OF DATA(2017)
摘要
In this paper, we present CrowdDQS, a system that uses the most recent set of crowdsourced voting evidence to dynamically issue questions to workers on Amazon Mechanical Turk (AMT). CrowdDQS posts all questions to AMT in a single batch, but delays the decision of the exact question to issue a worker until the last moment, concentrating votes on uncertain questions to maximize accuracy. Unlike previous works, CrowdDQS also (1) optionally can decide when it is more beneficial to issue gold standard questions with known answers than to solicit new votes (both can help us estimate worker accuracy, but gold standard questions provide a less noisy estimate of worker accuracy at the expense of not obtaining new votes), (2) estimates worker accuracies in real-time even with limited evidence (with or without gold standard questions), and (3) infers the distribution of worker skill levels to actively block poor workers. We deploy our system live on AMT to over 1000 crowdworkers, and find that CrowdDQS can accurately answer questions using up to 6x fewer votes than standard approaches. We also find there are many non-obvious practical challenges involved in deploying such a system seamlessly to crowdworkers, and discuss techniques to overcome these challenges.
更多查看译文
AI 理解论文
溯源树
样例
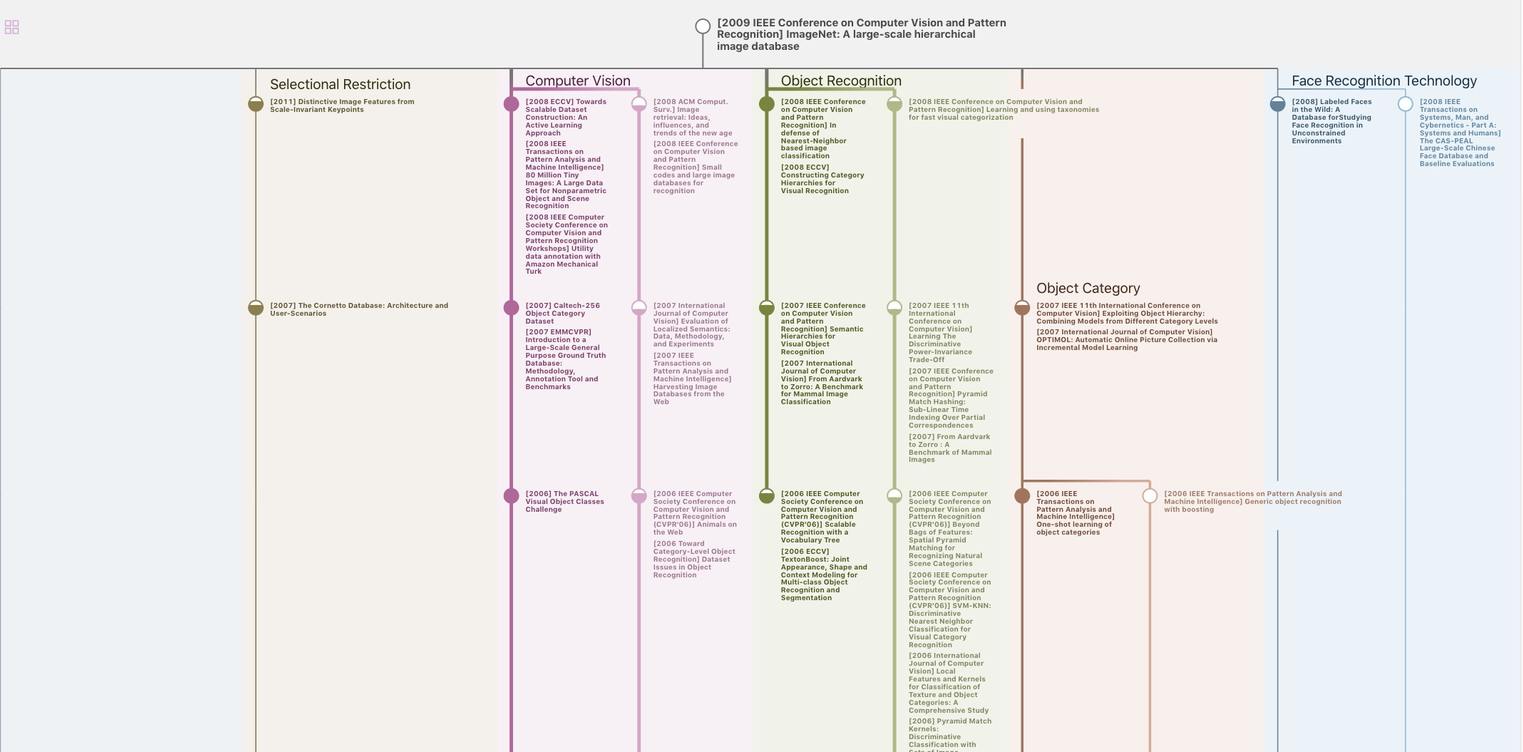
生成溯源树,研究论文发展脉络
Chat Paper
正在生成论文摘要