Making Machine-Learning Applications for Time-Series Sensor Data Graphical and Interactive
TiiS(2017)
摘要
The recent profusion of sensors has given consumers and researchers the ability to collect significant amounts of data. However, understanding sensor data can be a challenge, because it is voluminous, multi-sourced, and unintelligible. Nonetheless, intelligent systems, such as activity recognition, require pattern analysis of sensor data streams to produce compelling results; machine learning (ML) applications enable this type of analysis. However, the number of ML experts able to proficiently classify sensor data is limited, and there remains a lack of interactive, usable tools to help intermediate users perform this type of analysis. To learn which features these tools must support, we conducted interviews with intermediate users of ML and conducted two probe-based studies with a prototype ML and visual analytics system, Gimlets. Our system implements ML applications for sensor-based time-series data as a novel domain-specific prototype that integrates interactive visual analytic features into the ML pipeline. We identify future directions for usable ML systems based on sensor data that will enable intermediate users to build systems that have been prohibitively difficult.
更多查看译文
关键词
Machine learning tools,visual analytic tools,big sensor data,wearable devices
AI 理解论文
溯源树
样例
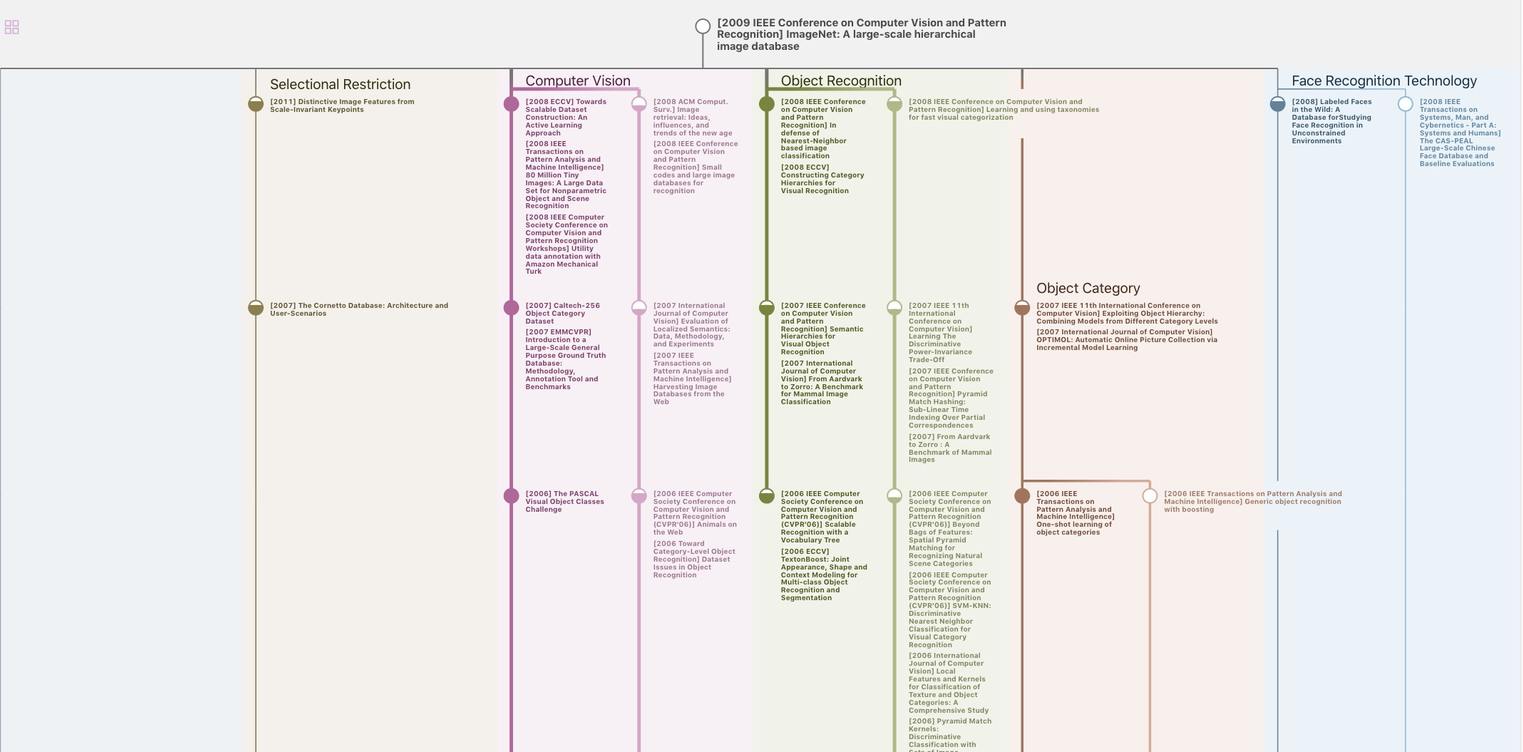
生成溯源树,研究论文发展脉络
Chat Paper
正在生成论文摘要