Detection Of Lumen And Media-Adventitia Borders In Ivus Images Using Sparse Auto-Encoder Neural Network
2017 IEEE 14TH INTERNATIONAL SYMPOSIUM ON BIOMEDICAL IMAGING (ISBI 2017)(2017)
摘要
This paper describes an artificial neural network (ANN) method that employs a feature-learning algorithm to detect the lumen and MA borders in intravascular ultrasound (IVUS) images. Three types of imaging features including spatial, neighboring, and gradient features were used as the input features to the neural network, and then the different vascular layers were distinguished using two sparse autoencoders and one softmax classifier. To smooth the lumen and MA borders detected by the ANN method, we used the active contour model. The performance of our approach was compared with the manual drawing method and another existing method on 538 IVUS images from six subjects. Results showed that our approach had a high correlation (r = 0.9284 +/- 0.9875 for all measurements) and good agreement (bias = 0.0148 +/- 0.4209 mm) with the manual drawing method, and small detection error (lumen border: 0.0928 +/- 0.0935 mm, MA border: 0.1056 +/- 0.1088 mm). The average time to process each image was 14 +/- 4.6 seconds. The obtained results indicate that our proposed approach can be used to efficiently and accurately detect the lumen and MA borders in IVUS images.
更多查看译文
关键词
Intravascular image,Deep neural network,Image segmentation,Sparse autoencoder
AI 理解论文
溯源树
样例
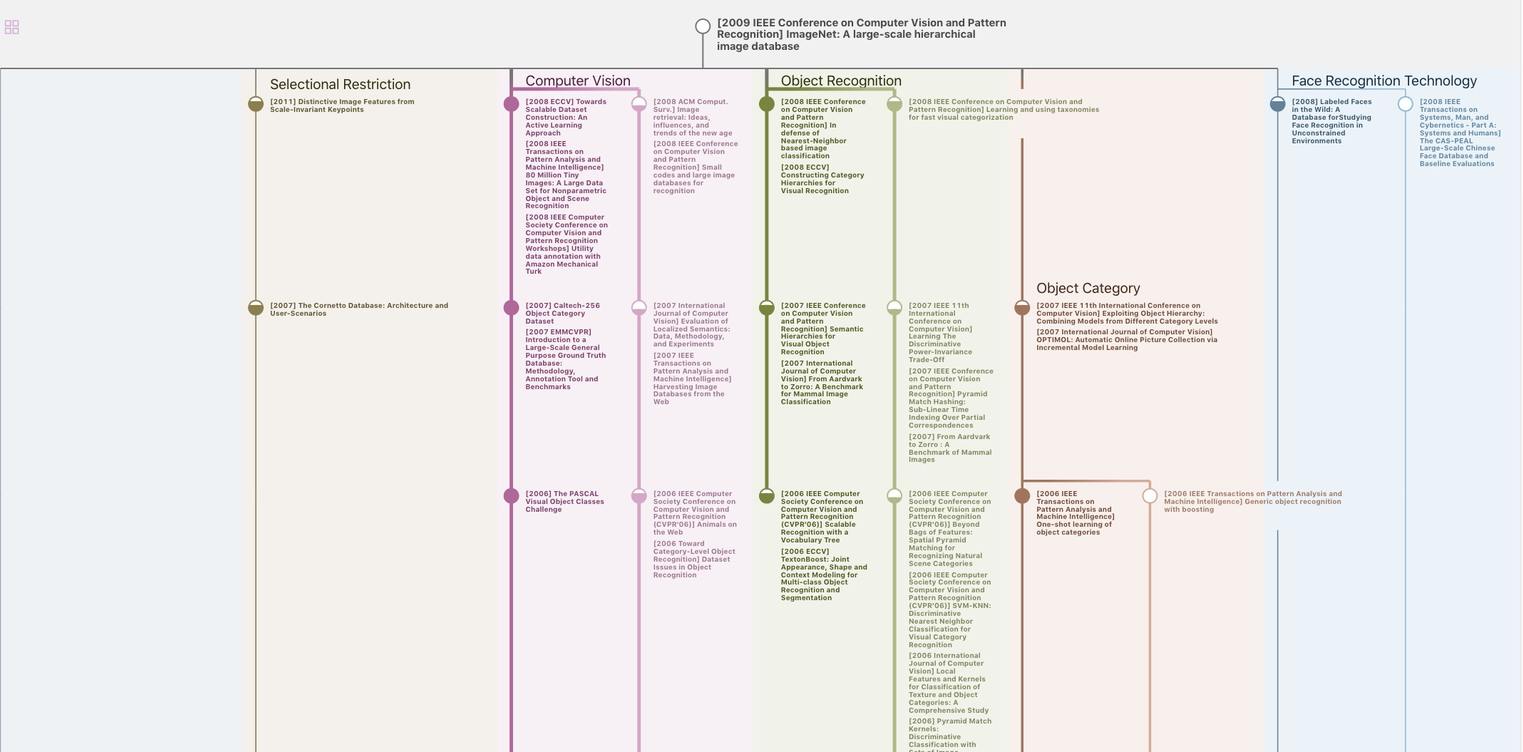
生成溯源树,研究论文发展脉络
Chat Paper
正在生成论文摘要