DOCS: a domain-aware crowdsourcing system using knowledge bases
Proceedings of the VLDB Endowment(2016)
摘要
AbstractCrowdsourcing is a new computing paradigm that harnesses human effort to solve computer-hard problems, such as entity resolution and photo tagging. The crowd (or workers) have diverse qualities and it is important to effectively model a worker's quality. Most of existing worker models assume that workers have the same quality on different tasks. In practice, however, tasks belong to a variety of diverse domains, and workers have different qualities on different domains. For example, a worker who is a basketball fan should have better quality for the task of labeling a photo related to 'Stephen Curry' than the one related to 'Leonardo DiCaprio'. In this paper, we study how to leverage domain knowledge to accurately model a worker's quality. We examine using knowledge base (KB), e.g., Wikipedia and Freebase, to detect the domains of tasks and workers. We develop Domain Vector Estimation, which analyzes the domains of a task with respect to the KB. We also study Truth Inference, which utilizes the domain-sensitive worker model to accurately infer the true answer of a task. We design an Online Task Assignment algorithm, which judiciously and efficiently assigns tasks to appropriate workers. To implement these solutions, we have built DOCS, a system deployed on the Amazon Mechanical Turk. Experiments show that DOCS performs much better than the state-of-the-art approaches.
更多查看译文
AI 理解论文
溯源树
样例
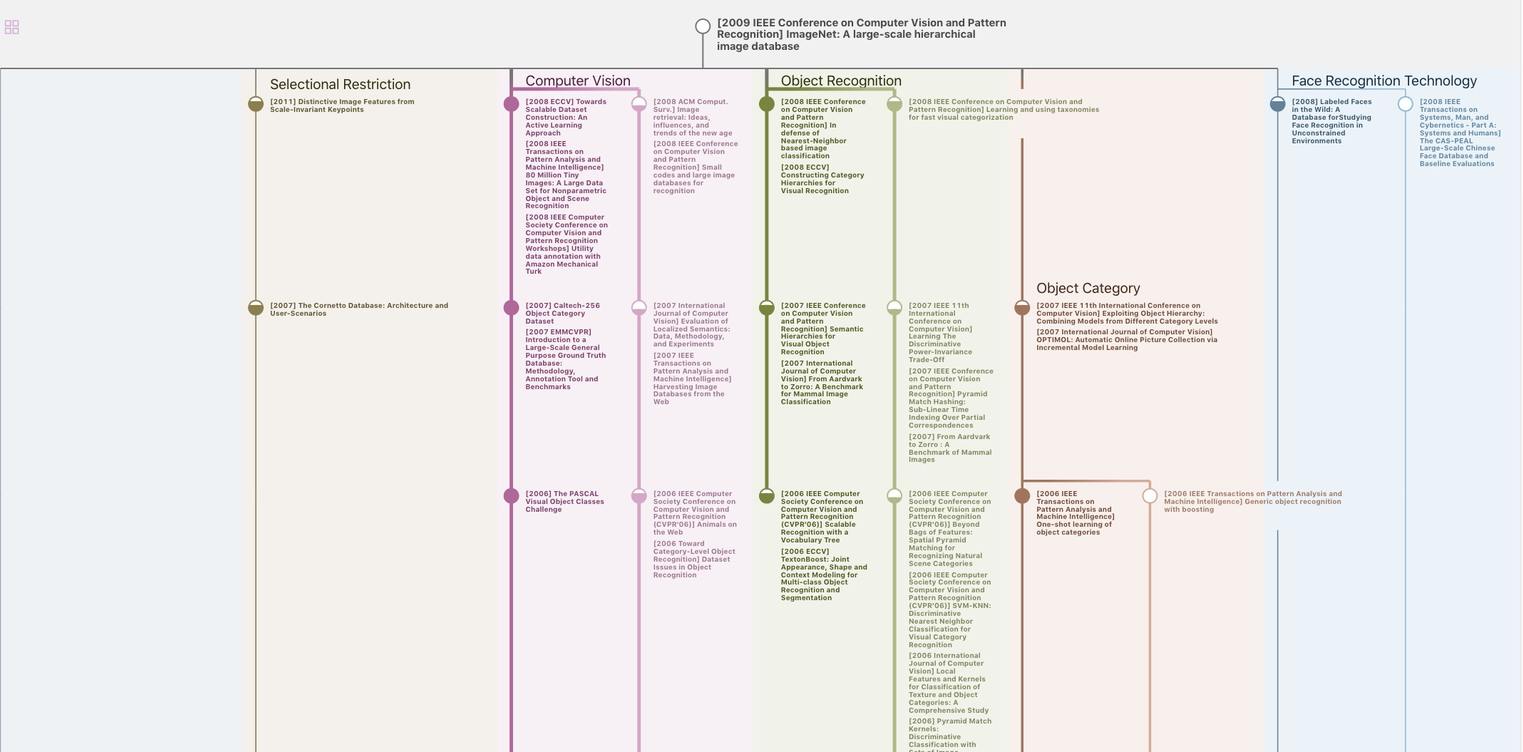
生成溯源树,研究论文发展脉络
Chat Paper
正在生成论文摘要